Shallow learning drone technology makes small robots smart
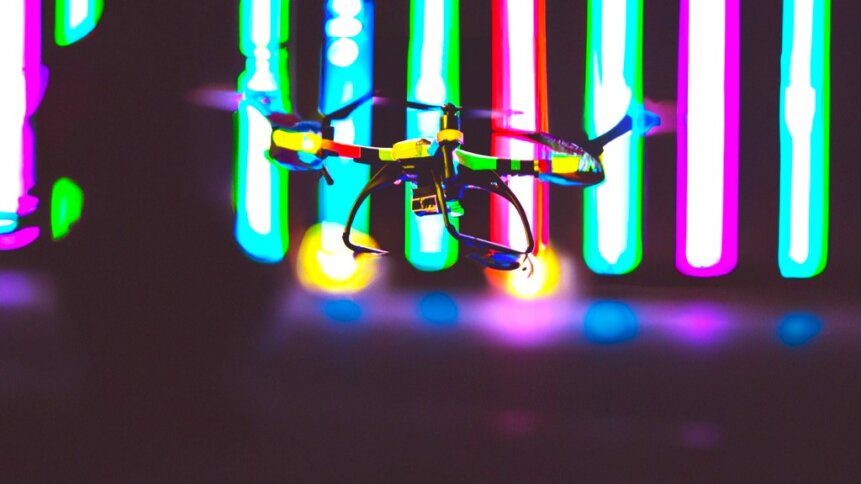
Getting your Trinity Audio player ready...
|
Business applications for drone technology include airborne delivery along routes such as the UK’s proposed Skyway network of autonomous flight corridors. But while larger drones can carry heavier payloads and support multi-sensor data fusion, they are more expensive and less agile in the air.
More compact examples of drone technology, on the other hand, are better suited to navigating tight spaces such as indoor environments. Autonomous flying robots can perform warehouse stock checks in hours, rather than days when the task is carried out manually. And they avoid staff having to climb up ladders.
Search and rescue missions are another use case where drone technology has the potential to outperform humans. However, to excel at the task, flying robots need to be able to navigate surroundings they may have never seen before. Also, given that locations could be remote or even underground, it’s beneficial for search and rescue drones to have the capacity to operate even when connectivity is poor.
There are other situations too, where having compact, lightweight drone technology that can easily dodge obstacles is helpful, such as using small flying robots as artificial pollinators. But less is rarely more when it comes to the hardware required to stop drones from bumping into things – until now.
Drone technology using a single monocular camera
In a study published in the journal Science Robotics, researchers from the Perception and Robotics Group based at the University of Maryland, in the US, have shown how drone technology can successfully navigate unfamiliar situations fed by data from as little as one camera.
Their approach involves determining the uncertainty in neural network predictions and using that information to keep small, palm-sized, flying robots out of trouble. Test settings included having drones fly quickly through an artificial forest, navigate differently shaped holes with just a few centimeters of clearance, and avoid thrown objects.
Dubbed Anja, the framework gives drone technology developers a frugal path to building compact designs with intuition generally associated with more complex, bulky, and expensive hardware.
You can find out more about how drones are the future of warehouse security via the resources section of our website.https://t.co/hVThxkhaLJ #Innovation #Logistics #Automation #Technology #Drone #Security #SecurityProfessionals #AI #Sorair #TechStar…https://t.co/0C7Jx1NwRS
— Sorair Technologies (@SorairTech) September 22, 2023
To achieve its breakthrough, the team gathers information cues from uncertainty in a property known as ‘optical flow’, which refers to the motion of brightness patterns in a scene. Computer vision has long used optical flow as a proxy to determine the magnitude and direction of movement between two images.
It’s not a perfect solution under all circumstances – for example, the motion of a barber’s pole is rotational, but it appears as being downward in direction, according to the optical flow. However, aside from a handful of optical illusions, the property remains useful.
A great example of optical flow in action is the optical computer mouse, which uses the feature to keep track of where the user is pointing the cursor. Optical flow can also help to identify the dominant direction of motion in video footage to compensate for camera shake and provide image stabilization.
Face tracking is another use case – for example, optical flow properties can help determine whether someone is smiling or frowning. However, returning to the theme of drone technology and how to stop flying robots from colliding with unknown objects – the Anja framework capitalizes on effects such as motion blur to aid navigation.
Objects close to the camera will traverse more pixels than those further away. However, the group’s method goes further and leans on the notion that there’s value in knowing what you don’t know. If something appears unexpected or uncertain, then it might be worth moving to a location where confidence in the data is stronger.
Keeping this idea in mind, you can begin to see how drone technology has the potential to find its way around even in the absence of having a large deep-learning neural network fed with a large amount of labeled data.
Ideal for resource-constrained robots
The group’s perception algorithm has just 2.7 million parameters, which may sound like a lot, but is several orders of magnitude less than other methods commonly used. “We hope that this can open new doors for robot autonomy at sizes that were not thought possible before,” comments the team, highlighting how a relatively frugal sensing stack can be competitive with larger, more complex systems.
Model inference – in other words running the shallow neural network to identify the uncertainty in dense optical flow – takes around 49 ms, which leaves plenty of time for the drone technology to react to its surroundings.
“We tracked the obstacle over three frames by detection (segmentation) to compute the direction the obstacle would hit on the image plane,” write the researchers in their paper, discussing how collision avoidance is enabled. “Then, we computed a safe direction and executed a control command to move in that direction for ‘best-effort’ dodging.”
Not only is the approach lightweight in terms of computing resources, it’s fast too – for example, compared with using depth sensing methods – which, in principle, could allow flying robots to travel more rapidly. And the team’s framework requires neither GPS, cloud compute, nor prior map knowledge.