Silicon personas, ChatGPT & the next big thing in digital marketing
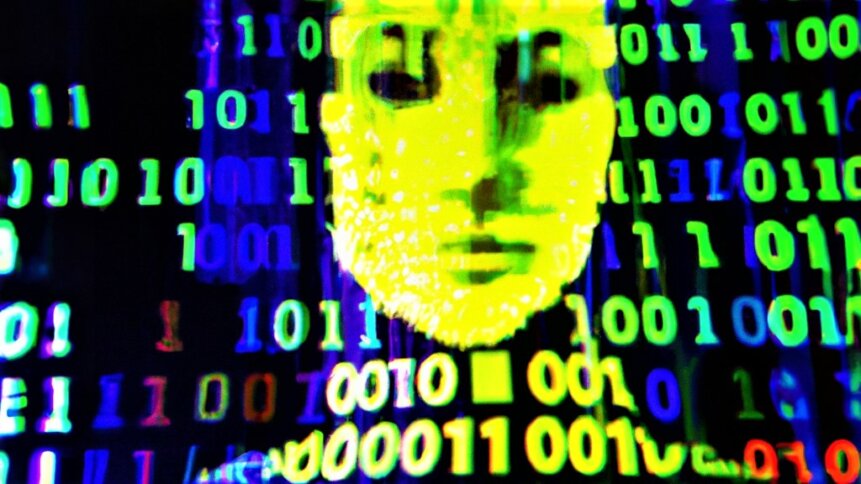
Getting your Trinity Audio player ready...
|
In 2023, it’s crystal clear that large language models (LLMs) are on a trajectory to becoming indispensable enterprise tools. Microsoft is investing billions in its partnership with OpenAI to capitalize on the potential of flagship LMMs such as GPT-4. And the fact that some industry executives are urging for an ‘AI pause’ for six months shines a bright light on the pace of disruption to a huge range of jobs, from managers to models. Adding to that shakeup is an intriguing use of LLMs as silicon personas, which was signposted in a research paper that quietly appeared on the arXiv preprint server in September 2022.
The study, dubbed ‘Out of One, Many: Using Language Models to Simulate Human Samples’, was formally published last month in the journal Political Analysis. But don’t be fooled by the journal’s title; the group’s work could radically change the way that product designers and digital marketers test their ideas and gather insight from potential customers, as we’ll get to.
The research team, which includes corresponding author Lisa P. Argyle, is based in the departments of computer and political science at Brigham Young University in the US. And, realizing that little attention had been paid to the possible applications of LLMs to advance the understanding of human social and political behavior, the group came up with a way of using models – in this case, GPT-3 – as surrogates for human respondents in a variety of social science tasks.
What are silicon personas?
Argyle and her colleagues termed these surrogates, silicon subjects, and these silicon personas – which could equally be created to represent user types – turn out to be incredibly lifelike. In the study, the researchers conditioned GPT-3 with the socio-demographic backstories gathered from 2012, 2016, and 2020 US election survey data, and examined whether they could use their silicon subjects to determine the outcome of the election.
The correlation between the silicon subjects and the actual voter data was incredibly strong, with humans and personas remarkably aligned. And the outcome shows that GPT-3 exhibits sufficiently fine-grained biases and preferences to – in this case – determine which party it would vote for based on the backstory of each of the silicon personas.
What’s most remarkable about the findings is how capable LLMs are outside of their temporal limits. “The training corpus for GPT-3 ended in 2019, so data from 2020 allows us to explore how the algorithmic fidelity of the language model changes when probed outside the time of the original training corpus,” explained the researchers in their paper.
The persistence of the biases in the model meant that GPT-3 could be used to call the result of the US election in 2020, when presented with silicon subjects conditioned with human-like backstories, even though it lacked training data from that period of time. And if you’re thinking that making a jump from silicon subjects to silicon personas – representing not voters, but customers – could be product testing and digital marketing gold, you could be right.
From digital synapses to digital marketing
But before we start to put flesh on the bones of the next big thing in digital marketing and product testing, let’s take a quick look under the hood of GPT-3. OpenAI’s breakthrough LLM (which was extended and finessed into the basis for the wildly popular ChatGPT) has 175 billion parameters, which was 10 times more than any LLM that had gone before it. The parameters represent the weights at each node in the multi-layered neural network, and determine which of these digital synapses ‘fire’ when GPT-3 is presented with a text prompt and attempts to guess the next word in the sequence.
In principle, the more parameters, the more capable the AI model. But all of those billions of parameters need to be trained, and that commits developers to using vast amounts of data. Initially, parameters carry random values, but over time, during training (which took months, using a custom-designed supercomputer hosted on Microsoft Azure) they converge to their optimum values.
READ NEXT
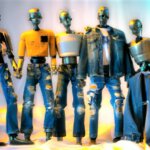
Will AI take our jobs? Yes and…
Many news articles will claim that GPT-3 is a black box, but you can start to see how LLMs make sense of the world when you consider the sources of the training data. And, contrary to some reports, OpenAI has shared some details on the training dataset that it uses. Training sources include Wikipedia, two internet-based books corpora (equivalent to more than 13 billion words), webpages recommended by upvoted Reddit pages, and a filtered and deduplicated version of Common Crawl – a huge resource containing petabytes of data collected from years of web crawling.
So what does this mean for product testing and digital marketing? Digital marketers have been quick to realize that ChatGPT is useful for rapidly generating copy and applying different writing styles – for example, to create social media posts or other marketing assets. But, as mentioned, silicon personas open the door to much more. And their synthetic data could be used in a variety of user testing scenarios.
Customer insight and product testing smarts
To give you an idea, we ran a super quick experiment using OpenAI’s ChatGPT web UI, feeding the advanced chatbot with the following –
Persona 1 = a middle-aged man who likes sports and has a gym membership.
And then asking ChatGPT to recommend a list of products targeting persona 1, which generated the sequence of suggestions below –
Fitness tracker or smartwatch, athletic shoes, resistance bands, foam roller or massage ball, workout apparel, protein supplements, water bottle, and gym bag.
But the fun doesn’t stop there. For example, you can tell ChatGPT that the year is 1950 and ask the advanced chatbot to regenerate the list, which now includes –
Dumbbells or barbells, athletic shoes, boxing gloves, jump rope, stationary bikes, athletic supporter, sweatbands, and weightlifting belt.
Already, we’ve got some useful insight into product line trends today compared with the 1950s. But this is just scratching the surface. You can go far with silicon personas. But you don’t have to explore this product testing and digital marketing landscape alone. Kwame Ferreira, Hugo Alves, and their colleagues started experimenting with AI as a way of synthesizing virtual users to solve one of their biggest pain points – product testing. And not only did the synthetic data work well, it helped elsewhere too. “As we evolved our experiments in AI it became clearer that we were getting a lot of value in the product discovery phase,” comments the team, which has opened the process as a beta version dubbed ‘Synthetic Users’.
If you have accurate backstories for your clients and customers, there’s a good chance that you’ll be able to put LLM-powered silicon personas to work to deliver user testing data or marketing insight that would otherwise have taken hours, days, or even weeks to collate. And, not only could you save time on your product research, you’ll likely save money too.