Autobrains builds scalable approach to realizing AV ambitions
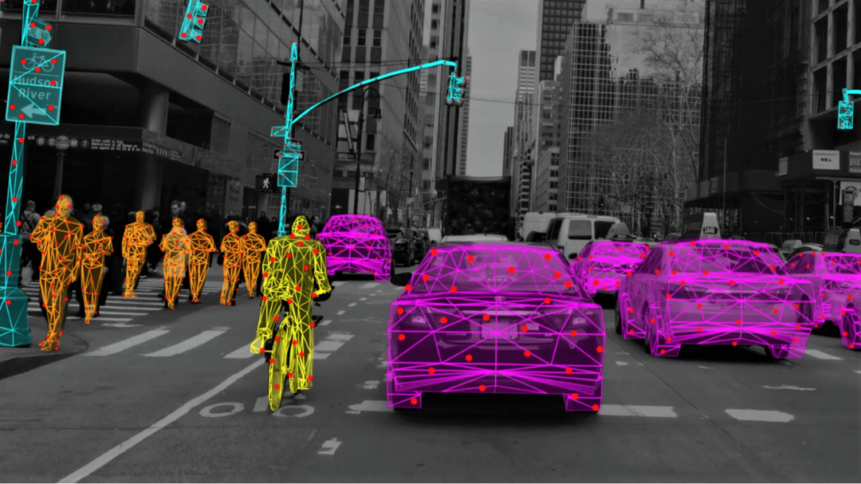
Getting your Trinity Audio player ready...
|
To understand how the bubble of excitement surrounding autonomous vehicles (AVs) got so large, it’s worth rewinding to 1997. Articles often cite DARPA’s Grand Challenge – a series of competitions with million dollar plus prizes for AV designs capable of self-navigating tricky desert terrain – as the catalyst for today’s AV developers. But years earlier, Volkswagen’s ambitions to automate its endurance testing produced some compelling results. The German car maker puts its vehicles through a rigorous program of testing around a purpose-built track at headquarters in Wolfsburg, which can add up to 10,000 miles of driving. And by the end of the project, its team had managed to place a robot in the driving seat, capable of changing gears faster than any human (check out the Autonocast #270 to listen to the fascinating story in full). But more than a decade and a half later, developers are still struggling to get AVs to fulfil their full potential on the road. And that points to a rethink, which is where Autobrains fits in.
In recent times, many long-term AV developers have either pulled the plug or scaled back their self-driving ambitions. Argo AI – which Ford and Volkswagen backed (in 2017 and 2020, respectively) – ended its AV journey at the tail end of 2022. And Tesla, which many hold up as a leader in automated driving (even though its features are some way off fulfilling full AV specifications), had to recall hundreds of thousands of vehicles in February 2022 due to concerns that its software could ‘infringe upon local traffic laws’. Getting AVs up to speed on the roads has turned out to be much harder than doing laps of a test track. And Joachim Langenwalter – Senior VP of Autonomous Driving at Autobrains, who has previously held roles at Stellantis, Nvidia, and other industry heavyweights – describes the issue as ‘hitting the supervised learning wall’.
Ever since supervised learning showed its first signs of image recognition success, artificial intelligence (AI) teams have fed systems with larger and larger data sets to ramp up on the rewards. But in an AV context, this is a massive task. Self-driving algorithms need to digest many millions of images, which need to be painstakingly labeled. And any gaps in that data can prove to be costly if a vehicle runs into something that its models have never seen before. To cater for these so-called ‘edge cases’ developers have rolled in other approaches, such as using synthetic data generated by digital twins.
Fascinating to watch
From a technology perspective, it’s fascinating to watch convolutional neural networks self-driving their way around digital cities. But automotive industry veterans will likely be thinking one thing – cost. What price point will these AV systems arrive at, and who’ll be able to afford them? “Economically, they are not viable at the moment,” Langenwalter told TechHQ. “L3 is already too expensive.”
Automotive automation spans a range from L0 (momentary driver assistance such as lane departure warning) to L5 (where the AV system is capable of handling all tasks and vehicle occupants need only be passengers). But as industry veteran Langenwalter and his peers are aware, the automotive sector has become stuck at the halfway point, with most of today’s systems badged at L2+. What’s more, not only do AV developers taking the supervised learning route have to shoulder large training costs, systems that make it onto vehicles typically need a lot of compute and memory to process their results.
And (not that the AV sector needs any further barriers to climb) there’s the question of vehicle software assurance. If an AI system is a black box, how do you look inside? Software developers are used to stepping through lines of code as they carry out their reviews, but AI is different. The US National Institute of Standards and Technology (NIST) has commented that the testing of autonomous systems remains an unsolved problem. And the upshot of all of this head-scratching is that it’s time for a change.
“Eventually, OEM’s need to have a viable business, and build a scalable approach,” Hilla Tavor – Chief Business Officer at Autobrains, and, along with Langenwalter, another new recruit at the firm – told TechHQ. Tavor joins the Israeli tech start up from Mobileye, which has had rich success turning camera technology into popular ADAS products. And Tavor’s background hints at the bright future that Autobrains sees in ADAS development.
Welcome news
One of the distinguishing features about the methodology being deployed at Autobrains is that it relies on unsupervised learning. “Our solutions come close to zero labelling,” said Langenwalter. “And we can find out what’s not working and trace it.” At a high level, these details will be welcome news to automotive industry executives concerned about data costs and software assurance of AV systems. And the tech firm’s backers include famous automotive names such as BMW, Toyota Ventures, and Continental.
Hilla and Langenwalter point out that there are opportunities to scale up the success in ADAS – for example, it’s possible to deploy front facing cameras at other locations on the vehicle. And, the firm’s signature-based algorithms can run on much smaller chips in comparison to systems that have to support conventional AV models. The AV sector may be changing lanes in its approach, but that shouldn’t take away from the potential for future success.