Synthetic data for AI fills gaps in edge cases
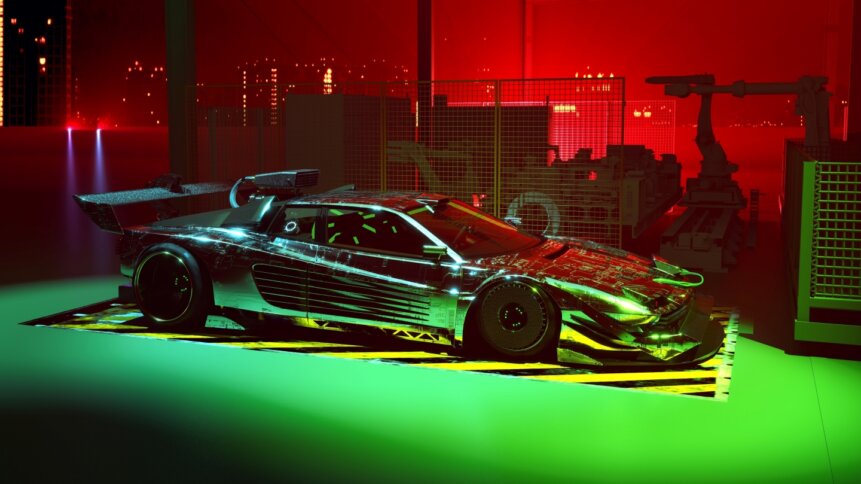
Deep learning has pushed the capabilities of artificial intelligence to new levels, but there are still some kinks to straighten out. Particularly in safety-critical applications such as self-driving cars. If an artificial intelligence (AI) recommendation engine gets its predictions wrong and puts a strange advert in your browser window, you might raise an eyebrow. But no long-term damage would have been done. Things are very different of course when algorithms get behind the wheel and encounter something they’ve never seen before. Rare events, or edge cases, present a tricky problem for developers of autonomous vehicles. Fortunately, synthetic data for AI – based on lifelike simulations of real-world events – could help to fill in the gaps.
No pixels were harmed during filming
The ability to create computer-generated images that wow movie audiences can be repurposed. Instead of bringing aliens to life, digital tools can, for example, create a vast array of unlikely, but theoretically possible automotive scenarios. Such unexpected events could include a truck and trailer upended on the highway. And then this synthetic data for AI can be deployed to put autonomous driving systems safely to the test.
Engineering programs can be used too. Computers have become highly capable of running physical models to test building designs and the integrity of other mechanical structures. And this functionality can be applied to generate lifelike data for autonomous driving AI algorithms to ingest. Clustering tools can compare the synthetic data with information gathered from the real world to check that both sets are comparable.
Synthetic data for AI is helping autonomous vehicle developers to accelerate their training programs by allowing algorithms to road-test their abilities 24-7 in a purely digital environment. Examples include solutions such as NVIDIA’s Omniverse Replicator. The tool allows users to augment real-world environments with digitally rendered scenarios such as a layer of thick snow covering the road, obscuring street signs. Another illustration of its capabilities is to digitally simulate a child running into the road chasing after a ball.
Cost-benefit analysis
Developers could, of course, use crash test dummies and various props to achieve the same thing, but the time and expense of doing so are much inflated compared with deploying synthetic data. Plus, if things went wrong, you’d risk damaging the vehicle and its sensors, whereas in a simulated environment everything can be simply refreshed and rerun.
Firms such as Synthesis AI have shown how synthetic data can be used to test the effectiveness of driver safety monitoring systems. These tools work by tracking the driver’s face to identify signs of drowsiness or distraction. Output can be linked to advanced driver-assistance systems (ADAS) – for example, to prime pre-crash mechanisms if the safety monitoring alerts fail to trigger a response from the driver.
Naturally, developers wouldn’t ask a test driver to fall asleep at the wheel on purpose – as a vehicle speeds along the road – so that they could put a potential facial detection algorithm (and the mitigations that go with it) to the test. Instead, synthetic data can be deployed. Synthesis AI points out that even 5-10 seconds of sleep – defined as a ‘microsleep episode’ – can be sufficient to cause an accident, so driver safety monitoring systems need to be capable of responding quickly and accurately to triggers. Changes in steering patterns can be one sign that the driver is becoming drowsy. But, more recently, using vision systems capable of differentiating between blinking and sleeping has gained interest as warning signs could potentially be gathered sooner. Facial expressions too can reveal signs of drowsiness.
READ NEXT
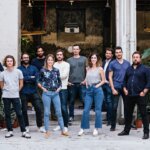
Tech training gets BNPL reboot
To help driver safety monitoring systems developers, Synthesis AI has built a service called FaceAPI. The tool allows users to create millions of unique 3D driver models with different facial expressions “FaceAPI is already able to produce a wide variety of emotions, including, of course, closed eyes and drowsiness,” write the creators. Expanding on the capabilities of the synthetic data-generating software, the model can also represent a driver looking down at their phone or turning to talk to a passenger rather than focus on the road ahead.
Head start
The availability of realistic synthetic data for AI can give firms a helping hand in entering markets where competitors may hold large datasets that would otherwise provide a high barrier to entry. Making it straightforward for start-ups to generate useful AI training sets based on synthetic data gives newer companies the capacity to quickly build momentum without needing to invest large amounts of capital.
Synthetic data goes beyond just recreating scenarios that would be problematic in the real-world. The concept gives AI developers much more scope to dig into new real-world areas where training data would ordinarily be expensive, time-consuming, or both, to collect at scale. Analyst firm Gartner is bullish on the growth of synthetic data for AI. The company forecasts that – over the next decade – synthetic data will become the main form of data used in AI.