How can HR policies tackle AI bias?
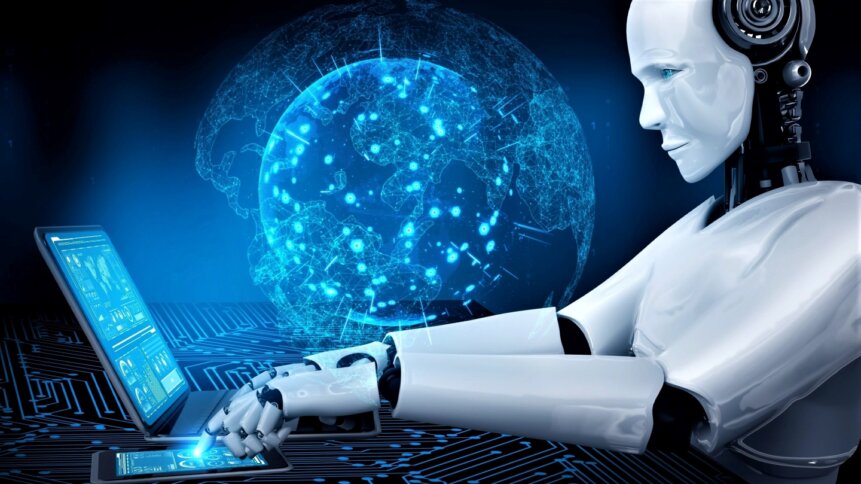
In the news, conversations around artificial intelligence (AI) are often prompted by edge cases such as self-driving cars careering off the road. Whereas, in reality, AI affects our lives in subtler ways that can go unnoticed. Back in 2019, the Brookings Institution – a think tank based in the US– noted that ‘private and public sectors are increasingly turning to AI systems and machine learning algorithms to automate simple and complex decision-making processes’. And this needs to be considered too, particularly in terms of the rising use of AI in HR.
Five years ago, when Oracle surveyed more than 1500 executives – a group comprising mostly of HR professionals, with the remainder working in finance or general managers – almost two-thirds of respondents claimed to be using AI in some form to provide HR analytics. Today, that number could be fast approaching 100%. But why the concern? In HR, AI has big selling points. CV screening software saves staff from having to pore over hundreds of resumes and AI chatbots can reduce the number of queries that departments have to respond to directly – to give just a couple of examples. The problem is bias.
Research conducted by Aylin Caliskan and colleagues, which was published in Science in 2017, showed that the word associations learned by machines from written texts mirror the associations made by humans. To give an example, humans typically have a negative perception of insects and so machines will also adopt that point of view – even though there’s no reason for a computer to dislike them. As AI algorithms crawl through vast data sets, the human bias that’s present in the information is projected onto the machines.
Quantifying bias
It may not come as any surprise that AI models, based on text written by humans, are biased. But the clever part about Caliskan’s research is that the team was able to show the degree to which the data was skewed. And that paves the way for users to gain more transparency on how AI-based HR systems, for example, are getting things wrong. At the heart of the 2017 study is the so-called ‘implicit association test’ – a groundbreaking social science tool introduced in the late 1990s that measures how rapidly people group words together. The quicker the association is made, the stronger the potential bias.
In an AI model, words are recorded as multidimensional vectors, which makes it straightforward for computer scientists to compare the similarity of different elements. The researchers were able to take pairs of words that were strongly associated, rightly or wrongly, by humans and quantify whether that connection persisted in the AI-generated model. To the team’s surprise, they observed a significant result for every implicit association test that they applied to the model – confirming that machines can be just as biased as humans.
The result doesn’t necessarily invalidate the use of AI and machine learning models in HR systems, or across other job functions. But it definitely highlights that users need to know the properties of the data sets that their AI models are based on. And the good news is that today, there are tools that can help. Etiq AI – which is part of The University of Edinburgh’s AI Accelerator program – provides software that’s capable of delving deep into the weeds.
Lifting the lid on black box AI
As Etiq AI’s developers explain, their analytical tools allow data firms to run tests on their AI and machine learning models as they develop them. This allows operators to correct errors, demonstrate compliance, and – importantly – eradicate unintended bias. In fact, the tool can even pinpoint which customers would currently be affected by any bias that had been learned by the machine.
One of the big downsides for businesses that use AI is that, in many cases, models are black boxes and clients have little idea of how inputs map to outputs. Tools, such as those offered by Etiq AI, and other providers, give companies access to that missing part of the puzzle. Being able to demonstrate to users how AI makes its decisions, starts to build trust in the business operations that automated products support.
AI needs to come with certification that models have been scrutinized. Otherwise, the biases and other quirks hidden in the data could have unintended results. Another study published in Science, this time in 2019, found that commercial algorithms used to guide health decisions underserved Black patients. Because the algorithm used health costs as a proxy for health needs, it falsely concluded that Black patients were healthier than equally sick White patients. “Reformulating the algorithm so that it no longer uses costs as a proxy for needs eliminates the racial bias in predicting who needs extra care,” writes the University of Chicago Booth School of Business team in its research.
Data screening tools are a big step forward for the fairer use of AI in business operations and provide a starting point for how HR policies can tackle AI bias.