Stefan Greifeneder tells us more about Dynatrace’s plans for the future
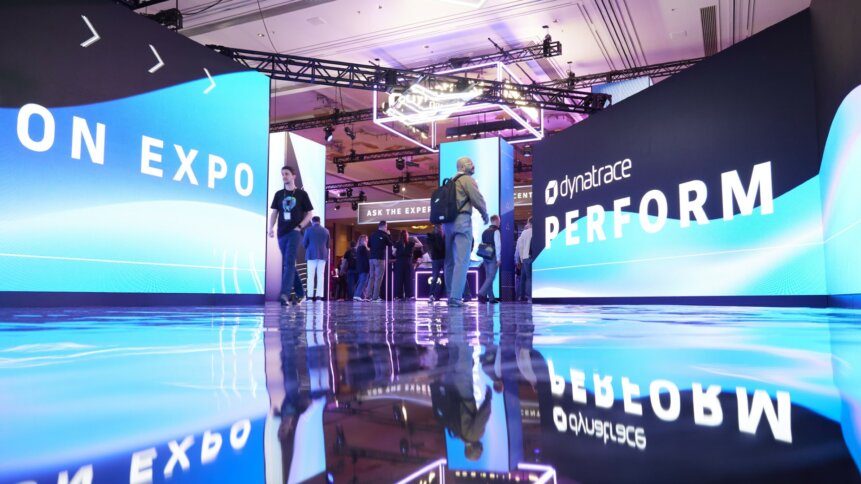
• The drive towards carbon neutrality is a key business goal in 2024.
• Many carbon calculators though are vague and of limited value.
• Dynatrace is working to deliver actionable clarity on carbon neutrality.
On achieving carbon neutrality and giving GenAI the right information: we spoke with Stefan Greifeneder, VP of product management at Dynatrace, about the waves the company is making at Perform 2024.
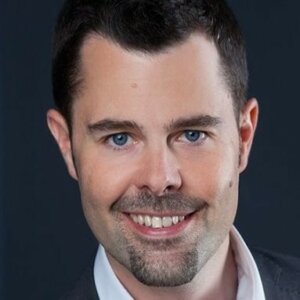
Stefan Greifeneder, Dynatrace.
A major announcement was the addition of AI observability to the Dynatrace stack, while Davis, the existing AI solution at Dynatrace, got a GenAI upgrade.
Can you summarize what differentiates Davis AI from the static of the last year that was all about AI?
The core differentiation is that in our space of stability, one of the core challenges is the case of errors, pinpointing root causes, things that require precision. Before GenAI, we were using our solution Davis AI to do exactly that.
The differentiation here is that we’re combining parts of AI that we already used, which we call predictive and causal AI. The core of Davis AI is this causality thing, because when talking about customers facing problems in big cloud environments in IT and software, it’s massively complex, there are lots of data points, lots of different components.
If something goes wrong, if something breaks, then it’s not just one thing broken but a chain of events.
What we have, due on the one hand to our causal AI and how we collect the data, is an understanding of the dependencies between components. So, we understand how things relate to each other. And we can follow that path; this is something that’s only possible if you understand those dependencies, if you have this information available.
It can’t be done, and not at that precision, with pure GenAI approaches. That’s why we use what we call hypermodal AI, meaning we mix three types [of AI]. That’s predictive AI for precise predictions based on available data, causal AI that’s following pathways and then generative AI, which we use going forward for, for example, remediation recommendations.
What’s your answer to the lack of trust in AI? Do you think observability can solve that?
Good question. What we’re doing right now in our solution to help our customers resolve issues, is not yet using generative AI.
What we’re doing with the predictive and causal parts of AI is following a white paper approach. We have precisely documented what we’re doing. We even show visually in the product how we came to each conclusion – you could think of it as explainable AI.
What we’re doing right now can be easily reconstructed or reproduced; it’s a kind of deterministic result. It’s the precise result, not just the correlation. This is why for those use cases in our customer base, we feel high trust. All of our customers have been using Davis AI since we’ve had it – the trust there is very high.
Now, if we talk about that, specifically, I think this is about building trust. But it’s also about having the right information available for the generative AI to come to good conclusions. Imagine asking generative AI “show me my revenue of application XYZ yesterday.” It would be hard to get a precise answer. But if you weave in the data we have combined with our causal AI then we can engineer the prompt and ask much more precise questions.
In the end, if you use it successfully a couple of times then the trust will be there. Generative AI is more blackbox than predictive and causal AI are, so by mixing it I think the trust is higher.
Dynatrace is a very technical company. Do you find you get better traction with systems engineers than the non-technical folk?
We see the go-to market motion, the sales conversations, at different flight altitudes. It really depends who you’re talking to. For practitioners, for the engineers, for the tech guys, we of course have more technical stories about the immediate value of Dynatrace when they start using it for their specific use case.
When we go up the food chain in the company, the message changes. Our real strength is helping people who are responsible for many areas. It benefits the engineer, but the real strength is for the CIO who can resolve a lot of problems across the organization.
I think for value messages there’s no technical understanding necessary because ROI, TCO discussions or customer satisfaction all come without technical knowledge. Where technology comes into it is in understanding the differentiation we have.
Some of the messages we and some competitors have are pretty similar, to be honest: everybody’s talking about AI and so on. To really understand what the Dynatrace difference is requires some technical understanding.
The partnership with Lloyds banking group to help cut carbon emissions – where do you see that going?
We all know ESG is a globally critical topic. Everyone’s looking at new regulations and it’s a very, very important issue. In our customer base, I think there are two angles. One is the ESG angle, carbon neutrality for example. The other one is cloud cost — those are tied tightly together, right?
What we’re doing with Lloyds is one example of what customers can choose to do; if they’re already using Dynatrace broadly, they get [the carbon impact data] on top – it’s not an additional priced item.
In comparison to other solutions, using Dynatrace means not only understanding carbon impact but optimizing it. You can see where you stand but also drill down and understand which applications, which parts of the infrastructure of your cloud are contributing in which way to your carbon footprint.
We see a great appetite in our customer base to apply it to their own companies.
If you head to the Dynatrace website, you can see the Carbon Impact demo for yourself.