A conversation with Dynatrace’s CTO
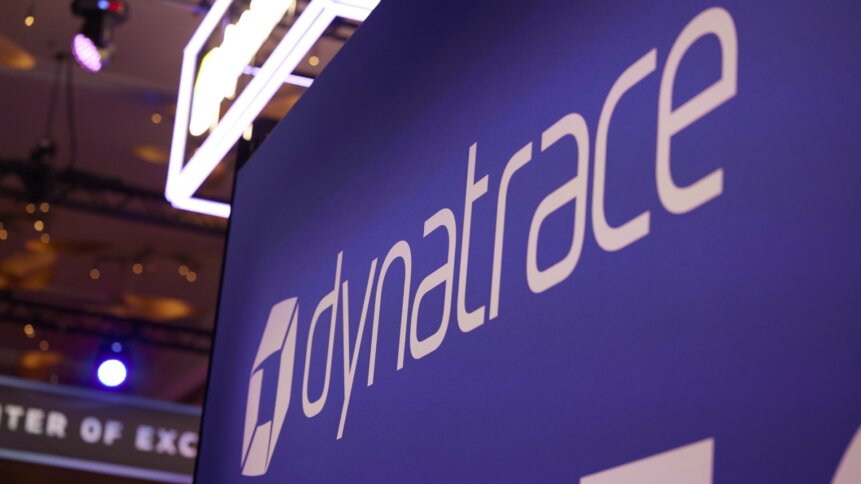
• Dynatrace can now deploy causal AI to deliver certainty of results.
• This fits a particular niche of need for enterprises that GenAI can’t deliver.
• It’s also delivering a carbon calculator that goes beyond standard, vague models.
From causal AI to harsh deletion; after a run of exciting announcements at Perform 2024, we spoke to Dynatrace’s CTO and co-founder, Bernd Greifeneder, to get some insight on the technology behind the observability platform.
As the “tech guy,” how do you approach the marketing side of things? How do you get across the importance of Dynatrace to those who don’t “get” the tech?
Right now we are on that journey – actually, this Perform is the first one explicitly messaging to executives. It’s worked out great, I’m getting fantastic feedback. We also ran breakout sessions with Q&A’s on this three by three matrix to drive innovation by topics like business analytics, cloud modernization and user experience.
Then, we have the cost optimization because every executive needs to fund something. I can explain ten ways to reduce tool sprawl alone with Dynatrace. Cloud cost coupled with carbon is obviously a big topic, and the third layer is risk mitigation.
No one can afford an outage, no one can afford a security breach – we help with both.
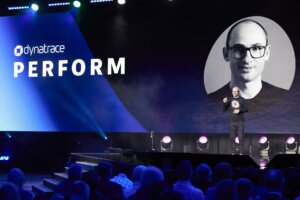
Bernd Greifeneder presented Dynatrace’s new products on the mainstage at Perform 2024.
Executives have always asked me how to get to the next level of use cases. I think that’s another opportunity; in the past we were mostly focused on middle management. If we first give executives the value proposition, they can go down to the next level of scale, implementing the use cases they wanted.
The other aspect is extending to the left. It’s more than bridging development with middle management, because you can’t leave it just to developers. You still need DevOps and platform engineering to maintain consistency and think about the bigger picture. Otherwise it’s a disaster!
How has changing governance around data sovereignty affected Dynatrace clients – if at all?
[At Perform 2024, Bernd announced Dynatrace OpenPipeline, a single pipeline for petabyte-scale ingestion of data into the Dynatrace platform, fuelling secure and cost-effective analytics, AI, and automation – THQ.]
Well, we have lots of engagements on the data side – governance and privacy. For instance, with OpenPipeline it’s all about privacy because when customers just collect data it’s hard to avoid it being transported.
It’s best not to capture or store it, but in a production environment you have to. We can qualify out the data at our agent level and maintain interest in it throughout the pipeline. We have detection of what is sensitive data to ensure it isn’t stored – when it is, say if analytics require it to be, you have custom account names on the platform.
That means you can inform specific customers when an issue was found and fixed, but still have proper access control.
We also allow harsh deletion; the competition offers soft deletion only. The difference is that although soft deletion marks something as deleted, it’s still actually there.
Dynatrace’s hard deletion enables the highest standard of compliance in data privacy. Obviously, in the bigger scheme of Grail in the platform, we have lots of certifications from HIPAA and others on data governance and data privacy.
[Dynatrace has used AI on its platform for years; this year it’s adding a genAI assistant to the stack and introducing an AI observability platform for their customers – THQ.]
What makes your use of AI different from what’s already out there? How are you working to dispel mistrust?
Would you want to get into an autonomous car run by ChatGPT? Of course not, we don’t trust it. You never know what’s coming – and that’s exactly the issue. That’s why Dynatrace’s Davis hypermodal AI is a combination of predictive, causal and generative AI.
Generative AI is the latest addition to Davis, intended as an assistant for humans, not to drive automation. The issue is the indeterminism of GenAI: you never know what you’ll get, and you can’t repeat the same thing with it over and over. That’s why you can’t automate with it, or at least automate in the sense of driving a car.
What does it mean then for running operations? For a company, isn’t this like driving a car? It can’t go down, it can’t be insecure, it can’t be too risky. This is where causal AI is the exact opposite of nondeterministic, meaning Dynatrace’s Davis causal AI produces the same results over and over, if given the same prompts.
It’s based on actual facts. It’s about causation not correlation, really inferring. In realtime, a graph is created so you can clearly see dependencies.
For example, you can identify the database that had a leak and caused a password to be compromised and know for certain that a problem arose from this – that’s the precision only causal AI provides.
Generative AI might be able to identify a high probability that the database leak caused the issue, but it would also think maybe it came from that other source.
This is also why all the automation that Dynatrace does is based on such high-quality data. The key differentiator is the contextual analytics. We feed this high-quality, contextual data into Davis and causal AI helps drive standard automation so customers can run their production environments in a way that lets them sleep well.
Observability is another way of building that trust – your AI observability platform lets customers see where it’s implemented and where it isn’t working.
Yeah, customers are starting to implement in the hope that generative AI will solve problems for them. With a lot of it, no one really knows how helpful it is. We know from ChatGPT that there is some value there, but you need to observe it because you never know what it’s doing.
Because of its nondeterministic nature, you never know what it’s doing performance wise and cost wise, output wise.
What about the partnership with Lloyds? Where do you see that going?
Especially for Dynatrace, the topic of sustainability and FinOps go hand in hand and continue to rapidly grow. We’ve also implemented sophisticated algorithms to precisely calculate carbon, which is really challenging.
Here’s a story that demonstrates how challenging it is: enterprise companies need to fulfil stewardship requirements. To do so, they might hire another company that’s known in the market to help with carbon calculation.
But the way they do it is to apply a factor to the amount the enterprise spends with AWS or Google Cloud, say, and provide a lump sum of carbon emissions – how can you optimize that?
The result is totally inaccurate, too, because some companies negotiate better deals with hyperscalers; the money spent doesn’t exactly correlate to usage. You need deep observability to know where the key carbon consumption is, whether those areas truly need to be run the way they are.
We apply that to this detailed, very granular information of millions of monitored entities. With Lloyds, for example, optimization allowed a cut of 75 grams of carbon per user transaction, which ultimately adds up to more and more.
Our full coverage of Dynatrace Perform is here, and in the next part of this article, you can read a conversation with Dynatrace VP of marketing Stefan Greifender.