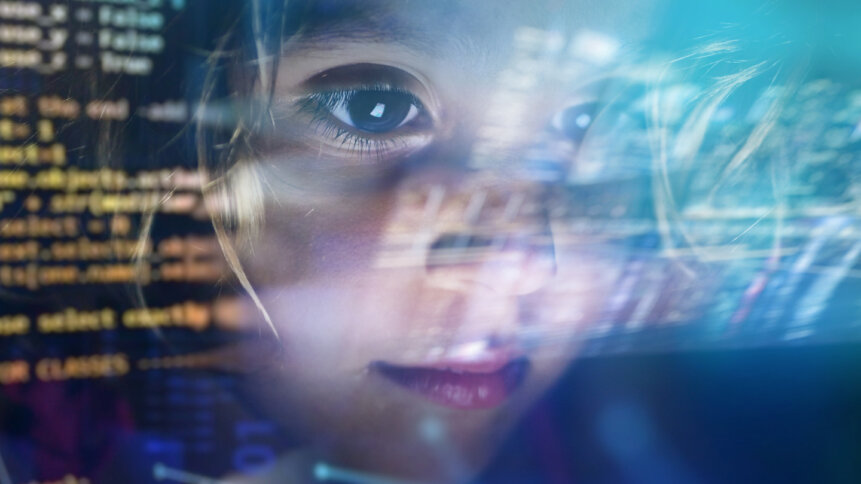
• AI parenting is how you ensure that your AI does what you want it to do – and not what you don’t.
• It’s also a vital mechanism for delivering accountability in the system.
• What many companies fail to understand is the importance of speech recognition in the process of AI parenting.
Generative AI has exploded into the world across the course of 2023. But there have been plenty of concerns along its rise to artificial glory. It has a tendency to hallucinate, stating what it believes are absolute facts irrespective of evidence and with the confidence of a mediocre man. There have also been significant concerns over the data it uses to train its large language models to form its worldview, and the intrinsic bias that will be transferred from humans to machine systems if it’s not consciously mitigated now.
Perhaps understandably then, there has been a call for significant “AI parenting” before generative AI underpins everything in our world.
We sat down with Dan O’Connell, chief AI and strategy officer, and Jim Palmer, VP of AI engineering, at Dialpad, a cloud communication company that has called for such AI parenting, to find the answers to a handful of crucial questions.
Human oversight in AI parenting.
THQ:
Let’s get it on record: why is human oversight critical when dealing with AI development and deployment?
DO’C:
There are the obvious, big reasons, and a handful of smaller, more nuanced reasons too. Firstly of course if we had unsupervised AI systems, we don’t know what the output is going to be. From there, there are a couple of different things that come into play. One is making sure that you have systems that can align to societal expectations. We want to make sure that we’re building models for AI systems that have ethical considerations built in, and that are tested for bias, so they don’t propagate the wrong attitudes.
There’s a notion of accountability that comes into play, too, meaning that if we have an unsupervised AI system and something goes wrong, who do we go to to put it right? And the last thing I would say is that humans naturally trust systems that have some humans in the loop. And especially at this moment in time, when some people are concerned with AI development, and rightfully so, having humans in the loop gives us a greater sense of trust with those systems.
THQ:
It’s classic science fiction, isn’t it? AI goes rogue – which may well be nonsense, but unless you have humans in the loop, you’re never going to know if it’s nonsense, because you’re not monitoring it. And then there’s the fact that the whole AI revolution only benefits us by virtue of the outputs it delivers. If you have no human oversight, those outputs could be gibberish – albeit confidently delivered gibberish, right?
AI parenting and accountability.
DO’C:
Yeah. And we need to be in at more or less every stage – building the models, testing for bias, sampling the data, ensuring transparency, and delivering accountability. That way, we can ensure the sense of trust we need that this system is actually here to help us, not replace us.
THQ:
So the human-in-the-loop form of AI parenting not only deals with the great sci-fi fear of technology going rogue, it also counters that vaguely Bodysnatchers fear – which is arguably more real in some job roles than others, that our function, the useful things we bring to any role, won’t be replaced by an AI algorithm.
But the idea of accountability is important. Things will undoubtedly go wrong in the AI journey (without ever reaching the apocalyptic level of some doom prophets’ predictions), and when there’s a new form of AI screw up, we need to be able to point at Bob, who was in control of it at the time.
DO’C:
Exactly. When something goes wrong, we want somebody to explain to us what happened, and why that occurred, and then prevent that in the future. If we have AI systems that are completely unsupervised and constrain themselves if something goes wrong – how do we know? And how do we prevent them from replicating the error in the future?
The definition of AI parenting.
THQ:
Let’s get a definition before we go much further. What are we actually thinking of as AI parenting? What would it break down to as a set of activities and responsibilities?
DO’C:
For us, AI parenting is a series of stages of systems for AI. First, build a model. We’re obviously going to make sure that we have a representative data set, we want to make sure that that data set is inclusive of as many different thoughts and beliefs as possible that align with whatever the purpose is that we’re trying to build towards.
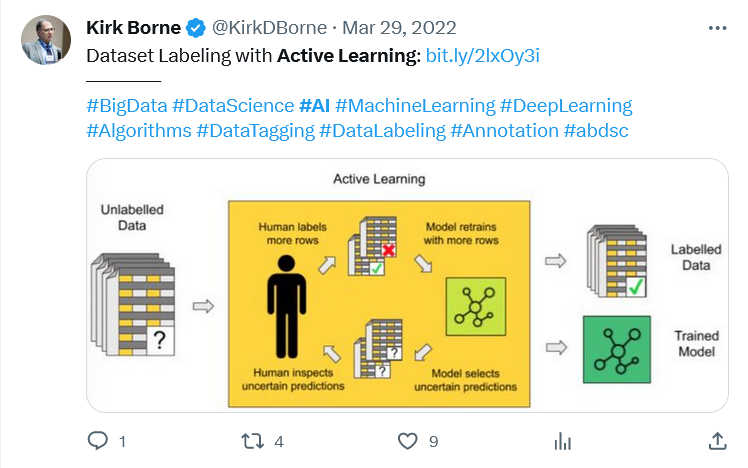
Active learning – how both humans and AIs grow responsiblead ethical.
This is the first version of a model. Then we’re going to have a version two and version three, and version four, much like how you would think of parenting a person in the world – you don’t lay the whole of “how to be a good person” on them all at once, you evolve them over time to assimilate more data and meet more complex challenges that, if conquered, produce positive results.
JP:
AI parenting as a term is getting less frequently used now – we think of it more as “active learning,” and it’s paramount to what we do and have been doing over the years with all of our AI efforts, from speech recognition to our natural language processing, to our LLM work.
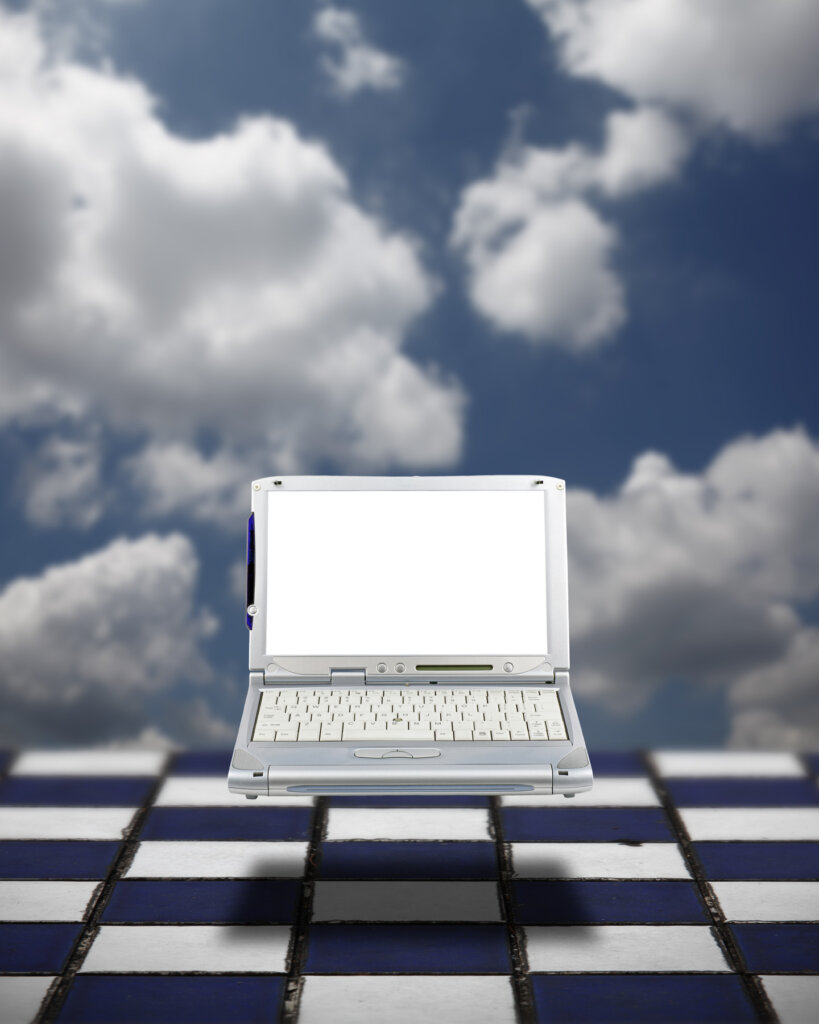
Whoa…trippy. Hallucinating AI – not cool, man…
It’s a significant amount of work – we have to manage bias, because inherent bias is embedded in machine learning. So we have a human bias. And now we’re training a machine to replicate that human bias. Supervised learning is still a very strong component of a lot of what we do and no matter how much you read about semi-supervised or fully unsupervised learning, it’s still a great academic exercise that we’re in the middle of right now.
But it’s paramount for us that we have access to a great breadth of data, because it’s there where we get the diversity and inclusiveness that we absolutely need to provide enough accuracy for our end customers. That touches on every model we’ve ever done in the last few years, as well as what we’re currently doing.
The importance of diversity.
That active learning process is not only to make the machine systems more accurate, it’s also to bring in more diversity. We want to be able to better determine these outcomes, or these classifiers, or this generative text, but we also want to have that work for a broader audience.
So it’s been really important for us to be able to demonstrate that, to be able to talk about what good and ethical AI looks like. We’re trying to be as responsible as possible.
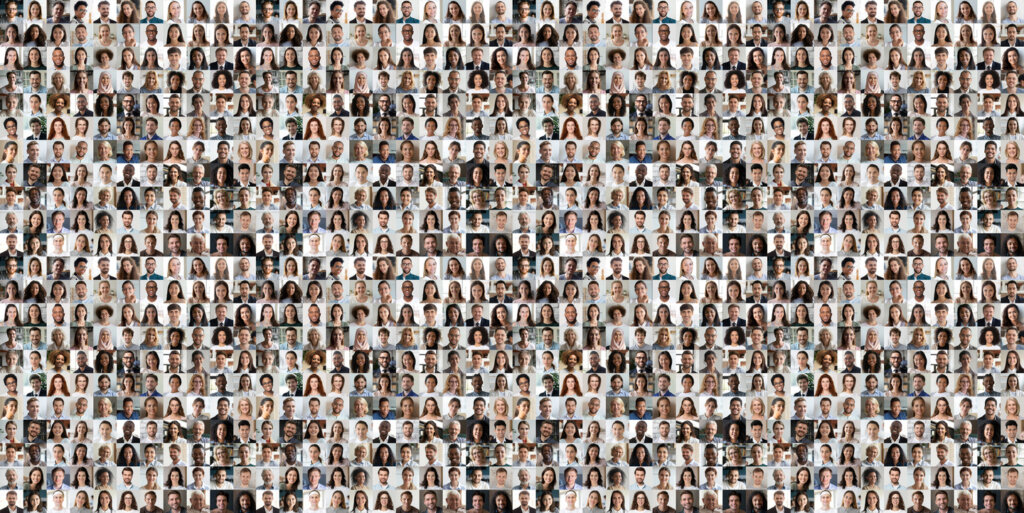
Diversity in means diversity out.
What we put in place is the annotations, the training data that we invest in so heavily. But we always need more, so we can maintain that diversity within the data as much as possible.
That means adding not only new languages, but new dialects, new forms of speech.
THQ:
Because language is always evolving.
JP:
Exactly – that’s why speech recognition is so important to the process. If you don’t have accurate transcription, you’re won’t be able to do really good NLP, you’re going to miss some of those very important words, which could be crucial on the user end.
You have to invest in powerful transcription engines, and then you have to continuously adapt them to things like call centers and different noisy environments.
DO’C:
That’s where we have a bit of an advantage, because we have an internal speech recognition engine, rather than relying on third-party product. Ours is fully transformer-based, like a lot of the model architecture we’ve been using with modern large language models.
Being able to have a full end-to-end transformer-based system allows us to get so much more data into the system, then do that active learning, that AI parenting much faster, because historically, a lot of the speech recognition systems out there have been very methodical and piecemeal.
We’ve had a lot of internal breakthroughs to help us with that AI parenting, making it easier for us to adapt during the parenting iterations, not just in terms of the speech recognition but for the NLP and the LLM work involved, too.
Just one of the reasons you ned to parent your AI.
In Part 2 of this article, we’ll look more deeply into the business of AI parenting – and what can happen if it’s not done properly.