Generative AI applications – a ticking clock for enterprise?
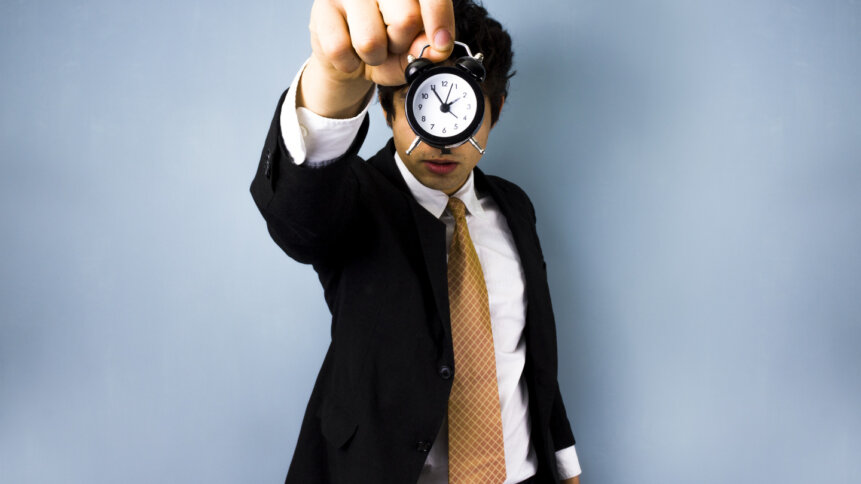
• Businesses are uncertain which applications of generative AI are right for them.
• It’s all too possible to choose the wrong applications for generative AI – and be left behind.
• It’s worth understanding the technology, and focusing on your business’ pain-points.
Whenever any new technology explodes onto the scene in quite the way that generative AI did back in October, 2022, it can cause a kind of skillet-to-the-face moment of paralysis in businesses.
It takes the equivalent of a business minute for enterprises to decide whether the new kid on the block is just a fad that can be ignored while they focus on their core offering, or a real game-changer that needs to be caught, taken on, and used to further or transform the business.
Many businesses are still stuck in that moment of paralysis, two-thirds of a year since generative AI first changed the world as we know it.
Most enterprises have concluded that generative AI is here to stay, and that it’s vital to maximize the usefulness of the technology to the benefit of their businesses, but the paralysis of the “fight or flight” moment persists because in most cases, the enterprises are experts in what they do.
They may lack the in-depth understanding (or the processes for arriving at that understanding) that can help them find the right way to apply the new technology to their existing business model – or indeed to change their business model to accommodate the advantages of generative AI.
Add to that the degree of uncertainty that has dogged large language model generative AI like ChatGPT and Bard – from the hysterical headlines that it will end the world to the more significant questions over exactly where it gets its training data from and the integrity of that data – and it’s increasingly understandable that some enterprises find themselves caught between the urge to action and the fear of getting it wrong.
Get it wrong and enterprises can waste enormous sums of money – and enormous swathes of time – implementing the wrong generative AI applications. But wait too long to decide how generative AI can improve your business, and you could get left behind by the pack of your competitors. It’s a real teeter-totter moment for many enterprises – and you have to get it right.
Generative AI applications – where to begin.
We sat down with Thor Philogéne, CEO of Stravito, a company that specializes in creating insight platforms for enterprises-level businesses, because we happened to know that Thor had just finished implementing the first of Stravito’s major generative AI applications, so he’d be well placed to give us the dos, the don’ts, and the ways to approach the process in a way that works for the business in question.
THQ:
Businesses everywhere are adding generative AI applications to tackle some function or other. What are the main ways that you think the technology can help them?
TP:
The thing about generative AI applications is that everybody wants magic. You see the technology, you let your mind wander, and suddenly it can do everything. But if we take a sober look at it, there are two main streams of value creation – efficiency and productivity.
For example, generative AI will reduce the amount of time spent researching and the amount of time spent analyzing important information, which will then free up time which can be spent on implementation of whatever learnings came out of that analysis. That’s one way in which the technology can increase both efficiency and productivity.
Generative AI applications – boosting user confidence.
THQ:
What’s the trust level in using generative AI to do that sort of research, on the strength of which you then go away and make incredibly important business decisions? And what does the trust level have to be before you can do that? Before you can trust the direction of your enterprise to it?
TP:
Ha. Excellent question. The way we approached it is to assume that it’s both fundamental and crucial. So in the case of Stravito, we not only provide our users with an answer, but we also provide them with sources. So it’s possible for them to go directly and see where that information was pulled from. And because we only use internal data, there’s no risk for any hallucination based on data points that might not have been accurate, that they or their generative AI found on the internet.
THQ:
Is that a core message to enterprises trying to implement their first generative AI applications? Only use data you can trust and trace?
TP:
Yes.
What we currently have, and what our clients are currently using, is something that is based off the data with which they have provided us. And we make sure to highlight issues, because in some cases, there might be different reports that have contradictory views. We highlight those contradictory views. And then we tell them where the AI found the information, with additional verification of all the sources, or as many as are available.
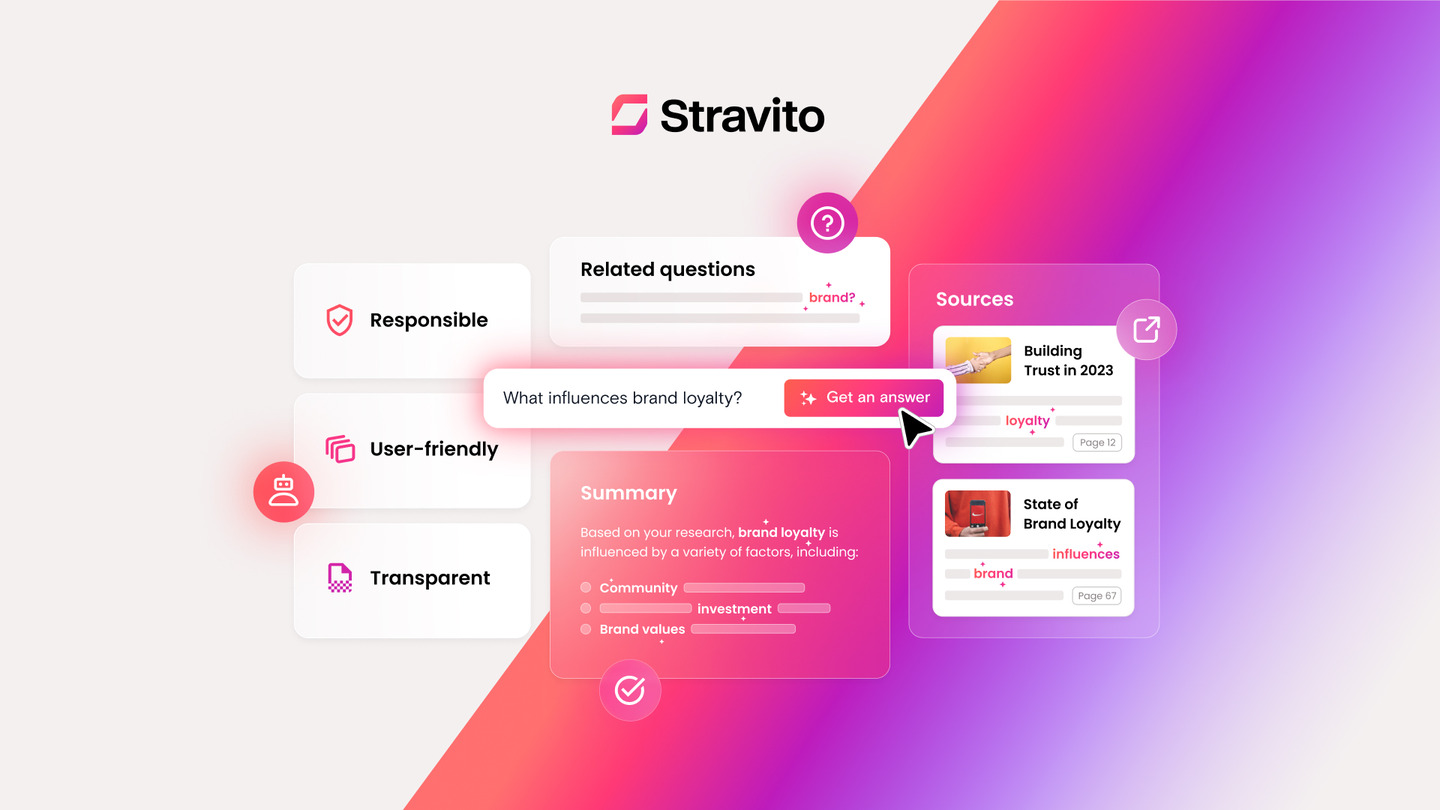
The Stravito model.
YOU MIGHT LIKE
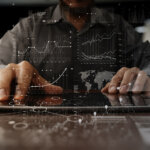
Adding layers of safety to generative AI
THQ:
So that boosts user confidence, and your own confidence as the provider of the generative AI application, that no-one’s going astray from verifiable data. That sounds like a lesson for others to follow.
TP:
Exactly – it’s something that, when they saw it, gave many of our clients such a sense of relief, because as you say, everyone’s aware of the data questions surrounding LLM generative AI applications. It’s enough to terrify people – “Will we get an answer? We don’t know anything. It’s a black box. We don’t know anything of where that answer came from.” So for many of our big enterprise clients, having that pathway to sources has been very well received, and helped to calm some of those understandable fears.
Generative AI applications – the uncertainty principle.
THQ:
That leads us to another big area when it comes to generative AI applications and enterprises.
We know that generative AI can be useful to enterprises in a whole range of ways, particularly the ones that you’ve mentioned. Where do we think the hesitation comes from? The uncertainty of businesses to use it in ways that are emerging, that are safe, rather than, as you say, just going out and attempting to do AI magic?
TP:
I think the hesitancy is relevant because generative AI is still in its early stages of development, which means that a lot of people that are confronted with it are wary of the long-term effects, which is perfectly normal.
I believe it’s essential for the enterprises to focus on real-life pain-points.
Instead of being wowed or overwhelmed by the technology, really think about the real-life pain-points that you can effectively address. And then explore how generative AI tools allow you to address those pain-points.
The point is that while I’m sure there have been conversations in some companies where it’s an unfocused thrust of “We need this, we need generative AI and we need it in this, that, and the other application,” implementing AI simply for the sake of using the technology, without a clear purpose or business case, is bound to lead to inefficient resource allocation – and, in my opinion, limited return on investment.
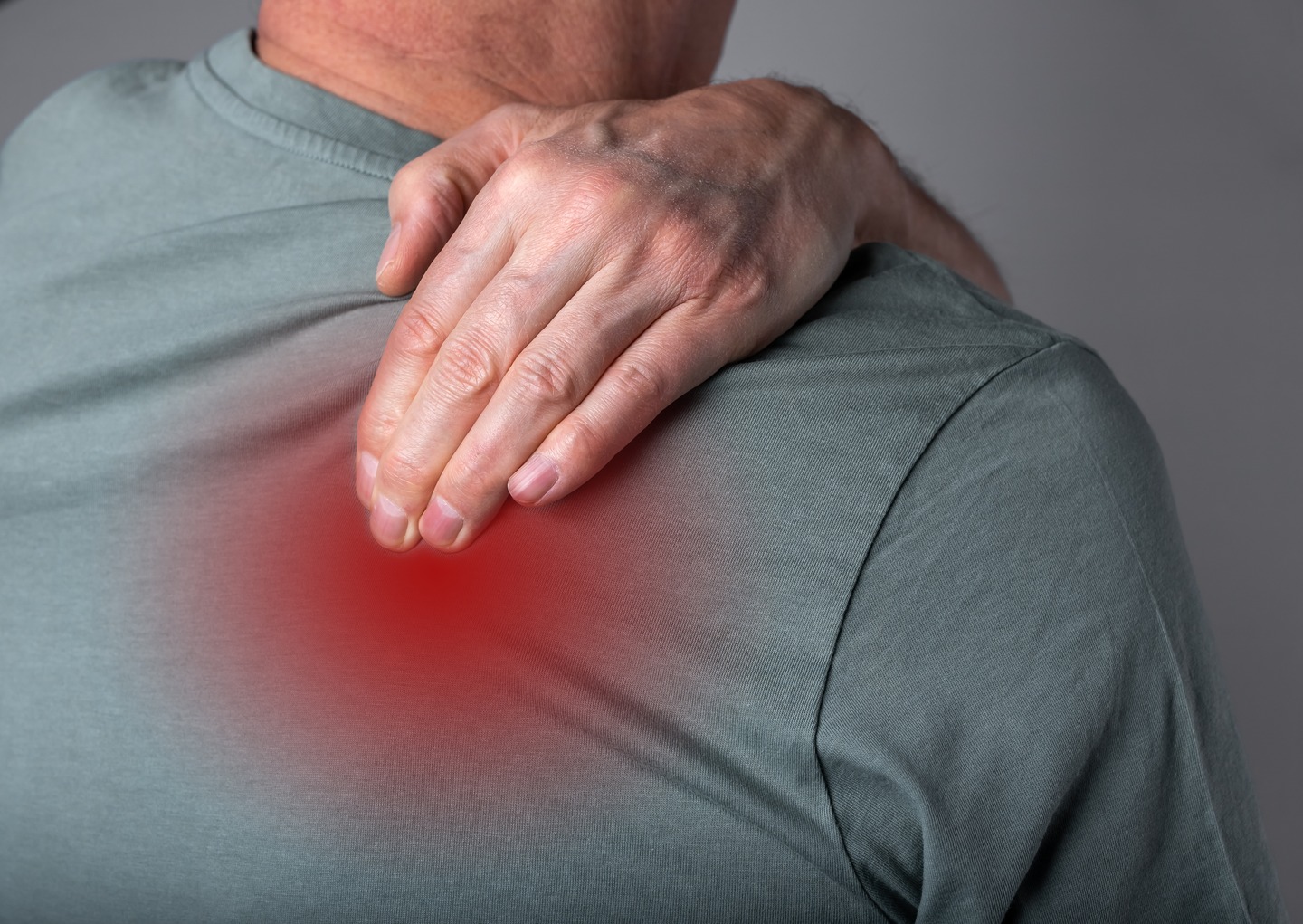
PHYSICAL pain-points are usually easy – and fast – to self-identify. BUSINESS pain-points though can linger unaddressed for years.
THQ:
A typical case of “Know what you have and know what you need before you go shopping”? Know the problems you’re trying to solve, and why you’re trying to solve them, before you go investing money in an omni-solution?
TP:
Absolutely.
Generative AI applications – identifying pain-points.
THQ:
The question is, though, is there as much of a danger in doing that, and jumping to “Oh, we must use generative AI, and use it for whatever” as there is a risk in being too scared to use it at all?
It feels like there’s a balance point at the moment where we have to use it, otherwise, we’ll be left behind. But use it wrong and we’re in that expensive position of overcomplicating our offerings for no tangible business benefit.
TP:
That hits the nail on the head, yes, because ultimately, it’s really about forcing yourself to narrow down where and how you want to apply generative AI applications. Again, I come back to the idea of removing the technology from the equation in the first instance and looking at your business. Looking at it honestly – where are your real-life pain-points?
Generative AI is still in its early stages, so for right now, that feels like the way to think about it.
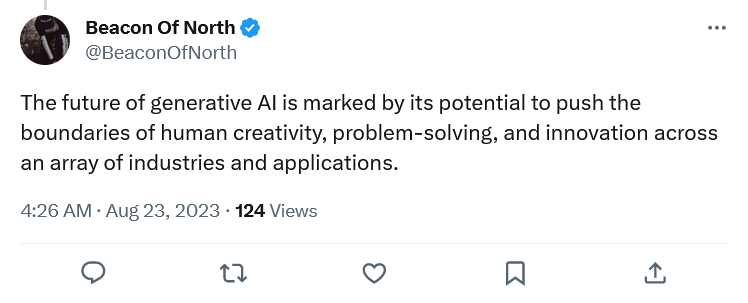
Using generative AI applications can be lucrative – but not necessarily easy.
THQ:
As a technological first aid kit, to ail what hurts you – and your clients or customers – in the experience of your pre-AI business model?
TP:
Exactly. But it’s also important to realize that it’s most likely eventually going to become a fully integrated aspect of many business processes, if not all business processes. So I think as a responsible leader, instead of straying away, you have to take the time to educate yourself on the history of the technology, the proper uses of it, and the best practices of it, before you apply it to your field. And then, with that knowledge, you should be able to decide where to start.
In Part 2 of this article, we explore the potential future of generative AI applications in enterprise, and show how knowing both your pain-points and your technology can pay substantial business dividends.