Adding layers of safety to generative AI
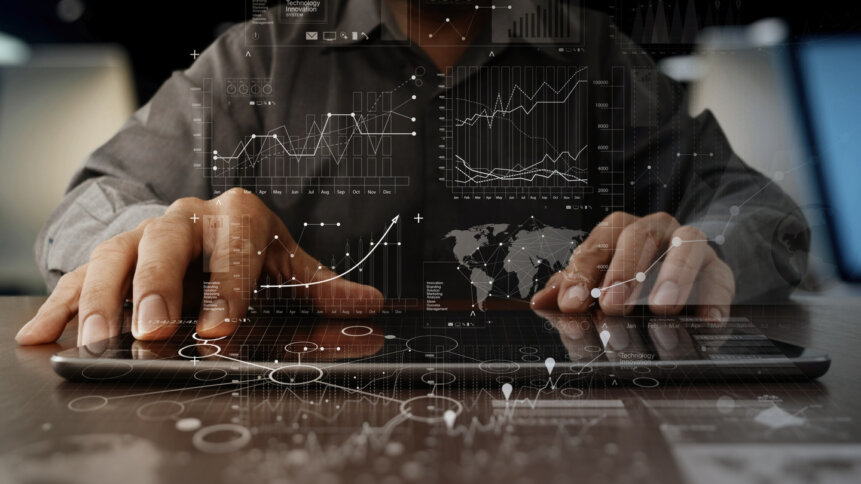
• Generative AI presents data leak challenges for companies.
• Visibility is key to keeping privileged data safe.
• Safety is more important right now than application.
In the quest to make generative AI genuinely safe for use by staff of a company, even when they try to add private or proprietary data into the system (which could then be re-used outside the company’s purview), we’ve been speaking to Rich Davis, Head of Solutions Marketing at Netskope – a company that claims to be able to add layers of safety to the process and stop your data flying out into the wider world of generative AI training.
In Part 1 of this article, we explored the data issues that are stopping many companies from letting their staff use generative AI on a day-to-day basis.
In Part 2, Rich explained the way in which Netskope’s solution to accidental generative AI data leaks worked – giving staff the freedom to explore, but silently (and with significant organizational buy-in) monitoring the data that’s being added to the generative AI, with a step-in option to avoid sensitive data being flung out into the world.
As we came to the end of Part 2 though, we asked Rich about the different layers of protection that are necessary to insure against accidental data leaks.
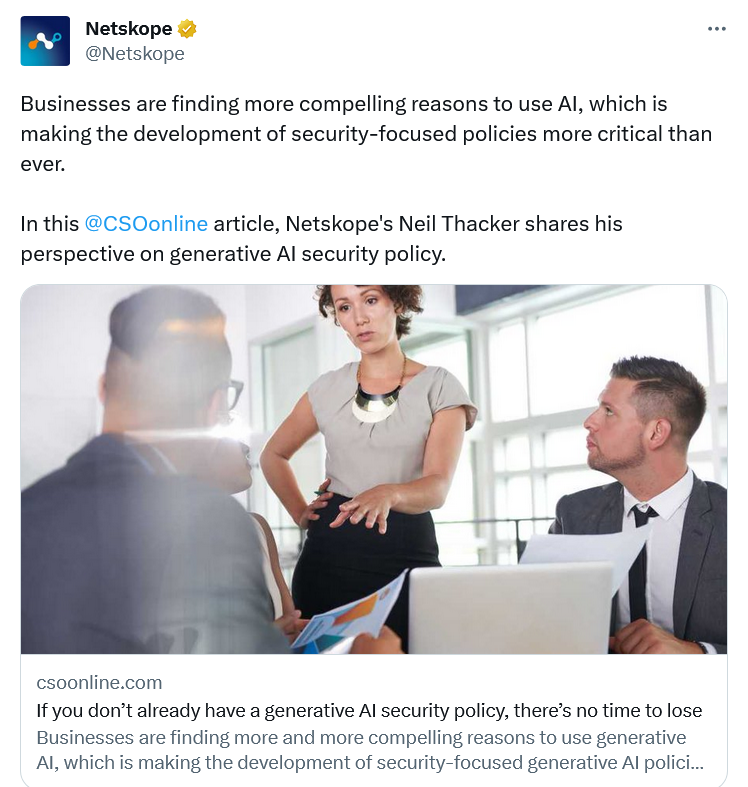
Generative AI is becoming “must-use” technology – as long as you can do it safely.
THQ:
Talk us through the protection methods you use in your system to keep company data safe, while letting people explore what generative AI can do for them.
RD:
Sure. Our solution is designed to be a cloud platform that users use to access any application anywhere. So it’s the same application if you’re using, say, ChatGPT as a cloud service or using an API and integrating it into your own application, or if you’re using one of the open-source versions of generative AI and you’ve built it in your own data in a public cloud – exactly the same.
So that’s the first thing – no matter where the data is going, you’ve got the same analysis, to streamline the operation.
Speaking generative AI’s language.
The second key thing is deep awareness. We currently index around 65,000 SaaS applications with a level of risk score – what we’re going to allow, what we’re going to block and so on, because there are a whole load of applications that are risky for a whole load of reasons – because they haven’t got good data privacy policies, they’ve got bad rotation, maybe they’re actually run by organizations in certain territories that are deemed dangerous.
That’s why that first step is important – allow and block certain applications. The second step is the access control area, which means we can make decisions like “we’re going to allow this, but we need this granular understanding.” That’s been the core of our solution over the last ten years, having this huge application library and the ability to add a new application to that library and fully understand all of that back-end communication channel – in around a day.
So we maintain a constant understanding of how every application talks and uses APIs and JSON to talk to the back end. They all talk in a different way, and you’ve got to understand that structure, otherwise you’ve got no visibility.
Take OneDrive. You want to share documents with somebody in OneDrive, you hit a button in your UI, and you type in the user’s email address. That never comes down to your client and back up. So anything that’s actually looking at data going up and back down, like a traditional upload and download, isn’t going to see anything.
And it’s the same here. Even if you’re typing text in and uploading it, it’s going as part of this JSON packet. To claim visibility, you’ve got to understand that, and you’ve got to understand it across a huge broad-brush spectrum. Every day, a new generative AI app is popping up. And you’ve got to understand that, you’ve got to add it to the library.
The power of data visibility with generative AI.
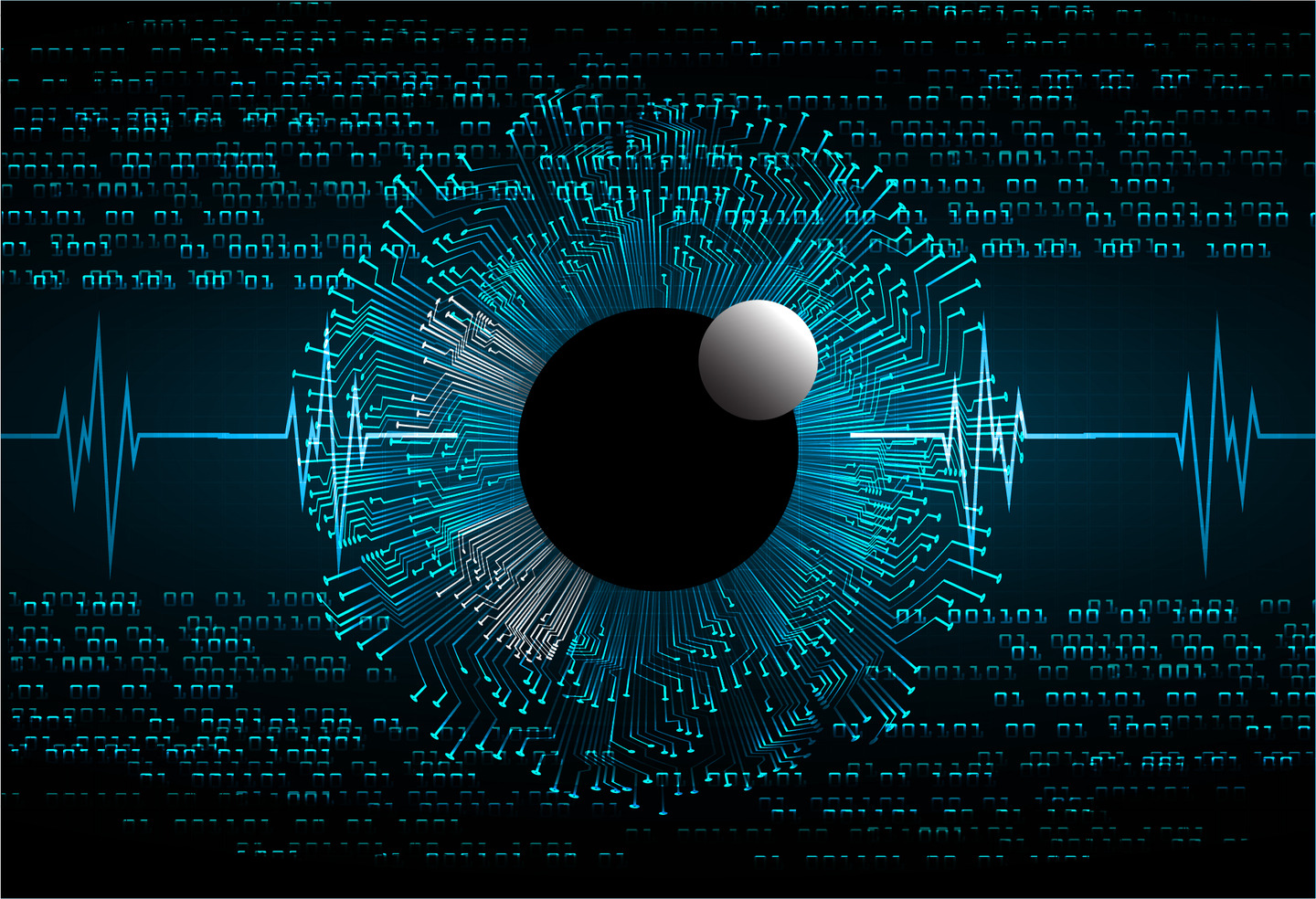
Data visibility is everything when making generative AI business-safe.
That’s why we’ve been able to launch our solution so quickly – our underlying technology has been doing this for years, and a generative AI is just another application you can quickly add to the library to understand.
And once you understand it, all of the other layers that you already have in place around threat detection capabilities using ML and AI-based classifiers, data protection, optical character recognition and so on just power on.
The key thing is being able to see the data. Once you see your data, you can apply technologies that we’ve been using for quite some time to it. Some of those technology layers are more advanced than others in terms of the level of granularity of live data and the level of accuracy.
THQ:
So the granularity of live data lets you spot things that shouldn’t go into generative AI?
YOU MIGHT LIKE
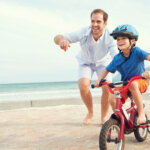
Generative AI – with training wheels?
RD:
Yeah. The top thing we’ve seen being shared within these platforms is source code, followed by intellectual property. Being able to identify source code being uploaded with a high level of accuracy is incredibly important.
Actually, we made an update to our machine learning classifier just last week that looks for source code specifically, to greatly increase the false positive-to-catch rate ratio.
That means we can increase the effectiveness of that source code identification, because that’s what’s top of mind. That’s what’s being used.
THQ:
It seems like lots of enterprises are having to make themselves go on a very steep learning curve, both about the technology of generative AI, and then the dangers of generative AI, and then the solutions to the dangers of generative AI.
It’s building into a giant educational snowball, isn’t it?
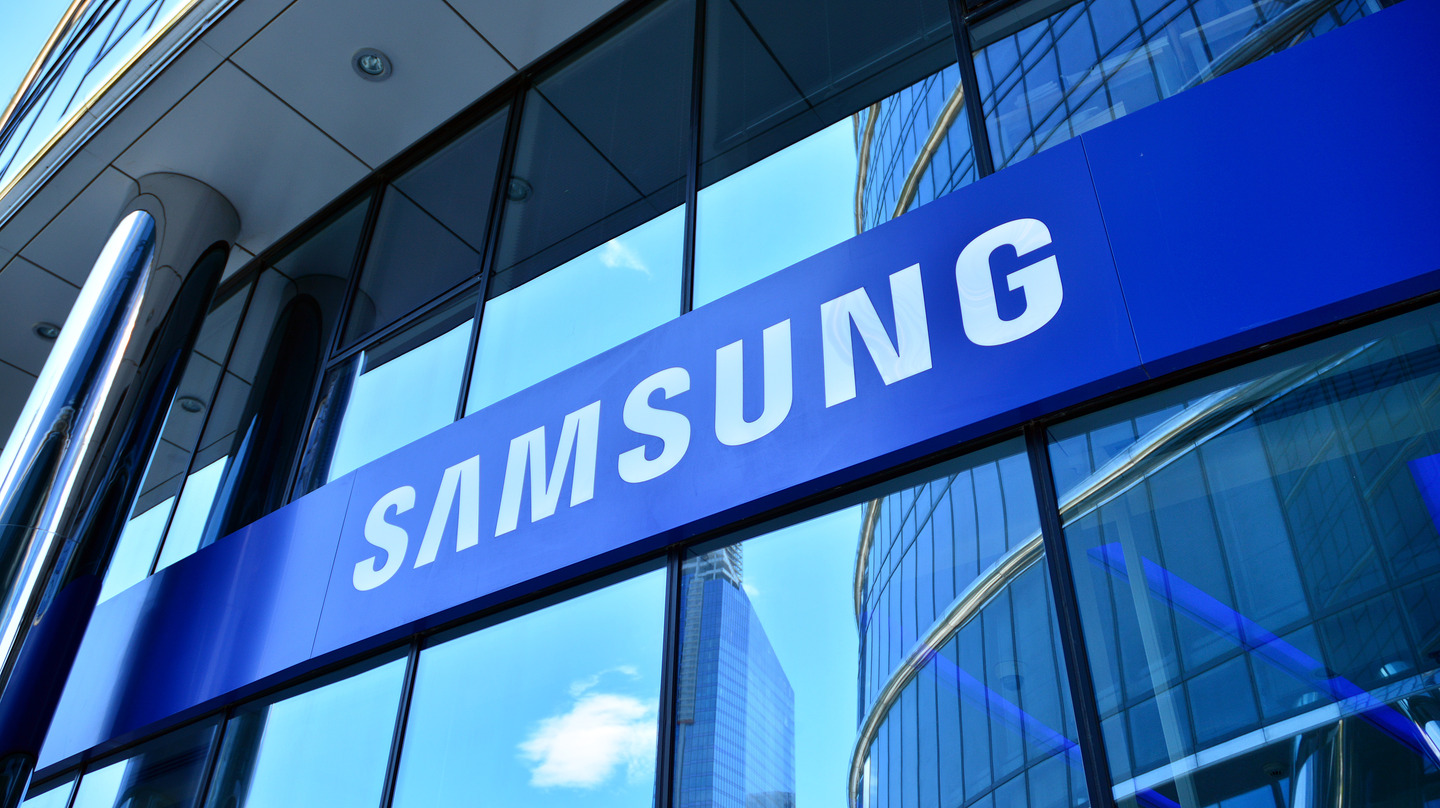
Samsung first really highlighted the data breach dangers of generative AI.
RD:
Exactly, and especially when they’re considering how it’s useful to them, and what they want to actually do with generative AI. Do they want to use the large public models from OpenAI and Microsoft, or Google, or whoever? Or do they want to use a smaller data set? How can they bring that in-house? And how can they train their model? And if they’re doing that, then how do they make sure that it’s also secure? There are multiple threads of conversation going on here, and they have to find the order of their priorities.
And the priority for so many companies I’m talking to is surprising, because it tends to put the “How do we use it?” question second, rather than first.
The first priority of most companies is “How do we make sure that we don’t accidentally lose data right now.”