Knowledge graphs: have supply chain data your way with generative AI
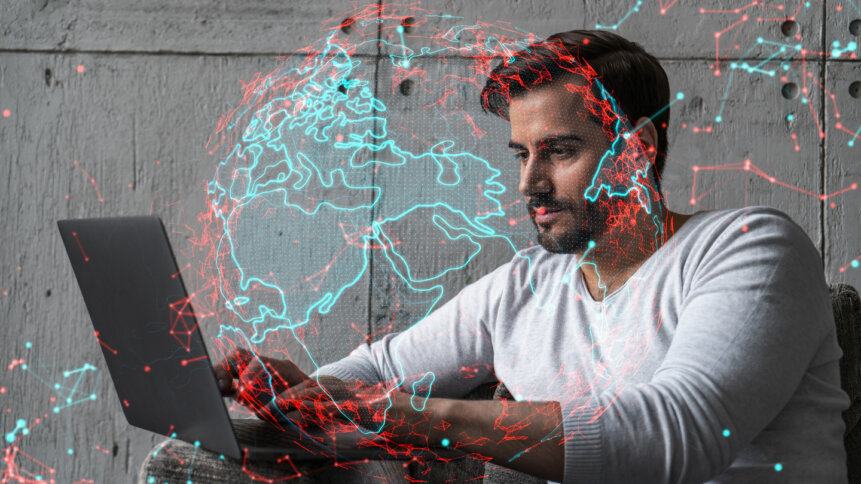
Getting your Trinity Audio player ready...
|
• Knowledge graphs can give you supply chain data visibility fast.
• You can create full data-trees for connected companies.
• You can then interrogate your supply chain data any way you need to.
Everyone knows that generative AI has already changed the world in immeasurable ways. But imagine a world in which you could use generative AI in a highly trained way on your most pertinent supply chain data. Where you could create sophisticated supply chain data-chains that would allow you to slice, dice, interrogate and gain immeasurable insight from your company’s data, that you’d never known was there before.
That’s the proposition behind a technology known as knowledge graphs. Whether you’re looking at business efficiencies, sustainable supply chains, or simply interrogating your supply chain data in a whole new, fine-mesh, comprehensive way to influence the way you steer your business, there’s every chance that knowledge graph technology might be able to help you meet your objectives.
We sat down with Paul Hopton, CTO at Scoutbee, a leading company offering knowledge graph technology to enterprise clients, to get down and dirty in the supply chain data, and see what insights it could give us.
Building a knowledge tree.
THQ:
Knowledge graphs. Pretend this is the first time we’ve heard of them – walk us through. How do they work? What do they do? What makes them better or more useful than traditional data and analytical methods? And above all, what value do they add?
PH:
Knowledge graphs are significantly different from traditional table-based views of a company’s supply chain data.
When everyone was getting excited about domain-driven design and domain driven development, we started looking at how you could use software to describe interesting environments.
And what we found was that you could capture all this knowledge about an area in software. Some of those early efforts really struggled and hit a wall when we tried to store that data, because we were dealing with quite a rigid SQL environment.
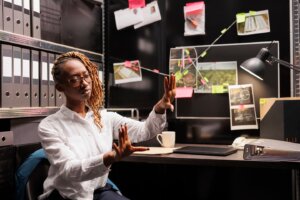
Bringing disconnected data together is an old idea – ask any detective.
You can have different objects represented, and you can have four lookup keys to represent things, but as you add more and more information, and more and more data sources, and as your understanding about the domain shifts and changes over time, it becomes very hard to maintain those traditional database structures.
So we had a big movement of people saying, “Well, let’s just put everything in a lake, let’s just have kind of a massive data lake, and then just try and use things like Map Reduce to go in to scour through the data and find what we’re looking for.
But that requires specialist skill sets to do it properly.
What knowledge graph databases do is provide a different way of representing data, and a much more flexible perspective on what the thing is that you’re looking at.
Attribute DNA forms data nodes and chains.
We look at a supplier and see a bunch of attributes connected to that supplier. Now it could be a product, it could be a capability, maybe it’s a certificate, perhaps it’s an address, and we can actually connect a bunch of these different nodes together, connecting to that point.
The relationship itself can be given a name, and you can give it values, and you can give it a weighting. So you can start to build a much more complex model around that key thing that you’re looking at within your supply chain data.
That allows you to very flexibly build a whole lot of knowledge around that key thing, without having to worry about table joins, database schemas, or doing data transfers.
That’s the key way in which they become valuable.
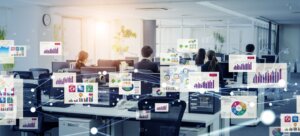
Supply chain data, brought together, powers smart business.
YOU MIGHT LIKE
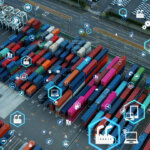
The challenges of Scope 3 data collection
Of course, it’s rare you have just one supplier. Knowledge graph technology means you can have all of your suppliers represented, with all that multidimensional data value, and once you’ve built the data-tree, you can walk it in practically any direction.
Connecting nodes for more valuable understanding.
If this supply array has a product, say a hairpin electric motor, you can automatically see which other suppliers in your supply chain data have hairpin electric motors as a product, and which other customers have them as a product which they buy from a particular supplier, or set of suppliers.
What happens then is that you can construct increasingly complex queries, and you can walk the graph in any direction that you need to.
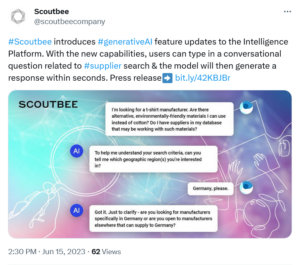
Connecting supply chain data on Twitter.
THQ:
Adding richness, connectivity and flexibility to both the way data is stored, and the potential queries that you can make of all those knowledge-strands. Slice and dice supply chain data any which way you need to, to get rich answers to queries on any element of the data you decide to query?
PH:
Exactly, yes, it really is just programmatically walking a tree then, which means there are lots of different ways of doing it. But you can also do different things, like using a query language called Cypher, which is equivalent to SQL but different in nature, so you’re not limited to querying in natural language terms.
Supply chain data compilation is like a puzzle box – knowledge graph technology can help you solve it.
Why should companies care?
THQ:
Let’s talk turkey. Is there any evidence that knowledge graphs deliver “X” more value to companies than traditional data interrogation schemes?
PH:
I did some research on this, and we can hang our hats on the fact that where clients have used knowledge graphs, they’ve typically had returns of 20% more value than they had using their previous systems.
THQ:
How are we arriving at that figure?
PH:
Through a focus on much faster development cycles. If you think about developing software in an agile way, you might not have a full set of requirements at the beginning.
The ability to dynamically build your database schema actually frees you from a lot of those rigid constraints.
Another feature of knowledge graphs that helps companies wring the value out of them is the ability to start traversing between graphs. Google has a knowledge graph, LinkedIn has a knowledge graph, and if you have a node for a company, you’re able to start connecting those nodes and effectively walk across to the LinkedIn graph to start investigating it.
Wikipedia has a knowledge graph. So if I want to see what a hairpin motor actually is, I can connect to the Wikipedia node and it will tell me.
All of this comes out of the semantic web, which was fashionable a few years ago. But it actually starts to become a way of linking pieces of knowledge together. And obviously, that’s where it becomes quite powerful as we start talking about generative AI.
Knowledge graphs and generative AI.
THQ:
Oh, by all means, let’s start talking about generative AI. What are the biggest confluence points between knowledge, maps and generative AI?
PH:
Generative AI chat interfaces allow you to very much democratize access to data. At the moment, we’re still in the early stages, so things are not much more complicated than doing a semantic search across the database.
But we’re starting to build our capabilities, so for instance, you can look across all of the suppliers in a particular category, or even all of them together, and start to gather quite interesting information together, from across your supply chain data.
Those kinds of queries would normally be very difficult to do, say in a project for a BI team. But generative AI means suddenly, this is something an everyday Joe kind of user can open up, and ask sophisticated questions.
One of the big influences that generative AI has is that it changes the relationship in terms of what the purchaser does, and what they can bring to the game. Traditionally, in software, you have a lot of people filling in forms.
It’s a lot about understanding what the process is, and having a lot of knowledge. But increasingly in supply chain management we talk about the Age of the Polycrisis – we always have to be ready for anything, and people are coming into the sector from a much wider range of backgrounds than they used to have.
Rather than training everybody to use old-fashioned tools to deal with the Polycrisis, the generative AI chat interface is very democratizing, and allows different people with different skill sets to bring in those minds and mindsets to supply chain management.
The solution to Scope 3 data.
THQ:
This has just occurred to us – we’ve been talking recently to people dealing with the oncoming storm of Scope 3 data on emissions.
That’s emissions data not from within their own company, but with the companies of their suppliers. If you wanted it to, and you could gather the data, knowledge graphs would entirely obviate the challenge of that data collection, wouldn’t it?
PH:
Yes, it’s a system that means the technological barriers to Scope 3 data collection and presentation become easy to get rid of, because it’s simply a case of adding another relationship join between one supplier and another. The only barrier that then remains is getting suppliers to give you this data. The technology is there. And we some early demos we’ve done around this, but it becomes very powerful.
In Part 2 of this article, we’ll look at the scale of potential implementation of knowledge graphs and their multiverse of data-strings.