Generative AI in social care?
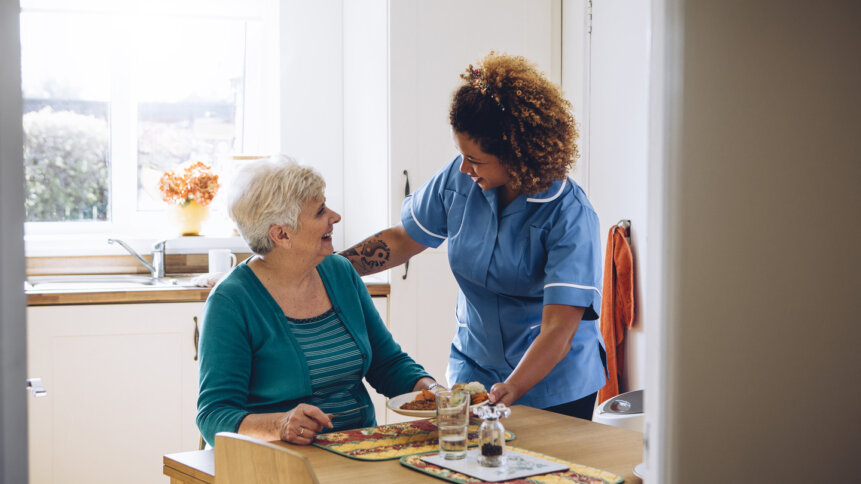
Since the explosive launch of ChatGPT from Microsoft-backed OpenAI in November 2022, the number of applications to which generative AI has been put has expanded exponentially, with critics arguing that the adoption and use-cases have gone too far, too fast, given the lack of an objective source of truth and in most cases, no public scrutiny of the training data for leading large language model versions of generative AI.
Meanwhile in the UK, the country’s system of socialized medicine (the NHS) has had a harrowing first quarter of 2023, with long waits to be treated and get the care people need, and both nurses, doctors, and paramedics striking for living wages and better conditions.
It was almost inevitable that the two worlds would collide at some point. Now they have. We sat down with Dr. Ben Maruthappu, CEO and co-founder of Cera, a healthcare provider in the UK, which claims to be the first in its field to have developed a generative AI tool to help cut down the time and the paperwork involved in onboarding patients to social care services.
READ NEXT
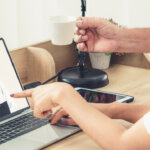
Digital connectivity in healthcare
We asked him exactly what the tool would do.
BM:
The idea behind our application of generative AI is to help transcribe conversations which have taken place with patients during their at-home appointments and convert them into care plans.
THQ:
And how does that change the world?
Tick tock, tick tock…
BM:
Well, it’s a process which usually takes staff up to 10-12 hours per patient in traditional social care provision. And it’s not just about transcription. Once the care plan information is processed using an AI-trained model, personalized tasks will be created for carers to define an outcome-based program, enabling carers to learn about the patients’ conditions. We take time out of medical and care staff’s day when they previously had to be sitting and writing up these plans and tasks and notes, and free them to do what they do best.
THQ:
You’ll have heard the same things we’ve all heard about generative AI – that it has novobjective source of truth, and that, unless you know the truth going in (which could be argued to rather defeat the point), it can often be staggeringly wrong with a highly persuasive level of plausibility. So, given that we’re dealing with patient data here, what are you using as a source of truth for generative AI at Cera?
BM:
We’re using existing care plans from within Cera to teach the generative AI what a good plan looks like. We have an extensive database of previous care plans, given that we care for tens of thousands of patients a day. That helps to ensure we have a substantial source of truth to base this tool on.
THQ:
And which version of generative AI are you using?
BM:
GPT-4.
Edge case training.
THQ:
The version that stopped its general data training in 2021 – though we appreciate in this Cera-internal training model that’s less relevant than it would otherwise be.
How much training in edge cases, for instance in transcription of conditions that could look or sound similar, have you done with it? Presumably, there’d need to be a lot more done before it could be effectively rolled out across the NHS, despite potentially being extremely helpful in the dual crisis of time and staff which currently exists across that organisation?
BM:
Pre-trained neural network language models like GPT trains data, allowing it to grasp highly complex representations of text and perform well on a variety of language tasks. The model also uses a concept called “few-shot learning,” where by being fed just a small number of examples of a specific task, it can function very well on that task without manual fine-tuning or a large corpus of specific training data.
In our case, we have thousands of data sets to train the model, and we will operationalize it using a “Human-in-the-loop” approach: the model will create a draft plan that will allow our workers to edit the output if needed, making sure it is completely accurate.
Additionally, we will enable a reinforcement learning and training loop that will optimize the model using that human feedback, at scale, in our nationwide day-to-day operations.
Ultimate destination.
THQ:
What’s the hope for Cera’s use of generative AI? What’s the dream? Why lean into it so heavily so early?
BM:
We’re leaning into this technology to better empower our staff and help alleviate pressures on the NHS, by increasing patient onboarding capacity at a time when it is needed the most.
By drastically reducing paperwork, it’ll allow office staff and carers to focus on delivering higher quality care, while making the sector more sustainable. It doesn’t have to stop at care in the home either – we believe that this could revolutionise the NHS too, to help tackle burnout in junior doctors and transform healthcare as we know it.
THQ:
The idea of releasing patients from hospital beds into social care would be fine in principle, but the UK’s social care system has been underfunded for over a decade.
The £86 bn funding package recently announced by the UK government as part of the Better Care Fund framework will be a welcome thing, to be sure, but it will take time – and consistent additional funding – for that to make a genuine difference, won’t it?
How long would you say you’re looking at before the effects of AI at Cera might be viably felt outside its current user-base?
BM:
Technology allows us to do more with less. Our use of GPT-4 to support care plans we estimate will save staff 2-3 hours per day, meaning they can focus on looking after more patients, supporting Local Authorities and the NHS to discharge patients from hospitals to home at a faster rate.
One of the reasons we established Cera was to create a more sustainable digital-first model, where technology alleviates staff pressures so they can focus more on what they do best; caring.
We’re already using smart technology to help improve patients’ wellbeing and health outcomes, which has already proven to reduce hospitalization rates by an unprecedented 52%, predict up to 80% of hospitalisations seven days in advance, reduced patient falls by ~17%, reduced urinary problems by ~47%, and infections by ~15%, and which has also helped to improve medication and prescription compliance in older patients by 35%.
AI technology is evolving rapidly, and we expect this to have a material impact on the capacity of our workforce within the next 2-4 months. Once that additional capacity is realized, we should be able to very readily onboard new patients at a much faster rate.
That means the impact should be felt outside of our current user-base within the same timeframe.
Potential downsides?
THQ:
In a healthcare system where currently, large swathes of the staff are in revolt against the government’s pay and conditions, is there a danger that, while in no way being the intention of the technology’s development, technology that’s genuinely enabling for medical staff could be used as a way of downplaying the necessary skills and dedication of health workers?
BM:
The use of generative AI technology can be intimidating but, in layman’s terms, it is converting content and understanding it in a human form, while offering smart recommendations and output. We believe with the correct use it is here to help, and not to hinder. We want to use it to maximize the time and efficiency of our staff, allowing them to focus on quality care rather than administrative work.
Almost every sector can be revolutionized by Chat GPT, depending on how it’s used. Used in the right way, it should optimize workers’ ability to spend more time on skills that cannot be replicated by technology, and reduce time spent on administrative tasks.
Nuts and bolts.
THQ:
Talk us through the process you’re using at the moment, and how generative AI will fit into the way things work?
BM:
The administrative burden of staff that work in care is substantial – it’s our aim to look at ways GPT-4 can significantly reduce this burden. One of the most time-consuming processes for our workforce is creating care plans for patients. Staff will now be able to use this innovation to help transcribe conversations which have taken place with patients during at-home appointments and convert them into care plans.
Once the care plan information is processed using the AI-trained model, managers will run a checking and sign-off procedure and edit the plans as needed. Personalized tasks will then be created for carers to define an outcome-based program.
We predict that the new technology will double the number of patients we are able to onboard each day.
Trust me?
THQ:
The addition of generative AI to healthcare, using patient data, is bound to raise both headlines and potentially controversy. Given the criticality of correctness in a medical reporting setting, what’s your level of trust in the technology?
Come to that, what do you think health trusts’ – or patients’ – level of trust needs to be to be comfortable with the addition of generative AI into their healthcare regime?
Is there, for instance, a requirement to tell patients that AI is being added to systems on which their healthcare plan might depend?
BM:
The accuracy and correctness of reporting using AI is absolutely critical, and we are training this GPT-powered model using a substantial existing dataset, which will inform the way it’s used and the output it produces.
Building the technology this way means we’re not starting from scratch, and already have a huge amount of existing material to springboard us into a highly intelligent and refined model. However, we will still run our human-led process in tandem while we’re testing the model to ensure its accuracy, as well as keeping robust checking processes in place by our management teams and operational leaders.
That gives us high confidence and trust in the technology for this particular use-case. But absolutely, it’s very important that anyone using this innovation in healthcare should do so with a healthy degree of scrutiny, significant testing and rigorous focus on data protection.
Like any new invention, there is always a period of acclimation, where society adapts to a new way of doing things. The speed and scale with which generative AI can change the world is still being fully understood.
Given its relative “newness,” especially in the world of healthcare, there will likely be an adjustment period where regulators, commissioners and providers define criteria within which generative AI can be effectively used.