Seeing the future of self-driving cars coming
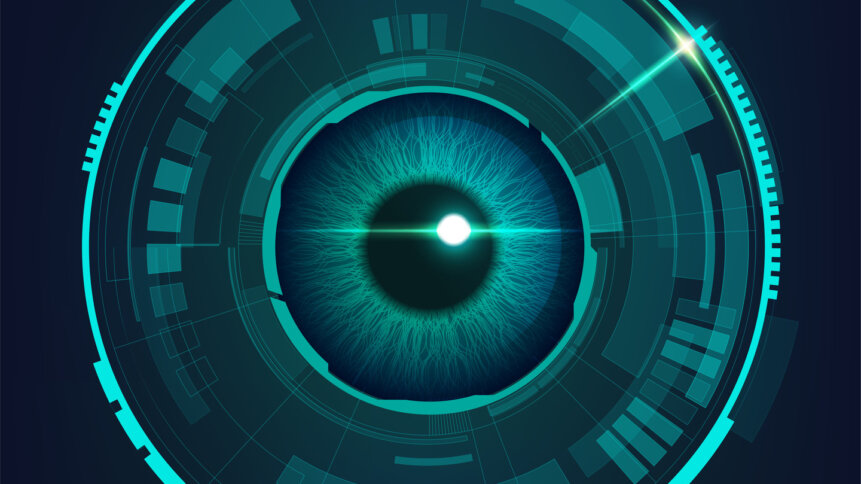
In Part 1 of this article, we spoke to Kevin Gordon of NexOptic – a company working to solve the problems of enhanced visibility and pixel-level clarity for an initiative on self-driving cars that goes by the name of ALIIS (Alice), based in South Korea.
Kevin explained how NexOptic – originally an optics company – came to be solving the problems that might well one day render self-driving cars the reality we’ve been promised is coming for a decade. We learned of the trials of getting noisy images cleaned, and the setbacks the company had had with scaling its initial process.
In particular, Kevin explained that the South Korean government had been helpful in facilitating the dataset build out for such projects, allowing younger companies at the forefront of innovation to get on board.
Argo – Nought?
THQ:
That’s quite the contrast to the way things have gone with Western developments in self-driving cars, isn’t it? For years, carmakers like Ford and Volkswagen were working – or at least funding – the Argo project together as a potential commercial offering. Big manufacturers, essentially looking to capitalize on the breakthroughs that were made, rather than governments backing entry-points for smaller, innovative companies.
And of course, in October, 2022, Argo went bust when the big manufacturers pulled the plug (though Ford is trying to resuscitate parts of it now).
Is it fair to say that whoever cracks the Level 4 automation first will essentially be the ones to revolutionize the world of transportation?
KG:
I think so. And clearly, it’s unlikely to be any of the Western carmakers who become the thought leaders on this now.
THQ:
It’s interesting to see what happens where there’s that dedication on a national level to get this done.
The Subway principle.
KG:
Oh, absolutely. The Western situation is in stark contrast with just how in tune with where things are going the South Korean government is.
There’s talk about seismic shifts in the automotive industry, and some of those shifts within the industry include things like picking your IP piecemeal, and building stacks based on the best in each area. A camera stack here, a noise reduction algorithm there, and then have it manufactured into a chip.
THQ:
The Subway sandwich approach to tech building?
KG:
Yeah. And you can be picky with the neural processing units, but your software solutions can iterate, you can swap them out, and it makes for a more generic ecosystem that’s a lot more adaptable, especially because this stuff is very fast-moving and as you say, the first movers will set the tone of how things look for everybody else. So if you’re the platform provider, spending tens of millions of dollars on a specific chip run which will be obsolete within a year or two doesn’t make sense.
Quiet, please.
THQ:
Coming back to the ALIIS application particularly. You were saying that the idea was to give self-driving cars more reaction time. How much more reaction time can ALIIS deliver?
KG:
That’s all down to how we interplay with the perception stack. The ALIIS we’ve talked about so far is a preprocessor. It goes ahead of the classifiers, detectors, and the segmentation algorithms. And here, the interplay between the perception stack and how tolerant they are to noise is key. If you’re in a dark driving environment, you have that fall-off from your headlights, right? The signal-to-noise ratio is really the dominating term on whether or not you can detect something on the periphery.
So in reducing the signal-to-noise ratio, that’s where we can legitimately talk about it, rather than how many additional feet we might see.
THQ:
We’re asking customer questions when there’s really only an engineering answer, right?
KG:
There are so many pieces in play. But if we have that preamble, where we’re reducing the signal-to-noise ratio, what we’re doing is reducing the noise by 10, 12, maybe 15 decibels.
There are two ways that helps. Firstly, when things are smaller and harder to perceive in that high noise environment, cut 15 decibels of noise out of the frame and suddenly they can be detected. And secondly, when it’s too dark to see things clearly, with the kind of noise reduction we’re getting, things can be seen.
What we’ve heard from customers is that the difference is on the threshold of where high beam highway driving is. Which translates to a lot of “reaction time” added into the system – which of course is why high beam headlights were developed in the first place.
Basically, where that light falls off is really where they’re looking to extend into. Now, it looks like there are regulations around how much light you can push out of the front of a car, so that’s a hard physical limit. That means they’re looking at software for that periphery.
Somewhere in the 10-20% range of extra visibility is doable.
THQ:
And of course, simply because there will be laws as to how much actual illumination you can put out, if you can increase the translation of the illumination that you have, and therefore get yourself or your system better results, that’s going to be good thing.
The ultimate label-maker.
KG:
Yeah, absolutely. And there’s another way that this can contribute as well.
We know, specifically on the Korean government project, there is something quite nice for us. They have all this training data, and perhaps it’s noisy. You could, in theory, use our ALIIS algorithm to process all the noisy images and have nice, clean images for training. Of course, that makes them dependent on this algorithm for runtime as well. I mean, we’d love it, but that doesn’t fly. So we’ve looked at another alternative there as well, which is the idea that this data all has to be labelled.
Who labels it?
I mean, it might be computer-assisted, but at the end of the day, there’s going to be a human in the loop. And, you know, humans are going to suffer from the same perception challenges in the presence of noise. So what we’re doing right now is cleaning up the training data for the human operator. Apply the label, and then you can train the algorithm on the labels with the noisy data. So now it learns the ALIIS function as part of the object detection regime. There are a lot of different ways to mix and match this, which is cool.
Gasoline and water.
THQ:
There are a lot of challenges to get all this done, no? And as with many projects, you can get some of them done reasonably easily, reasonably fast, but getting it all done at the same time and reducing the file size so it’s useable in real time feels like the quantum leap.
KG:
That’s something we struggled with early on. With noise reduction, it’s deceptively simple. Take away the graininess in the photo. However, there are big implications. If you’re removing the noise, it’s more compressible, and noise… I like to make the analogy that noise is like water and gasoline. Noise is not good for a compression engine. But if you remove it, suddenly, your image is more compressible.
With noise, it’s affecting object detection algorithms, it’s affecting the quality of photos and how you can edit them. And so it becomes this element of physics coming into the system that is limiting all these other processes.
Automotive is well-known as a slow-moving industry, and even if it’s fast-moving, it’s still slow to realize revenue as you’re meeting up with these long-term development processes and going to market.
The triple hit and the double cone.
So, we focus on the consumer side, which is heavy on “quality according to human perception.” And then on the side, we’re looking at quality in terms of machine perception. And on the backburner, there was always the kind of file size reduction element which we found out about by happy accident.
So if we’re going for consumers, and it’s more about the visual perception, we have a series of metrics that we can track for that, and it’s really easy.
Similarly, if it’s on the autonomous driving or safety applications, we have another set of measures, and again, we can steer the process appropriately. In that case, we’re looking at things like, as you say, making it do all of this and run in real time, which is a trickier challenge.
THQ:
Especially at automotive speeds. And even more especially at the speeds of which optimized self-driving cars would be capable, given the legal ability.
KG
And brutal as well, because this type of algorithm, pixel-to-pixel networks, have some of the heaviest requirements in terms of processing power, just because with some algorithms you can get away with downsampling the image before it goes in… but we can’t do that, because it needs to be high-resolution coming out.
A lot of algorithms, because they go from an image on the input to maybe 100 labels at the end, the amount of compute required is very favorable.
They have a cone-shaped compute, where it’s maybe heavy in the first bit, but by the end that compute is quite lightweight. Whereas for us, it’s big on the input, big on the output, which means it has heavy compute requirements.
THQ:
You have a double cone – broadening back out to deliver high-resolution imagery at the output.
KG:
Exactly, yes.
We took a popular neural network used for medical applications, which has become a de facto standard under the hood. But it isn’t going to cut it for real time applications with higher pixel count cameras, so we heavily modified it, both to reduce the compute and use that compute more effectively. And, because we want to put this at the front of the entire stack (the selling point of a preprocessor is you don’t change anything else, you just pretend you have either more light in the scene or a better camera up front), it has to be transparent on its latency.
The 4K budget balance.
The amount of workload it’ll do will still depend on the resolution of the camera you attach it to and the chip that you’re using – obviously, more horsepower in your chip means we can run the preprocessor faster.
But to give you an idea of the kind of throughput that we can realize with this pixel-to-pixel algorithm, there’s a chip that was released last year that’s easily delivering 4K at 30 FPS (frames per second) with this algorithm in real time, so there’s very little latency. We can handle these higher resolution workloads. Automotive companies are not seeing 4K right now, they tend to be lower resolution, which does the job just fine.
THQ:
So while you can refine this as far as it can possibly go, the version that makes it to any kind of market is likely to be a balance of what’s good enough to get the job done and cheap enough to sell?
KG:
Yes, absolutely. And it’s also worth remembering that compute budgets on these platforms are going to be allocated across a whole bunch of different functions. It doesn’t make sense for us to say “We can run your real time image preprocessing, but we’re going to take 90% of your compute while we’re at it.”
That is one thing that we’ve built into our systems – you can pretty much specify our compute budget, and then we can optimize the best solution for that compute budget.
THQ:
The future of self-driving cars is coming at us through the dark. While Western carmakers may be stepping back a little, at least with ALIIS, we can still see that future coming.