Self-driving skillset – game theory for autonomous vehicles
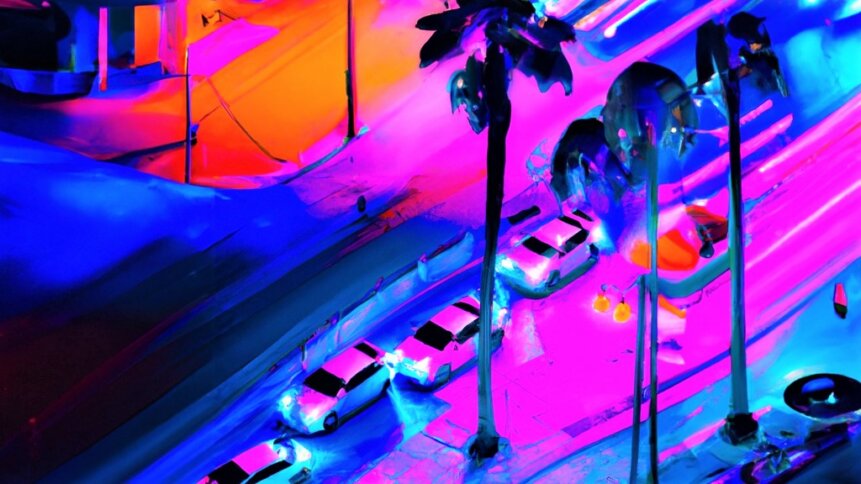
Getting your Trinity Audio player ready...
|
Artificial intelligence (AI) techniques such as deep learning play a key role in enabling self-driving vehicles – for example, helping with feature extraction and object classification. AI can turn a fusion of camera, LiDAR, and automotive radar data into meaningful navigation information. But there are other tools that can help the decision-making process, such as game theory for autonomous vehicles.
Game theory may be in the shadow of recent breakthroughs in AI, but its automotive future could turn out to be a very bright one indeed. Groups around the world have been busy looking at game theory for autonomous vehicles, and the list of potential applications is a long one. But before we hit the highway, let’s first buckle-up and take a quick tour of game theory itself.
If you’ve seen the film ‘A Beautiful Mind’ — directed by Ron Howard and starring Russell Crowe — then you may already have a head start, of sorts. The biopic centers on the life of John Forbes Nash, an American mathematician awarded the 1994 Nobel Prize in Economics Sciences (jointly with John Harsanyi and Reinhard Selten). Nash’s portion was awarded for his work in game theory, specifically the Nash equilibrium theory.
What is game theory?
Game theory, which applies to strategic interactions, sheds light on how two or more decision-makers will behave in a competitive environment. It can be applied to scenarios of various kinds, not just games. But a key point is that the environment is interactive. In other words, the utility and well-being of one party affects the utility and well-being of the other parties too.
Nash’s contribution to game theory points to an equilibrium state, which can be used as a basis for resolving strategic interactions so that no entity loses out. Individually, players may benefit from pursuing a different approach, but at the expense of other players, which starts to hint at how game theory can be applied on the road. It may also flag why self-driving cars can sometimes appear hesitant in traffic. But that response may have more to do with aggressive human driving styles rather than a failing in game theory for autonomous vehicles.
Configuring traffic scenarios as a game featuring traffic lights, queuing, and the presence of heavy goods vehicles (which may behave differently to regular automobiles) — to list just a few elements — has the potential to provide ‘an efficient and applicable decision making model’, as recent analysis shows. Researchers at Stanford have developed a fast solver that considers an autonomous driving vehicle and the cars in its surroundings as agents participating in a game. Executing its planning algorithm at frequencies above 70 Hz, the team finds that the control scheme is capable of complex driving behaviour so that ‘vehicles negotiate and share responsibility for avoiding collision’.
Arriving at a state of affairs where no entities loose out (in other words, crash!) shows how Nash equilibrium conditions can help self-driving vehicles to navigate safely. But game theory does carry certain assumptions, which includes that all players are rational. And, naturally, this can present issues in a world where people are sometimes contrary and don’t always make the best decisions. But sometimes those choices are more rational than they may first appear.
Experts at the Institute for Transport Studies (ITS) in Leeds, UK, cite findings from the European CityMobil2 project to highlight a quirk of autonomous vehicles maximized for safety that became apparent during the trial. The project featured self-driving minibusses that were deployed in several European cities, including La Rochelle, France, and Trikala in Greece. And, because the behaviour was easy for pedestrians to predict, people started to step out in front of the minibuses as they became more used to the vehicles, bringing the self-driving machines to a stop.
VR to the rescue
Thanks to virtual reality (VR) systems, algorithms can be fine-tuned safely without putting human participants at risk. To further develop game theory for autonomous vehicles, the ITS group simulated a range of pedestrian crossing environments and different self-driving vehicle types.
More recently, engineers from UC Santa Cruz have solved a 60-year-old game theory dilemma, which could open up a subset of game theory relating to differential games (where players are in motion) to self-driving car developers. Elsewhere in the US, engineers and lawyers have teamed up to use game theory to determine who is at fault in self-driving car accidents. And, for scenarios where multiple parties are to blame, how to split the accident loss among them.
As with the minibus study, there are some familiar patterns that creep in as people share the road with machines. “We know that human drivers will take more risks and develop moral hazard if they think their road environment has become safer,” notes Sharon Di, a researcher from Columbia University’s School of Engineering, who teamed up with legal colleagues in the study. “It’s clear that an optimal liability rule design is crucial to improve social welfare and road safety with advanced transportation technologies.”
Game theory for autonomous vehicles is a work in progress, but it’s a topic that’s certainly worth keeping an eye on alongside other breakthroughs in automotive AI.