Re-identification risks: can data ever be fully anonymized?
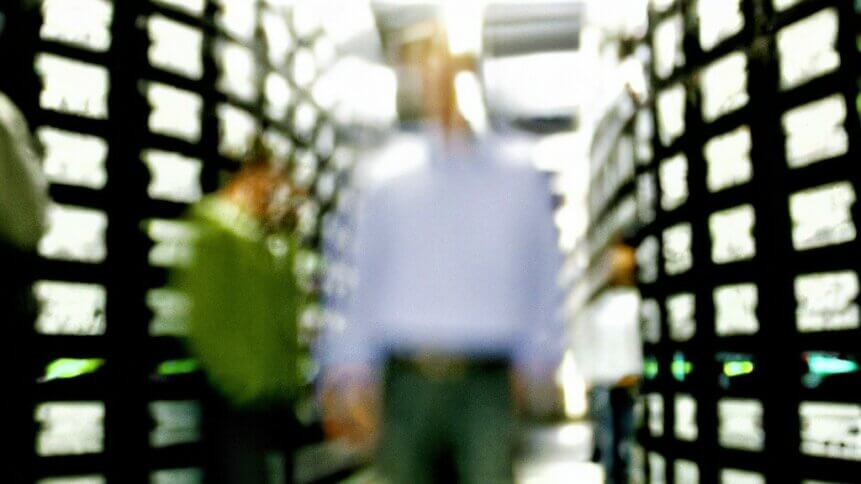
Getting your Trinity Audio player ready...
|
Picture a seesaw. On the one side you have big data. Output from smart gadgets, wearables, IoT devices, and other equipment connected to the internet is adding to a wealth of digital information. Buried within these details could be ground-breaking discoveries in healthcare, clues to new medicines, and other revolutionary gains for humankind. And to deny data scientists and other analytical experts access to this stockpile of potential wisdom feels wrong. But tempering this enthusiasm for big data – and sitting on the other side of our metaphorical seesaw – are requirements to protect people’s right to privacy and minimize the risks of re-identification.
Data anonymization is ripe with examples of privacy blunders. And research suggests that it may be virtually impossible to guarantee that somebody, somewhere, won’t be able to piece together details sufficient to re-identify anonymized individuals. The risks of re-identification are real and grow as the amount of background data on individuals accumulates on the web and in other publicly accessible locations.
There are many legitimate reasons for releasing anonymized information into the wild. For example, funding bodies may mandate that scientific data is free to access, and made available for secondary research purposes. Tools can help data owners determine re-identification risks. Algorithms digest the various information fields and output a score that correlates with the likelihood of being able to put names to anonymized ids.
As Google’s Cloud Data Loss Prevention team points out, bucketing identifying features such as ‘age’ and ‘job title’ into ranges, rather than including the exact figures, can help to blur the view for adversaries. Google offers a Data Loss Prevention API that, according to its creators, can intelligently detect sensitive information and use de-identification methods to ‘mask, delete, tokenize, or otherwise obscure’ the data.
Tricks include shifting dates by a random amount of time, but methods need to be chosen carefully not to obscure any valuable patterns. Higher anonymity values may boost privacy, or at least appear to. But they may carry a penalty in terms of data utility. A bigger concern, however, is whether it’s possible to truly anonymize data in the first place.
Copula functions highlight re-identification risks
In 2019, researchers – using statistical tools dubbed copula functions, which measure the dependence of one variable on another – concluded that 99.98% of Americans would be correctly re-identified in any dataset using 15 demographic attributes. And 15 attributes isn’t even scratching the surface. Modern datasets contain many more points per individual. In their paper, published in the journal Nature Communications, the data scientists reference a de-identified dataset containing 248 attributes per household for 120 million Americans, to highlight the depth of information that’s available commercially.
Copula functions crop up again in related work. And when you learn more about how they work, you can see why. For example, imagine you have data on people’s heights and weights – copula functions might show that taller people tend to be heavier than shorter people. And if the joint distribution of somebody’s height and weight is unique, recreating that joint distribution carries potential data re-identification risks.
What’s more, you might think that as the size of a dataset goes up, there would be more chance to hide and greater overlap between attribute distributions. But experts have shown that re-identification risks remain high even in country-scale location datasets. It turns out that re-identification risks fall slowly with increasing dataset size. The study estimates that it’s theoretically possible to identify 93% of people in a dataset containing information on 60 million individuals, using as few as four pieces of auxiliary information.
The work, carried out by members of the Department of Computing at Imperial College London, UK, focused on location data. And the research flags the potential for geo-tagged tweets, online check-in details, or even simply observing peoples’ behavior and their whereabouts, to de-anonymize data. Location data – as various unintended examples have shown – can reveal much about us. The information can help to predict our incomes, identify where we live, work, when we sleep and wake up, hint at our age and gender, and reveal who our friends are, as well as places we like to visit.
Mobility fingerprint
Human mobility turns out to be a highly effective fingerprint on our behavior and brings with it the risk of data re-identification. Screening algorithms, as touched on earlier in this piece, offer some reassurance on the effectiveness of anonymization methods. But, typically, they are only looking at the data distributions and watching for any unique cases. They tend not to take ‘adversarial’ actions into account, such as the possession of auxiliary information, which – as the Imperial College London team has indicated – can quickly bring someone’s identity into sharp focus.
The balance between using data for good and protecting individuals’ right to privacy remains difficult to get right. And analysts even need to carry out some ‘crystal ball gazing’ to comply with regulations. Europe’s GDPR defines anonymous data as information that doesn’t identify individuals based on ‘available technology at the time of processing’. But compliance also requires factoring in future ‘technological developments’, which feels like a stretch given how difficult it can be to anticipate progress in potentially game-changing fields such as artificial intelligence (AI).