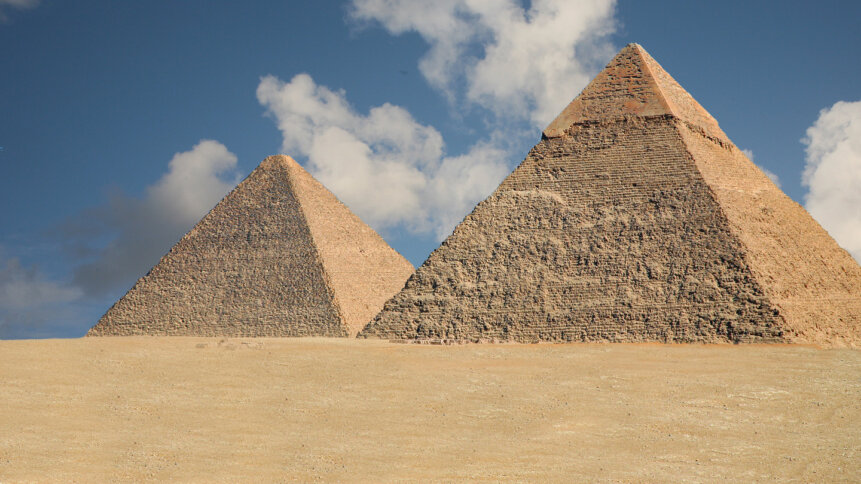
Getting your Trinity Audio player ready...
|
In Part 1 of this article, we spoke to Krishna Subramanian, co-founder of Komprise, a company that manages unstructured data projects, about the importance of taking care of unstructured data at the first available opportunity. She mentioned that enterprise customers who are currently drowning in unstructured data could save between 70-80% of their unstructured data infrastructure costs by getting that data organized, mobilized, and correctly stored.
While we had her in the chair, we asked Krishna exactly how unstructured data management worked, so that enterprises would know what they were getting into – especially if they decided to take the plunge in 2023.
THQ:
So as we understand it, getting unstructured data under control is a financial necessity in terms of complying with some ordinances, but it also makes sense to minimize your data storage costs long-term. And also of course, by organizing unstructured data, you can begin to mine it for potentially profitable returns, where previously it was simply an economic liability.
What are the opportunities that companies open up by being able to see all their unstructured data and mine it all? What sort of things are they able to do with it?
The exciting part.
KS:
In Part 1, I called that the exciting part. As you say, there’s the legal side, and the cost-saving side, and that’s all great and useful, but that’s just kind of table stakes. What companies are able to actually do with the data, that’s the exciting part, because it varies so much from business to business, and there are opportunities that companies won’t have thought of before.
READ NEXT
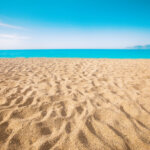
The challenges of unstructured data
You need to be able to know what the data is before you know what you might be able to do with it, and before they start to get a handle on their unstructured data, they don’t have that information.
Once you have your unstructured data with at least a structured framework around it, that’s when you can start to think about smart data workflows. Because if you have a systematic way to understand the data, and create some kind of virtual structure around it, that’s when lightbulbs start going off above your head.
The driving eye.
I’ll give you an example. In self-driving cars, the cars take lots of pictures as they are driving. So you might have hundreds of pictures of the same stop sign from different cars, or pictures of all the bicycles on the road. And a lot of that is actually not relevant. If the car makes a mistake, you want to know what it looked at in that moment, but if it didn’t make a mistake, and the algorithm worked fine, you don’t have to bring that data in and keep it forever, because you know it’s the same stop sign. And you know it’s what the car recorded.
So there’s some culling you should do at the edge before you bring that data to a data center, because it’s too much data, and you can’t keep all of it around. With a smart data workflow, that would analyze all the data at the edge, index the data, and then you could pick it up, you could run some pre-processing and say, “Okay, I know interesting events happened at these times, and I only want to keep the images correlated to those timeframes. Don’t bring anything else to the data center.”
Create a workflow like that and the software could then do that for you, and then move just the right data into a data center or a cloud for further processing. So maybe then you want to run some AI algorithm on the rest of the data, or you want to do some data mining, because now you have historicals, maybe from all the times a particular model of car crashed, or didn’t stop correctly at the stop sign, or braked too hard. You want to take all those datasets, put them together, and run something on them to maybe see if there are external reasons why these things happen at this stop light in this model of car, or whether there’s something in the coding of the car that needs tweaking.
The unstructured difference.
All these things are quite easy for us to do with structured data, by the way. If I gave you this problem in a retail environment, you would know how to do this, you would know that you need a database, you would know that you need a data warehouse, you would know that you would need an ETL tool.
There’s all this technology for structured data. But when it comes to unstructured data, there’s none of this technology, there is nothing like a database of unstructured data, there is nothing like an index of unstructured data, there is nothing like systematic pre-processing for unstructured data, so all this is getting built right now. Data lakes are starting to address unstructured data. They’ve been very focused on semi-structured data, and then expanding into unstructured data.
So data analytics is growing with unstructured data. And in different industries, processing of the content of unstructured data is growing too, like finding personally identifiable information in files or detecting things inside a video image. All of those are good, but that systematic way to index data, call data, create a workflow, extract data into an environment, and then summarize the results into tags? That’s what data management does. And that piece is missing for unstructured data. So smart data workflows of unstructured data is where we see a lot of opportunity. Does that make sense?
THQ:
Yes, it’s like unstructured data – the final frontier. Every time a lightbulb goes off above someone’s head, it will either begin the process of taking something we can already do with unstructured data and customizing it to their specific need, so that now a tool exists to do that, or it’ll identify something that we can’t already do with unstructured data, but that smart data workflows can probably help us turn into a reality. Like building the science of managing unstructured data at the frontier of commercial need.
The next 12 months.
So what do we think are the prospects for unstructured data management uptake in 2023?
KS:
You know, I don’t think it’s an overnight thing. I don’t think one year from now, everybody will be doing unstructured data management. But what will happen, and what is already happening, is that the industry will innovate on this problem.
So as I mentioned already, vendors like databricks, and Snowflake, and all the data warehousing and data lake companies are starting to provide analytics of unstructured data. And that will continue across 2023 — they will add more capabilities to analyze unstructured data. Companies like ours are providing a way to index unstructured data and create those smart data workflows of unstructured data.
Indexing the unstructured.
We’re continuing to innovate on that front to make it easier and easier. And our customers are starting to involve their departmental users. That’s a very big thing, because so far, managing unstructured data has mostly been done by IT teams. And IT don’t really know what’s in the data. They’re basically just storing it and protecting it. So they’re looking at the infrastructure of the data. But by involving the departmental users, they can tell it “Hey, this data is useful to me, this is what I want to do with it.” By creating that kind of collaboration with users, they’re taking a very important and necessary step for this whole thing to evolve to the next stage. That’s what I think we’ll see more of this year.
THQ:
While we’re on it, how do you go about indexing unstructured data?
KS:
Unstructured data doesn’t have a common structure. But it does have something called metadata. So every time you take a picture on your phone, there’s certain information that the phone captures, like the time of day, the location where the picture was taken, and if you tag it as a favorite, it’ll have that metadata tag on it too. It might know who’s in the photo, there are certain metadata that are kept.
All filing systems store some metadata about the data. A product like ours has a distributed way to search across all the different environments where you’ve stored data, and create a global index of all that metadata around the data. And that in itself is a difficult problem, because again, unstructured data is so huge. A petabyte of data might be a few billion files, and a lot of these customers are dealing with tens to hundreds of petabytes.
So you need a system that can create an efficient index of hundreds of billions of files that could be distributed in different places. You can’t use a database, you have to have a distributed index, and that’s the technology we use under the hood, but we optimize it for this use case. So you create a global index.
From difficulty to rocket fuel.
But it’s not enough just to have that. You have to keep enriching that index, because the metadata is very basic, it might tell you the name of the file, and when it was created, and who created it, and who’s using it, and that kind of thing. But it won’t tell you things like “This is a picture of a dog,” or “This is a genome.” It’s not going to have that kind of information. So you need to run the processing to get that kind of information. And then you need a way to tag and enrich the data, and then keep that tag consistent as you move the data around. That’s what a global file index does. It creates a “database,” if you will, a distributed database of all the unstructured data, and that database continually gets enriched with more information.
THQ:
And are we optimistic about businesses taking on this challenge in the next 12 months?
KS:
Very optimistic, yes, because we are seeing our customer base doubling every year. It will be led by certain sectors first – life science has embraced this technology, and so has big pharma, for instance. But the more companies take to it, the closer it will get to ubiquity, to a way of tackling a problem that’s affecting more and more enterprise-level businesses.
THQ:
Taking a big problem and turning it into potential rocket fuel in terms of putting the data to use?
KS:
I love that definition. That’s right, yes – turning a problem into an opportunity through advanced analytics.