Tube tech: payments, smartphones, and big data
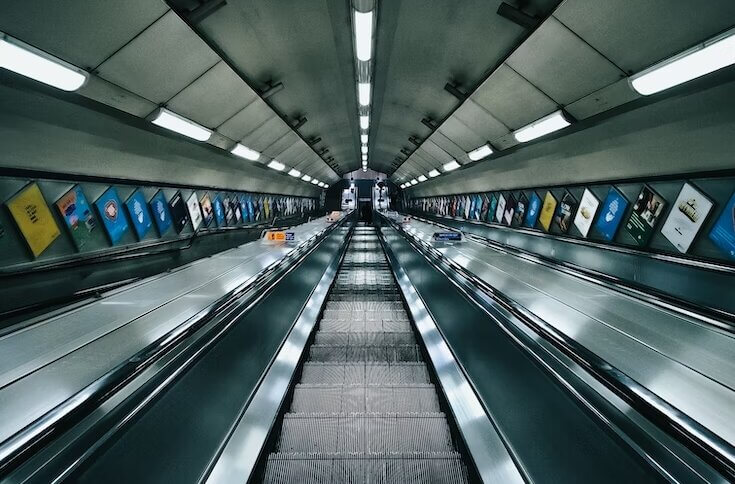
Long-established payment cards such as London’s Oyster card, New York’s Metro card, and other examples from around the world, represented a paradigm shift in transportation payments. The paperless tap on, tap off technology puts convenience in the hands of travelers and rewards operators too. Osyter cards enable 15 more passengers to pass through a London Underground ticket barrier per minute compared with paper tickets. But the big win is data. Information gathered from so-called Automated Fare Collection (AFC) or Electronic Fare Payment (EFP) systems allows operators to study passenger behavior much more easily, and in greater detail. Researchers benefit too.
Transport for London (TfL) has a variety of open data that it makes available for developers. And public transport journey information prepared for research use can be found on the London Datastore. Oyster card datasets released by TfL in 2012 and 2015 have been transformed into some amazing visualizations, such as the Tube Heartbeat, by talented data scientists. And there’s even a Microsoft PowerBI dashboard that shows you right now the number of Oyster card taps by day and station type.
AP insight
TfL has been using depersonalized ticketing data since 2005 to look at journey patterns. The information provides details on gate-to-gate trips, but doesn’t show which routes are being used by customers to get there. To shine a light on this, the transport operator ran a pilot study back in 2016, which showed how Wi-Fi connection data could be used to fill in some of the gaps. Conducted according to guidance from the Information Commissioner’s Office (ICO) – the UK’s data protection authority – the four week trial was designed to ‘give TfL a more accurate understanding of how people move through stations, interchange between services and how crowding develops’.
Given the nature of the subway network with some tunnels almost 60 metres underground, using cell phone signals to cluster traveller movements wasn’t going to work. But beginning in 2012, when London hosted the Olympic Games, TfL commissioned the installation of public Wi-Fi access points across its network. And by the start of the data gathering pilot, TfL had 1070 Wi-Fi access points up and running, which covered 97% of its stations.
When mobile devices with Wi-Fi functionality enabled come within range of an access point, the probing signals sent by smartphones (or other Wi-Fi enabled devices) are logged. These requests to connect include Media Access Control (MAC) addresses, which for the study were encrypted and depersonalized. Such a process is termed ‘pseudonymisation’. And it’s a method of distinguishing individuals in a dataset by using a unique identifier that does not reveal their ‘real world’ identity – a technique that’s in accordance with the ICO’s Anonymisation Code of Practice.
From the 54 London Underground stations included in the pilot, the TfL team collected 509 million probing requests, which allowed data analysts to construct 42 million journeys that could be broken down into a variety of ‘movement types’. These included entry or exit to and from Tube stations, pass throughs where travelers were judged to be onboard a train, interchange – in other words, moving from one Tube line to another, as well as subcategories such as passengers alighting a carriage.
Crowdsourcing
Crunching the data showed that Wi-Fi requests could provide an accurate picture of crowding on the London Underground network. This not only helps operators to make plans and manage transportation more efficiently, but also allows customers to identify quieter routes and traveling times, improving the quality of their journeys. For example, the information could be used to show whether services have seats free, have some standing room, are busy, crowded, very crowded, or full. Passengers can also be provided with estimates of how long it will take to change from one line to another to complete their journeys. At Euston station, the study showed that 32% of passengers could save two minutes on their journey by taking a shorter route. And it also pointed to other features such as providing route recommendations if customers are traveling with luggage, to give another example of the insight that can be gathered.
READ NEXT
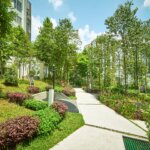
Smart tech for the 15-minute city
A major benefit of gathering journey data for transport operators is to help them optimize upgrades to the network, identifying where finite budgets could be best spent, and providing evidence to support the investment. Aggregate footfall information also supports other revenue-raising activities such as setting rents for retail clients and identifying advertising locations.
The London Underground pilot was not just an exercise in data analytics, it gave the team the opportunity to test out IT hardware and determine what would be required for a full rollout. In the 2016 pilot, it took around 20 to 40 minutes (on average) to collect the data on a secure service. And the longest lag was reported as 102 minutes. But with the lessons learned the group was confident that it could acquire data in real-time, which would make the system much more responsive.
Realtime data
A real-time system provides a valuable planning tool in the event of station closures and unforeseen incidents. And, today (since 2019 on the London Underground, and since 2022 for some stations on the newly built Elizabeth line) the system is running full time. Signs make clear that depersonalized Wi-Fi data collection is taking place and travellers can easily opt out by turning off Wi-Fi on their devices.
And if you’re thinking that metro services are unlikely to be the only locations using Wi-Fi to gather customer insights, you’d be right. Shops, restaurants, and other locations can benefit from the use of anonymized data. And to make clear to customers what data is being used and why, location analytics firms proposed a code of conduct together with The Future of Privacy Forum.
Privacy, of course, is a sensitive issue, and smartphone operating systems such as Apple’s iOS and Google’s Android platform feature MAC address randomization options. In principle, this makes it harder for third-parties to gather analytics based on probing requests made customer devices. But, as the TfL study showed back in 2016, together with the live information provided today on the network, data gathered in the right way can pave the way for better transportation and more comfortable journeys.