Number crunching AI’s carbon costs
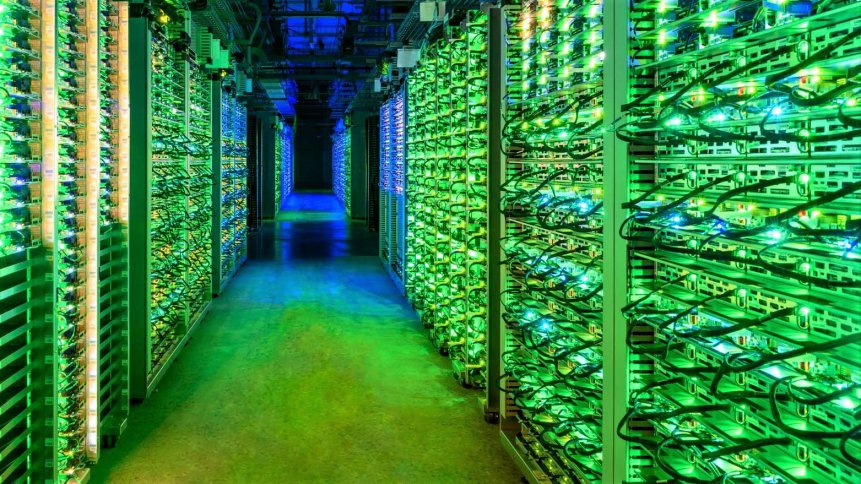
If you are in search of fascinating topics, then artificial intelligence (AI) will not disappoint. AI encompasses a powerful set of mind-bending statistical algorithms that can play chess, decipher scribbled handwriting, understand speech, classify satellite images, and much more besides. And the availability of giant data sets for training machine learning models has been one of the key elements in AI’s successes. But all of that computational work doesn’t come for free. Some AI experts have grown concerned about the environmental impact associated with building new algorithms, and the debate is triggering new thoughts on how to make machines learn more efficiently to reduce AI’s carbon footprint.
Back to earth
To dig into the details, we first need to consider the thousands of data centers (dotted across the world) that whirr away 24/7 processing our computational requests. For AI researchers, these tasks include training multi-layered algorithms with billions of data elements (or tokens – bits of words that amount to four characters in English or around 3/4 of a word). And the computational work that’s involved is astonishing. AI infrastructure company Lambda has served up some interesting facts on GPT-3 (OpenAI’s powerful natural language model for generating human-like text). According to Lambda’s analysis, training GPT-3’s 175 billion parameter model would take 665 years should you wish to do so on a single NVIDIA RTX 8000, which is no slouch when it comes to graphics cards.
In simple terms, the bigger the model, the better the performance, and Lambda’s team notes that the size of state-of-the-art language models is increasing by a factor of 10 every year, which takes us back to concerns of AI’s growing carbon footprint. Returning to data centers, it’s possible to add some more numbers to the discussion, at least at a high level. According to International Energy Agency (IEA) estimates, the consumption of electricity by data centers around the world amounts to a total figure of somewhere between 200 and 250 TWh. To make this figure easier to visualize, 225 TWh is enough energy for a high-speed electric train to travel 9 million kilometers – assuming that the locomotives and rolling stock consume on average 2.5 KWh per km of travel. And while only a portion of this power (at the data center) will be used for training and running AI models, sources suggest that the compute demand for machine learning and training is outpacing the average rise in data center activity.
At this point, it’s fair to acknowledge that data centers have done a great job of managing their energy demands – environmental concerns are one motivator, but it’s appropriate to mention that electricity represents a significant operating expense, and is ‘mission critical’ to each facility. While global internet traffic has surged, by 40% in 2020 alone, data center energy use has remained relatively constant for the past decade. “Strong growth in demand for data center services continues to be mostly offset by ongoing efficiency improvements for servers, storage devices, network switches, and data center infrastructure, as well as the high and growing share of services met by highly efficient cloud and hyperscale data centers,” writes the IEA.
Photons and more
Also, vertically integrated data center operators such as Amazon, Google, Facebook, and others, will be quick to add that their facilities are powered by renewables. Naturally, this reduces the burden that data processing places on the environment as the electricity powering the racks of computing hardware and necessary auxiliary services such as heating, cooling, and lighting can be sourced from the sun and the wind, for example. However, as the FT has unpicked, even though data center energy agreements may offset 100% of their power consumption through renewables, facilities may still be consuming fossil fuels during times when wind- and solar-power is unavailable. There are also the embedded carbon emissions of the computing equipment itself to consider, which arise as the manufacturing methods and component materials sourcing activities generate carbon emissions too – a point that Microsoft acknowledges.
Earlier this year, Microsoft discussed the topic of efficient model training in a recent blog post. Developers are busy exploring ways to shrink AI’s carbon footprint – or at least, restrain its growth. Steps here include finding ways to reduce the compute and memory demands during model fine-tuning, with proposals suggesting that a reduction in GPU use by a factor of three is achievable during this stage of the process. Model compression is showing promise too, where sub-layers of the AI data are pruned into a sparser, but still representative version of the previously assembled conditions. Here, studies have shown that compressed models could require around 40% less training time while achieving similar algorithmic efficacy.
Developers can also benefit from monitoring tools that will pinpoint gains achieved through optimized code or data hosting arrangements. “CodeCarbon is a lightweight software package that seamlessly integrates into your Python codebase,” write the inventors who have made their tool freely available. “It estimates the amount of carbon dioxide (CO2) produced by the cloud or personal computing resources used to execute the code.”
Full circle
Coming full circle, it may be possible in the future to deploy more energy-efficient AI to help guide more efficient data center operation to lower – you’ve guessed it – AI’s carbon footprint. Today, there’s already software available, provided by Cirrus Nexus, which data center operators can use to assign a cost to carbon and propagate this through an AI algorithm. Rather than just showing CO2 calculations, the results provide insight into ways that users can configure their facilities to maximize the environmental gains available.
Making visible the carbon footprints of the algorithms powering today’s technology helps on multiple fronts. It’s seeding discussion on the most efficient way to train future AI, holds the IT sector and its customers more accountable for the environmental cost of computing. And lastly, it could be good for business. Tellingly, Amazon released a customer carbon footprint tool earlier this year, and other big names, such as Google, also allow clients to export information on cloud carbon emissions – a service currently available in preview.