Big Data: Making the Most of Data Science
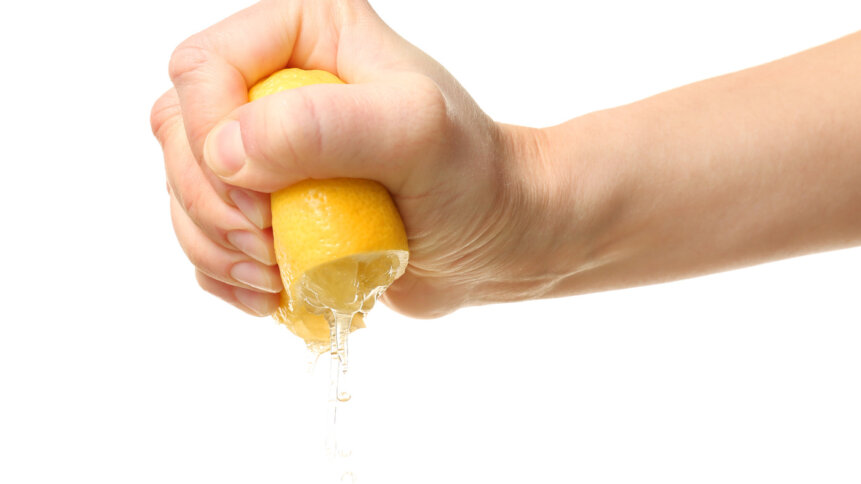
As the drama and paradigm-shifting presentations at Big Data London wound to a close on the second day of the conference, organizers got some of the big names in British data science together for a feature panel on how to make the most of data science in business decisions.
Chaired by Nicholas Deveney, Director of Consulting and Data (Eden Smith), the panel included Richard Pugh, Chief Data Scientist (Ascent), Michael Terrell, Head of Data Science (Channel 4), Shorful Islam, CEO (Be Data Solutions), and Megan Stamper, Head of Data Science (BBC Product Group).
So – how do companies make the most of data science to guide their day-to-day and strategic operations?
Solve the Right Problem
Richard Pugh had definite ideas. “You have to make sure your data scientists engage with the actual business problem. But also, business stakeholders have to engage the data promise, too. Business stakeholders find it very difficult to engage with the process. And in fairness, we in the data science community probably haven’t demonstrated consistent value, because we end up relentlessly and brilliantly solving the wrong question. So, to get the best out of your data science, nail the right question. The success of data science depends as much on people as it does on science.”
Nicholas Deveney added “Our ability to deliver relevant, actionable insights is key, too. If the task was to sell clothes, we could bring you insights like ‘On a Wednesday, people called John buy more trousers.’ But what the hell do you do with that insight? It’s not targeted insight, it’s just a factual output based on crunching the data. So, getting the right questions are key for the business stakeholders to get the most out of their data scientists, but our delivering the right, actionable results is key to ensuring they trust us to do it again, time after time.”
When Is Data Science Not Data Science?
Deveney then threw a new question out to the panel. “How complex does the solution need to be to be described as data science?”
Michael Terrell pondered the levels on which the question was applicable. “When the business has broad problems, you can’t code for them, so the problem gets broken up. But when the problem gets broken up, there are lots of answers to the various parts of the question, but CEOs don’t see the answers to their broad, initial questions.”
Megan Stamper added to that. “The nature of R&D accepts failure over a long time – and it sees the value of that. If you find out a way not to answer a question, it has value because it takes you closer to the right answer.”
That’s a pure, scientific logic that has come down to common understanding through Thomas Edison. Asked by a journalist in the 1920s how it felt to fail 1000 times in his attempt to make an incandescent lightbulb, Edison spun the question. “I did not fail 1000 times to make an incandescent lightbulb,” he remarked. “The lightbulb was an invention with 1000 steps.”
“But,” continued Stamper, “many businesses in the modern world won’t accept that. The idea of spending money to ‘fail’ is not commercially acceptable.”
The Importance of Play
Deveny took up that point. “How important is the ‘playground’ approach to successful data science?”
Stamper responded. “At the BBC, we have a large R&D team, so we have the freedom to spend time to innovate – but that’s not enough to reach our audiences. Built-in portions of time need to be fundamental to data science.
Terrell agreed. “If you’re building a model with these tools, and then you ask how it was made, it gives you a lot of insights.”
Moving onto questions of machine learning, the panel agreed that while some external commentators might see it as the beginning of the end of human data scientists, in reality, what auto-machine learning brought to the party was the opportunity to let data scientists think more, freed from the burden of all the tedious coding tasks, for which machine learning was the perfect tool.
The Rise of the Machines?
Richard Pugh came back with a key point. “There’s a real skill that’s missing in education – abstraction of problem. The data scientist is still the creative role in this process. If you’re only using auto-machine-learning, how are you checking your biases.
Stamper took the audience back to the start. “One of the things we can do it keep focused on the right problem. Automating all of that? Seems unlikely – certainly, not yet.”
Pugh added that to get the most out of data science, you needed a diverse team of data scientists. “Otherwise, you only have a homogenized experience to bring to the problem,” he explained. “When problems come up, how on earth are you looking at that. First, figure out what the business needs – and hire data scientists to match it.”
“Teach your data scientists to do a bit of marketing,” added Stamper. “When people engage with a task, it should be because they believe it adds value – so you have to find the value you’re bringing by answering whichever question you’re working on.”
Shorful Islam assented, bringing the panel back to fundamentals. “Sometimes, data scientists go down a rabbit hole on a project. We need to focus on the business problem.”
TL;DR?
So, the key takeaways if you’re in business and you want to get the most out of your data scientists are:
- Ensure you specify very clearly the problem you want to have solved – and what value the solution will bring the business.
- Respect the ‘playground’ environment of pure science – data science is science first and foremost. There is every chance that you will be paying for perceived ‘failure’ at some point. It will be worth it.
- Understand that work on solving different parts of the big problem gets you closer to the answer to the big problem you think you have. Patience may be expensive – but it’s also sometimes essential.
- Educate your data scientists on the business proposition, to avoid their disappearing down research rabbit holes that do not advance your project.
- Fundamentally, ensure that two-way communication between the business and the data scientists is maintained. That way, the anxieties of each side can be talked out, and each can learn from the experience of the other.
Manage all that, and you’ll be making the most of your data scientists.