Sitting up straight? Machine learning strikes the right pose
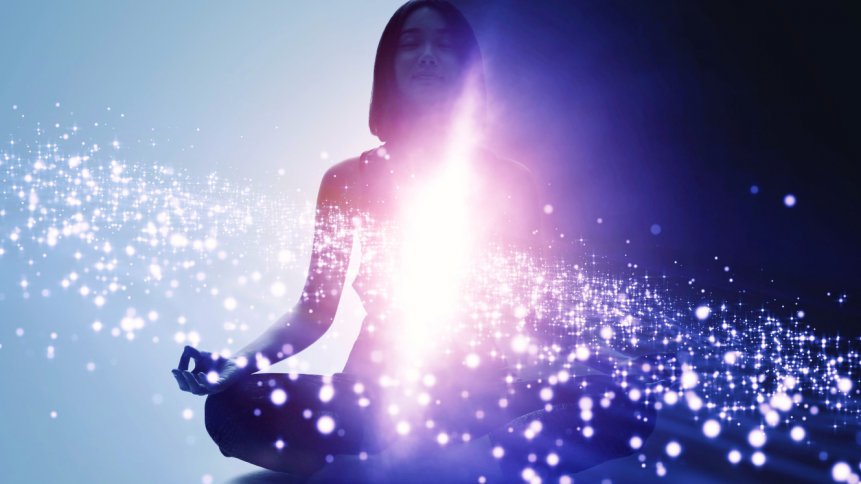
PC’s and laptops are indispensable work tools. And if you were in any doubt, EuroStat (the EU’s statistical office) reports that as many as 85% of employees use a computer for work-related tasks. For those of us that aren’t sitting up straight – even when motivated by an array of ergonomically encouraging mice, keyboards, chairs, and other well-intentioned kit – our computing activity could add up to plenty of aches and pains over the longer term. Fortunately, machine learning experts may be able to provide the necessary prompts, using a selection of data-driven devices tuned to detect differences between healthy and high-risk postures.
“Prolonged computer-related work can be linked to musculoskeletal disorders in the upper limbs and improper posture,” write researchers based at the Technical University of Varna (TU-Varna), Bulgaria, in the journal Computers, as part of a collection of studies focusing on advances of machine learning and deep learning in health. Motivated to find a solution to prevent people from sitting poorly at their PC’s and laptops, the team set about gathering training data from willing subjects fitted with motion capture sensors.
Helping robots determine texture
“The system included 59 sensors, of which 21 were placed on the body, and 39 were placed on the fingers of both hands,” explained the team. “Each recording session included three sub-sessions—standard keyboard and usual posture, ergonomic keyboard and usual posture, and ergonomic keyboard and correct posture”. Having monitored all of the scenarios, the next step was to extract the key features. And to do that, the researchers first pre-processed the information using so-called Hjorth parameters, which convert raw numbers into more meaningful data. This approach, which has its origins in signal processing, has been used to find patterns in brain activity and, lately, to help robots determine texture.
In the current study, the researchers fed their numbers into a machine learning toolbox to identify the similarities and differences between the different setups. A key finding was that even a relatively small change, such as swapping keyboards, could lead to a big improvement in posture, as recorded by spine position. Also, interestingly, the position and movement of a subject’s arms were found to be tell-tale signs of whether the user was sitting with good posture or not. During testing, the machine learning classifier was able to distinguish between good and bad sitting postures 98.4% of the time, based on the full set of sensor inputs. Significantly for device developers, results based on a smaller subset of inputs, which would be more practical to implement on a day-to-day basis, were still accurate more than 90% of the time.
Apps and peripherals
The group’s results suggest that simply placing sensors on the fingers, or even just on the thumb and pinkie (which were sensitive markers of typing position) could be sufficient to detect whether a wearer was adopting a good posture, slipping out of one, or sitting in a way that could be harmful over the longer term. In terms of what’s out there right now, there are a number of solutions, which include setups based on cameras. Developers also point to the use of readily available, and relatively low-cost, peripherals such as Microsoft’s Kinect (now integrated as part of the computing giant’s Azure platform), which have the ability to gather depth information. Intel’s RealSense is another example. There are also seated posture apps that run based on the feed from your webcam.
Posture assessment based on image capture is certainly compelling for manual handling and manufacturing scenarios, which includes work published this year by engineers in Taiwan on scoring real-time occupational assessments using a combination of machine learning and joint angle measurement. However, one concern with video or camera analysis is that users may have reservations in terms of privacy, which is another reason why alternative sensing solutions might turn out to be more popular – at least in the office.
World of ideas
Engineers in Thailand have proposed an IoT-enabled smart chair that they claim is able to detect five sitting postures with 96.2% accuracy, again based on a machine learning approach. And a team in India has devised a belt that warns of poor sitting habits by monitoring the position of the wearer’s spine. There are wireless solutions too such as configuration featuring an RFID antenna and a single RFID tag, as demonstrated by scientists in China. The idea here is that a user sits in between the antenna and the tag, and receives prompts on their posture based on how their body attenuates the wireless signal being broadcast. Again, like the smart chair solution, the RFID setup was able to recognize five postures – sitting upright, and a variety of other positions, including leaning back and bending forwards over the keyboard.
Rolling back to the TU-Varna team, the scientists’ prediction for the future of posture analysis and prompting is greater use of smart textiles – for example, smart clothing that features integrated accelerometers. There could be cross-over applications here too in the world of sports, providing data for professional athletes and their coaches.