Can Robots Have Team Spirit? They Can Now
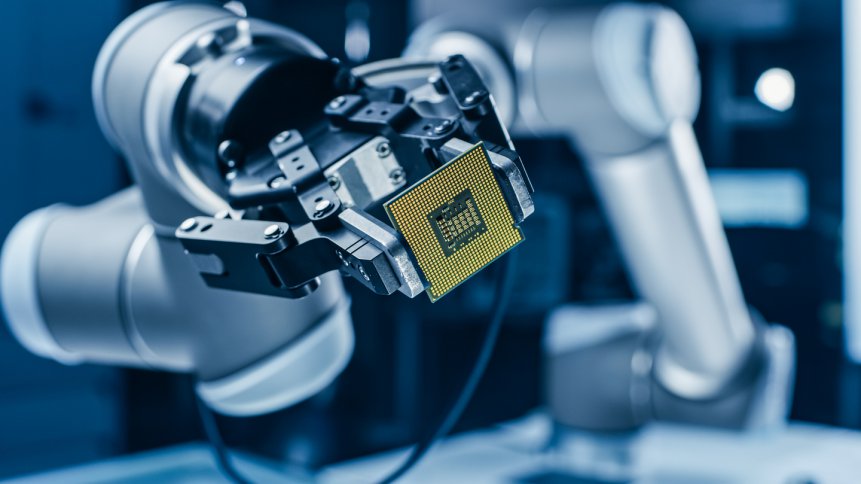
Robots are a fundamental part of the digital transformation of several sectors of industry, from manufacturing to warehouse management. But there has always been an issue with multiple robots (or drones) conducting collaborative actions. When lines of data communication between them are open, we’re now at a point where robots can co-operate at least as efficiently as humans, but with significantly greater speed, strength, and overall efficiency – that’s a fundamental element of many modern production plants.
But when those lines of communication are down, or unavailable, the collaboration quickly runs away from true and either becomes clunky or stops functioning altogether – neither of which is genuinely acceptable in the tight-margin, high-productivity business sectors that invest heavily in robotics precisely to ensure consistency and speed, like the chip-making sector.
Go Team Robot!
Now, students at the University of Illinois Grainger College of Engineering have developed a method by which robots and drones can be trained to continue acting collaboratively with little decrease in efficiency, even when communication between them is down or impossible.
The method is based on multi-agent reinforcement learning, a kind of AI which learns based on previous iterations of actions. The machine learning process builds in a kind of utility function which essentially tells the robot or the drone when it is performing an action that is positive for “the team.”
The concept of the team is not one that has been widely used in robotics before – most robots are programmed with what might be thought of as “individualistic” function sets. Huy Tran, one of the researchers behind the collaborative breakthrough, said “With team goals, it’s hard to know who contributed to the win. We developed a machine learning technique that allows us to identify when an individual agent contributes to the global team objective. If you look at it in terms of sports, one soccer player may score, but we also want to know about actions by other teammates that led to the goal, like assists. It’s hard to understand these delayed effects.”
The Fumbled Pass
But if robots with learned team spirit can work towards recognized team goals, irrespective of communication with the other members of the team, a degree of negative reinforcement is also necessary. So the researchers from Illinois Grainger also trained their machine learning model to recognize actions that don’t contribute to the team goal, so that the robots would need no additional communication or confirmation that such actions were to be avoided.
This is not the digital equivalent of shame for performing an incorrect action, merely a refined training to avoid actions that can be identified as not contributing to the overall team goal.
How do you teach a robot the value of collaborative team spirit, when such a concept is outside the boundaries of its ‘natural’ inclinations? Essentially, you teach it in the same way you would teach an ordinarily egocentric child to develop their superego and work together with team-mates – you test your ‘parenting’ algorithms by playing games with the robot, and seeing whether their collaborative ability develops in response to your training and the situation in which it finds itself.
Game On!
The Illinois Grainger team trained their collaborating robots by testing their machine learning algorithms in repeated, simulated games of Capture The Flag, and in particular, games of a populate computer game called StarCraft. While Capture The Flag has a few fairly standard variables – equivalent to the situations in which the robots, once released into the manufacturing or warehousing environments, might face every day, StarCraft has more curved ball situations, which allowed the Illinois Grainger team to observe how their machine learning algorithms coped with unexpected turns of events.
Tran reported that the algorithms withstood the curved ball scenarios surprisingly well, resulting in robots with a significantly robust team orientation even when the unexpected happens in the real world.
That also means that the scope of the uses to which such communication-blind collaborative robots could be put in the real business world broadens significantly. As well as working collaboratively in warehouses and factories, it’s possible such team-oriented robots could also be used in more command and control, decision-critical situations, like military surveillance drones, high-pressure urban traffic signal control, operating a complex regional or national power grid, and even in the systems controlling autonomous vehicles – especially in terms of safety protocols in complex environments.
The Collaborative Future
While the research is fairly fresh, and so it might take some time to filter through into the commercial market, it’s an extremely positive development in AI and machine learning that there are algorithms for team-goals in and of themselves. In fact, while the Illinois Grainger results extend the scope of collaborative robots that have no communication, communicating collaborating robots are already out there in the workplace. So, once the work is robustly tested and proved in complex environments, it won’t have to re-invent the wheel to find itself a place in the business world.
But by extending the capabilities of non-communicating collaborative robots, it could be the basis of a new level of digital transformation, as collaborative robots (or computer systems optimized towards team goals) would be significantly less likely to break down or snarl up a production system any time they were out of data communication range – and therefore less likely to need regular human intervention in the processes of their work.