Does AI Practice Electric Bigotry?
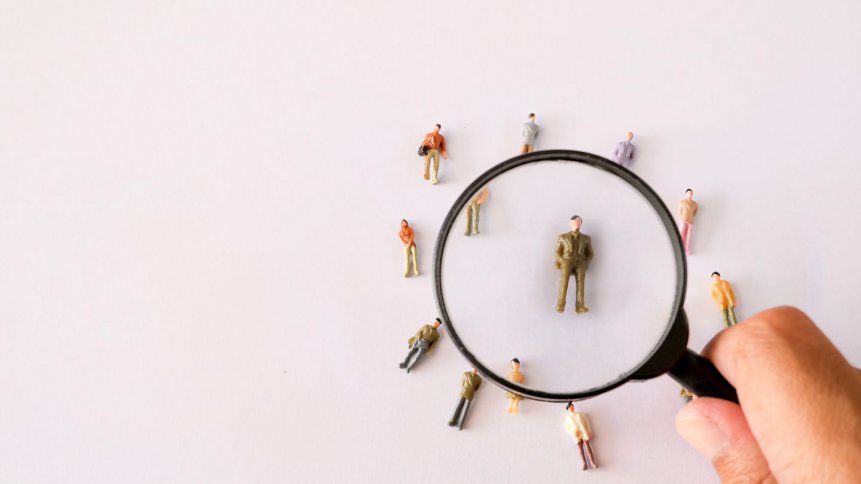
The point about AI algorithms is that they take available data, funnel it through a process, and output a result – and they do it fast. Faster than humans ever could. And they learn from their previous experience. In any logical setup, it should be impossible for AI algorithms to reproduce, for instance, a bias against black people when sifting candidates for a job, or a bias against candidates with Autism Spectrum Disorder.
But the UK Information Commissioner’s Office (ICO) is to investigate whether AI algorithms to sift applicants in recruitment are transferring unconscious biases into the recruitment process, effectively computerizing the process of prejudice in the jobs market, and shutting out potentially qualified candidates on the basis of racial or neurodivergent data.
The Human Factor
The fact of the matter of course is that it’s by no means impossible for AI algorithms to reproduce bigoted or exclusionary results. If the process applied to the raw data is created from a positional norm that has only a narrow band of acceptability in expression, for instance, neurodivergent people may be weeded out on the basis of the way they express their answers to questions, which may fall outside the boundaries of acceptability the algorithm has been fed. That will be an inaccurate and discriminatory result based on an unfairly limited definition within the data processing part of the algorithm.
And of course, in AI, the longer a process runs without flagging an error or getting human correction, the less likely it is to be understood as being in error – and without detailed human checking of the candidates who were not selected to be interviewed, and why, the issue can go on undiscovered indefinitely.
So, the short answer would be yes, AIs can practice electric bigotry – but only if they’re flawed in their initial setup by the human factor.
READ NEXT
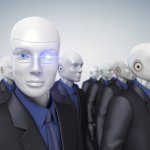
Is AI Failing Businesses?
That’s not to necessarily suggest that the people programming AI algorithms are actively racist or biased in favor of neurotypicality – though the ICO pointedly said it would be investing the negative effects such algorithms could be having on people from communities who were not represented when the algorithms were tested.
Norms and Data
That means any AI programmed with initial conditions or data sets exclusively by people who fit a societal norm of ‘success,’ and tested only on people who also fit that norm, will inherently go on to associate Candidate Group=A – people who look, speak, talk, think, and in recruitment terms, write like the developers and the test group – with “Good Candidates,” and may well then exclude Candidate Groups B, C, D, and more from that “Good Candidates” group, producing a homogenous group of candidates going forward to interview, and shutting out those who don’t conform to the parameters of Candidate Group A on some potentially work-irrelevant points.
There’s a way of looking at this as a symptom of the system biases that currently exist in society. Within what remains at heart a pale-skinned, moneyed patriarchy, there are levels to the ‘norm of success’ – with rich or middle-class, non-disabled, cis, heterosexual, white men at the top, and everybody who differs from that template in some degree going down the ladder a step for every degree of difference.
But you don’t need AI algorithm-programmers and testers who are all rich or middle-class, non-disabled, cis, heterosexual, white men to end up with an algorithm that excludes people who differ from the template on the basis of their points of difference. All you need to get that result is a creative and testing group that doesn’t flag up any difficulties with the process. And in a society geared towards providing smooth results that look the same, you need as many difficulties as possible flagged up during testing. That means your AI testing panel has to be as diverse as possible, so that it leads to an eventual AI that won’t auto-dismiss candidates because of their skin color, name, method of expression.
The Harvard Study
The surprising thing about the ICO’s decision to investigate is that it was necessary in 2022, given that in 2019, a study by the Harvard Business Review, conducted with professionals from both Northeastern University and the University of Southern California confirmed that AI recruitment tools “reflect, recreate, and reinforce anti-Black bias.”
Its analysis of job board recommendations found that 40% of respondents said they had experienced recommendations “based upon their identities, rather than their qualifications.” 30% said that the job alerts they received from the board were for positions beneath their current skill level. Similarly, almost two-thirds (63%) of respondents said that academic recommendations made by the platforms were lower than their current academic achievements.
That’s an AI recruitment platform essentially telling black candidates that “surely” they can’t aspire to higher-paid or more responsible jobs. It wasn’t necessarily programmed that way, but it may have been tested – or more likely, learned from repeated data trends that successful candidates for higher-paid, more responsible jobs had other attributes.
Never Send An AI To Do A Human’s Job?
These issues have raised the question of whether AI – and indeed, the people building the AI, and the societal norms in which they live – is sophisticated enough yet to be used in recruitment. Certainly, Amazon no longer thinks so – in 2018, the company noticed that its AI recruitment tool was selecting principally men for roles, having used a data sample from a ten-year period in which the majority of successful applicants were men. Taking this as its norm, the AI selected for repetition, rather than differentiation, despite there being several highly viable women among the applicants who applied.
In fact, the system actively penalized those candidates whose resumes included the word “women” – as in “women’s programming club” or “women’s basketball team.” In a relatively short space of time, Amazon’s recruitment AI effectively taught itself misogyny, and began recruiting accordingly.
After trying quite hard to un-write the machine misogyny, the team trying to fix the AI was eventually disbanded.
It’s arguable that human-to-human recruitment isn’t the whole answer, either – unconscious bias in interviews is absolutely still a factor in recruitment in the 2020s. But until we can edit out the unconscious biases that we add in to AI algorithms, it’s possible that a multi-human panel approach, with correcting voices to balance individual biases, is a more effective way of leveling the playing field in recruitment than letting potentially uncorrected AI do our candidate-sifting for us.