3 ways attitudes to enterprise ML shifted in 2020
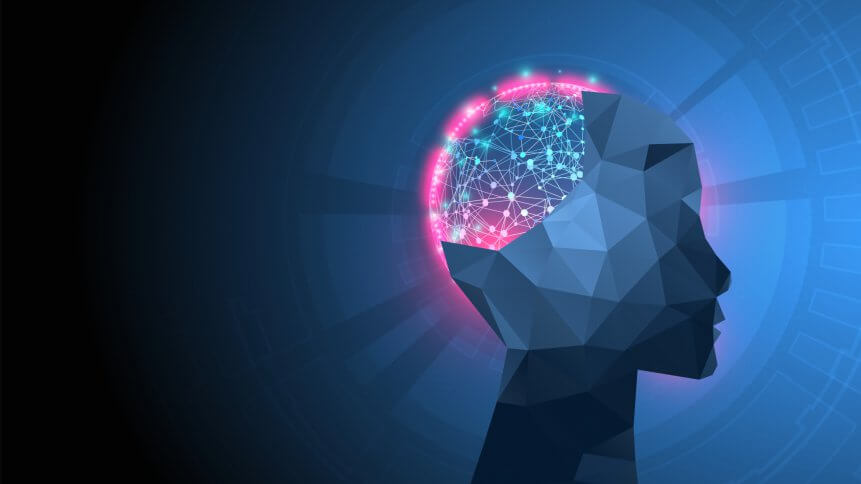
2020 was a year of belt-tightening for many organizations, due to that old pandemic and the impact of social distancing and general uncertainty on more or less every market.
In May this year, Gartner predicted global IT spend would fall 8% over the course of the year as business and technology leaders refocused budgets, prioritizing things like cloud collaboration solutions and cybersecurity.
We might be prone to think investments in technologies like artificial intelligence (AI) and machine learning (ML) have been shelved for now. But a new report from Algorithmia suggests that’s not the case. Not only has the upheaval of 2020 not impeded AI/ML efforts that were already underway, but it appears to have accelerated those projects as well as new initiatives.
A key takeaway from the blind study, which included 403 business leaders involved in machine learning initiatives at companies with US$100 million or more in revenue, is that enterprise IT departments are increasing machine learning budgets and headcount despite the fact that many haven’t learned how to translate increasing investments into efficiency and scale.
This suggests that, in light of the accelerated digital transformation taking place by the necessity of newly-remote workforces, businesses are anticipating increased competition in the year to come, and view AI/ML technology and talent as a strategic investment to improving productivity and resilience.
Companies are turning to their investments in AI to deliver both short-term cost-cutting and long-term technology innovation to drive revenue and efficiency. Here are four stand-out trends from Algorithmia’s 2021 enterprise trends in machine learning.
# 1 | Organizations are increasing AI/ML budgets, staff, and use cases
Organizations were increasing their investments in AI/ML before the pandemic, according to the report, and the economic uncertainty of COVID-19 has added to the urgency. The 2021 survey revealed that 83% of organizations have increased their budgets for AI/ML and that the average number of data scientists employed has increased by 76% year-on-year.
In addition, organizations are expanding into a wider range of AI/ML use cases; the survey found that the percentage of organizations that have more than five use cases for AI/ML has increased 74% year-on-year.
Notably, the top use cases that organizations are focusing on are related to customer experience and process automation—areas that can offer top-and bottom-line benefits during times of economic uncertainty.
# 2 | Challenges span the ML lifecycle, especially with governance
Organizations are experiencing challenges across the ML lifecycle, with the top challenge by far being AI/ML governance. 56% of all organizations rank governance, security, and auditability issues as a concern — and 67% of all organizations report needing to comply with multiple regulations for their AI/ML.
In addition to governance challenges, organizations continue to struggle with basic deployment and organizational challenges. 49% of organizations ranked basic integration issues as a concern, and the survey found that cross-functional alignment continues to be a major blocker to organizations achieving AI/ML maturity.
# 3 | Despite increased budgets and hiring, organizations are spending more time and resources — not less — on model deployment
Despite the increase in budgets and headcount, organizations are now spending more time and resources on model deployment than they did before. Algorithmia found that the time required to deploy a trained model to production increased year-on-year and that 64% of all organizations take a month or longer to deploy a model. 38% of all organizations are spending more than 50% of their data scientists’ time on model deployment — and organizations with more models spend more of their data scientists’ time on deployment, not less.
The bottom line is, organizations have increased their AI/ML resources without solving underlying challenges with operational efficiency. This has exacerbated the problem and led to organizations spending more time and resources on model deployment.
“COVID-19 has caused rapid change which has challenged our assumptions in many areas. In this rapidly changing environment, organizations are rethinking their investments and seeing the importance of AI/ML to drive revenue and efficiency during uncertain times,” said Diego Oppenheimer, CEO of Algorithmia.
“Before the pandemic, the top concern for organizations pursuing AI/ML initiatives was a lack of skilled in-house talent. Today, organizations are worrying more about how to get ML models into production faster and how to ensure their performance over time.”
Oppenheimer was encouraged, he added, that challenges with AI/ML are less to do about whether or not organizations should invest in them at all, and more about how to maximize their value.