Key factors driving AI in 2020
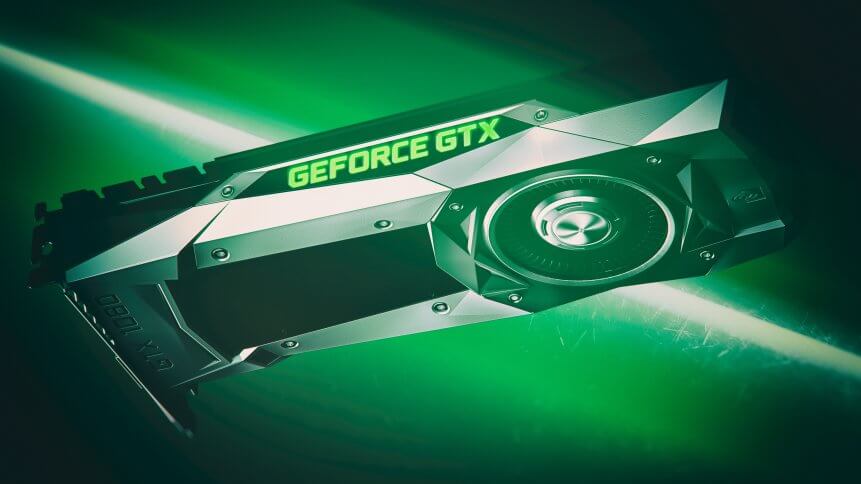
AI has changed. The Artificial Intelligence (AI) that we first knew in the Science Fiction (Sci-Fi) movies of the 1980s was portrayed as fanciful magic, where computers would talk to us like humans and be able to understand our needs, hopes and perhaps even our emotional desires.
The trouble, a quarter-century ago, was that the IT industry was conceptually capable of building the logic constructs and computation engines that would deliver AI, but even the smartest techies were held back by several factors… not all of which their fault.
Three defining factors
Today’s AI has changed because the developers building it have produced vastly more sophisticated algorithms than those that were driving initial forays into this field.
Secondly and crucially, our new AI systems have also benefitted from access to massively widened datasets that were never available before the birth of the Internet and cloud data centers.
Thirdly, computers have quite simply become more powerful.
They have become faster at processing (with some boosted by the additional charge offered by Graphics Processing Unit technology), bigger in terms of their data storage capacity and more intelligently internetworked into clusters of computing power across distributed networks.
These combined forces have come together to give us the ‘new AI’, or at least the AI that is now driving areas of software application that can take advantage of automation efficiencies like Robotic Process Intelligence (RPA). As AI-powered RPA now helps to shoulder the drudgery of repeatable human work tasks, several other factors will drive the way AI develops next.
YOU MIGHT LIKE
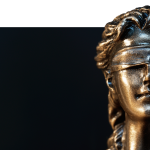
Why fair artificial intelligence might need bias
Open route to standardization
The next wave of AI will be heavily influenced by the use of open source technologies. This is because the open model of community contribution focused shared codebases has become the de facto route for any technology to enjoy ubiquitous standardization on the route to going viral.
As we now work to refine, extend, augment, model, build and debug our AI systems, it is important that we do so in open [source] arenas where the collective mass effort of entire software communities can help us get smarter.
To get that ubiquitous standardization to happen, we will need to increase our efforts to eradicate AI bias and increase AI ‘explain-ability’. Sometimes known as explainable AI (or XAI for short), this notion of computer intelligence produces results in a way that can be understood by a human being.
Some XAI includes features such as ‘what if’ tools to tweak AI software engines and experiment with results as the AI crunches through data. There are also options for users to view a score explaining how each dataset factor contributed to the final result of the model predictions.
Wider application of AI
As we get even better at AI (and XAI), we will see its implementation surface widen across areas including image recognition, translation, speech recognition, autonomous driving and the still-nascent areas of assisted decision making.
Assisted decision making won’t be fully responsible for managing people, driving teams, influencing entire departments or running whole companies in the foreseeable future, but it will drive in an increasing amount of autonomous controls where business actions are taken without human interactions being required.
Major database vendors are spinning this line and calling their systems autonomous because the database knows when to perform tasks like data duplication, patching, provisioning and the chore of the ‘nightly build’ where databases have to engage in internal mechanics to serve the needs of software developers.
YOU MIGHT LIKE
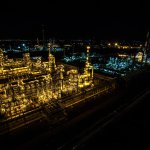
How wide is the RPA ‘kill zone’?
Abstract AI artistry
Because of this reality, we can say that a good deal of AI development will happen at the back end of the computing stack, but it is how AI is used at the upper tier in the user front end that may influence its next growth phase even more.
AI will be working away in the back end, yes, that’s where it lives. But we will now start to use an increasing amount of tools that ‘abstract away’ its complexity and allow users to interact with it in the simplest ways possible.
In practical terms this means the ability to ask questions of AI systems in natural human language or speech. Software engineers are now developing these abstraction layers to embed AI smartness into apps that we’re already using.
So, in 2020, we can say that AI is getting smarter, more ethical, more explainable, used across a wider transept of use cases at both the front and back end of the IT stack and increasingly abstracted. And you didn’t even need an AI engine to tell you that, right?