AI in healthcare requires a measured dose
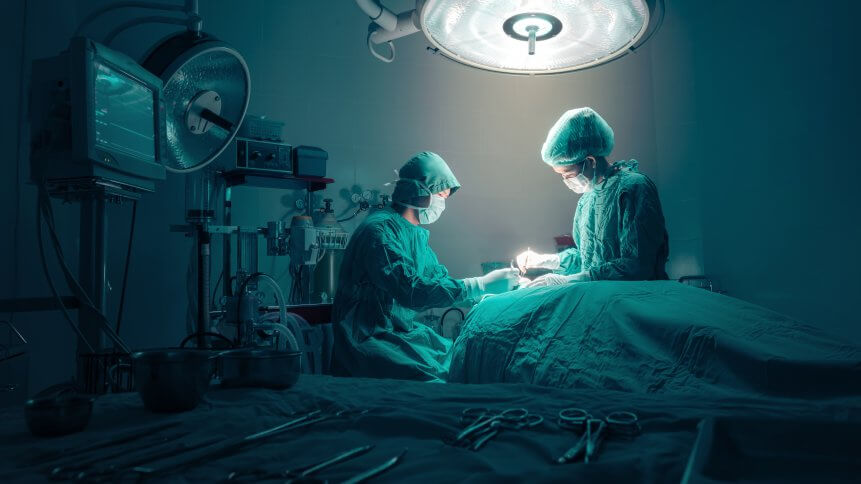
According to Statistics MRC, the global market for AI in healthcare could reach US$19.25 billion by 2026. The high capital requirement is hindering market growth, but some players are already standing out.
Although this is a new frontier, a lot of the leadership is coming from IBM, which remarkably is now a 108-year-old tech company. IBM Watson Health has brought together researchers, data scientists, clinicians, public sector officials, and business leaders to address the biggest challenges in healthcare and build smarter health ecosystems.
IBM’s CareDiscovery product offers proprietary methodologies that can detect unseen inefficiencies in clinical care and compare performance against different benchmarks.
While developing these tools, the company used a massive database of 21 million all-payer discharges from over 2,700 hospitals. And although this harvesting of data may give some people pause, the effects are already being felt in the quality of care and patient outcomes.
When AHMC Healthcare, a hospital corporation in California, needed to improve clinical operations for sepsis management, they turned to this product in order to move away from manual, labor-intensive processes for data collection. They were then able to evaluate system performance with a higher degree of precision.
Sepsis is essentially a self-destructive bodily response to an infection. It’s the most expensive condition treated in US hospitals but early diagnosis and rapid initiation of appropriate treatment can help to contain the damage and cost.
Healthcare organizations have specified evidence-based process measures for reducing morbidity and mortality. However, only 49 percent of US hospitals were in compliance with these standards in the first nine months of 2017.
With IBM’s technology, AHMC Healthcare was able to pinpoint the breaking points throughout operational processes and boost compliance from 10 percent to 100 percent in some facilities.
More broadly, technology offers a way to automate and contend with an American healthcare system that could be reasonably characterized as inherently dysfunctional. A Watson Health blogpost about medical imaging automation and workflows clearly alludes to this: “Connecting with referrers and payers often involves a large amount of time and energy to deal with faxes, phone calls and paperwork to confirm clinical data or secure preauthorizations — all in an effort to head off claim denials.”
At a time when data is increasingly informing decision-making regarding diagnostics, treatment selection, and overall operations, there is money to be made in healthcare AI. The Statistics MRC report suggests that a lot of those profits will come from overseas.
Asia Pacific holds the largest market share during the forecast period, due to increasing R&D expenditure and developments in the pharmaceutical and biotechnology sectors. Also, the sheer number of people and the growth of their incomes could generate demand for improved healthcare services and drive regional market growth for associated technologies like AI.
Throughout global healthcare, data helps but risks remain. AI in healthcare is improving hospital operations, but some forward-thinking doctors are prescribing better regulation, transparency, and the peer-reviewed substantiation of marketing claims.
As in other sectors, there is a risk of overreliance on new technology, however impressive it may be or initially appear to be. Good clinical judgment still has an important role to play and can help to detect false triggers. Documentation errors or delays and the complexity of healthcare measures may also distort the perception of hospital operations.
In a recent medical journal article, Thomas J. Hwang, AB, Aaron S. Kesselheim, MD, JD, and Kerstin N. Vokinger, MD, JD, Ph.D. noted that last year the FDA cleared an AI program for diabetic retinopathy that provides screening decisions without the need for clinician interpretation. The authors wrote, “Although such technologies hold promise, they also raise questions about their safety and effectiveness.”
In the same way that some new healthcare tech allows for a comprehensive view of hospital operations, we may need to take a more comprehensive view of these transformational technologies.
According to the JAMA authors: “The distinctive characteristics of AI/ML-based software require a regulatory approach that spans the lifecycle of these technologies, allowing necessary steps to improve treatment while ensuring that the algorithm is safe.”
There may also be a cause for concern here because the FDA approval process contains pathways that could be characterized as “loopholes.” These authors diplomatically didn’t use that term… but I will, and the disquieting Netflix documentary “The Bleeding Edge” makes the case for anyone who’s on the fence.
If there’s “substantial equivalence” to an already-marketed device, then a new medical device can be expeditiously cleared based on that imprecise metric and spared from a more stringent review process. Between 2017 and 2018, the FDA cleared 11 AI/ML-based software products as devices through this pathway. No devices were approved through the more demanding premarket approval pathway.
It may be time to rewrite the rules to both foster innovation and ensure safety but in so doing regulators may end up specifying arbitrary timeframes that complicate business interests.
The JAMA article authors wrote that it is unclear if the existing regulatory model is suitable for AI/ML-based software, which is inherently iterative. They explained, “AI/ML-based software can change its behavior in real-time after it is distributed and has ‘learned’ from clinical application and experience.
“This continuous learning could result in outputs that differ from what was initially reviewed prior to regulatory approval.”