The dark side of artificial intelligence
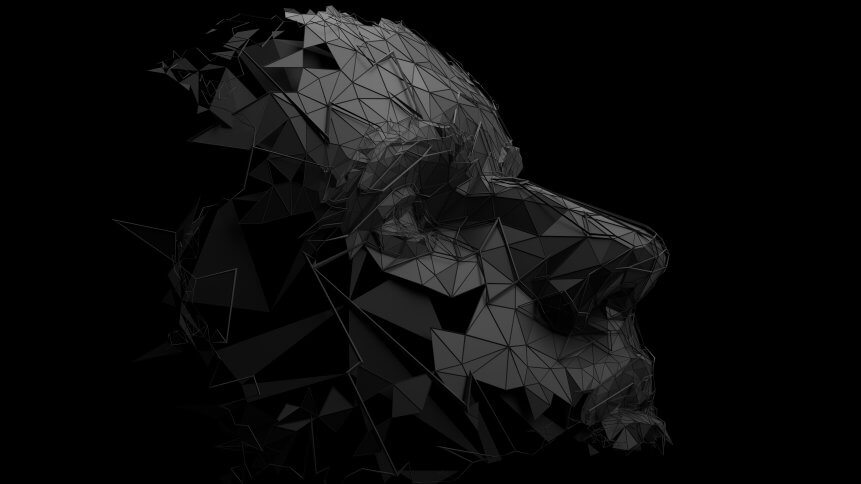
All forms of machine learning (ML) and artificial intelligence (AI) are great news and are increasingly easy to implement in modern business environments, many of which are already feeling the benefits of AI-driven predictive intelligence.
You may stop and ask yourself what’s wrong with the above statement.
The truth is, ML and AI are hard to design and architect, tough to apply and sometimes provide the wrong kinds of results, which ultimately fail to help the business case that initially justified their spend.
When chatbots go wrong
We know that AI chatbots can go wrong. Microsoft’s already infamous episode with the profanity-spouting Tay chatbot showed us that human speech and personal interaction should (in some sensitive areas at least) remain the sole preserve of real humans.
But badly trained out of control chatbots are just part of the challenge; it is actually really tough to get the right data into an AI and ML engine in the first place.
Given that we’re talking about the rise of so-called ‘citizen data scientists’ (business users and other non-technical staff who will use automation tools to apply AI to business use cases), should we stop and ask whether we are putting too much power into the wrong hands?
People who build ML tools have to make choices. These are choices governing how data should be processed, which features should be showcased, which algorithms should be selected, how data models should be tuned and refined, how these models be should be deployed— and a whole lot more.
Machine learning to the rescue (of ML)
This responsibility has given rise to the birth of what has been called automated machine learning (or AutoML) tools and several exist in both commercial and free and open source formats. AutoML exists to do all the ‘working out and preparation’ for the user so that the intelligence itself is constructed correctly.
It is, if you will, machine learning coming to the rescue of machine learning.
Without going into the detailed mechanics of hyper-parameter tuning and all the other AutoML features a user can avail themselves of, this new branch of data science is not always guaranteed to produce the right results if it is applied in the wrong environment.
“Data and algorithmic literacy is a key problem facing organizations that do not go away with AutoML tools: users still need a basic understanding and awareness of the ML process and techniques, and senior management need to understand what AutoML can, and cannot, do,” said Paul Clough in his role as Professor of Search & Analytics at the University of Sheffield.
Professor Clough argues that despite the current hype surrounding AI that is built around AutoML, it will not fully automate the end-to-end process of data to insight (and action) as is often suggested; some aspects will remain largely manual and require human intervention.
YOU MIGHT LIKE
Is the finance sector floundering with AI?
Deeper, darker, data discoveries
Looking deeper into the darker side of AI, we are also seeing its influence on the still-nascent area of customer experience (CX). Analysts are now conducting studies that examine the application of AI in terms of its application to serve customers and deliver support services.
A recent Forrester Consulting study found that poor self-service and automation capabilities are leading to frustrated customers and increased call center volume– which conversely is not a typical challenge for firms who are more mature in terms of their application of CX practices.
What this is doing is amplifying the distance between those firms who have embraced and adopted the whole CX movement and realized that they can’t simply go to market with the same old product or service that they always have without really caring about the customer— and those that haven’t.
As we know, in the modern age of social media, customers are very quick to voice discontent openly and this has led to the rise of far more customer-centric care across almost all industry verticals. If firms fail to use AI services that positively increase customer engagement, they risk falling behind.
According to the Forrester Build Competitive Advantage Through Customer Engagement and AI report, “Exceptional customer experience is a cornerstone of business success. Better customer engagement leads not only to higher customer satisfaction but also to greater top-line revenue growth and more satisfied customer-facing employees.
“Organizations with greater engagement maturity reap benefits, not only more often, but of greater value, than less mature companies.”
Despite all this negative naysaying, we will get there. These are the teething pains that we were bound to go through on the road to AI-enriched digital transformation. If we understand that there is a dark side to AI, then we can look for the light at the end of the tunnel.