Closed-loop AI: robots building better batteries in the lab
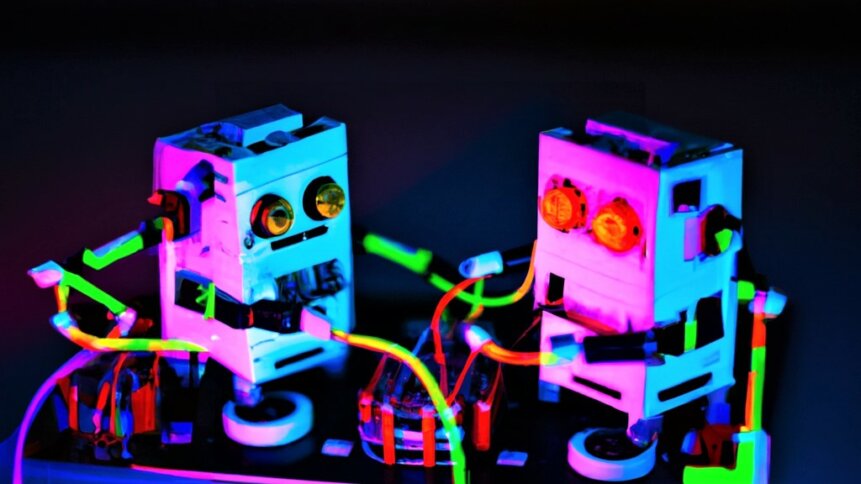
Getting your Trinity Audio player ready...
|
Artificial intelligence (AI) brings a kind of magic to various business needs – from opening up access to company legal advice to predicting what types of products customers want to buy. Systems are becoming so capable at workplace tasks that some people are wondering whether AI will take our jobs. But few would complain if machines could build better batteries so that smartphones and other portable devices lasted longer between charges. And that’s where closed-loop AI methods, featuring robot lab assistants, are making their mark.
Publishing their latest results in the open-access journal STAM methods, researchers in Japan have unveiled NIMS OS – a Python library for automated materials exploration. The orchestration system, which is available on GitHub, features closed-loop AI that generates a proposals file of materials combinations for robot testing and then refines the most promising candidates based on those experimental results.
As the team – which includes members of the Center for Advanced Battery Collaboration and the Research Center for Energy and Environmental Materials, both part of Japan’s National Institute for Materials Science (NIMS) – explains, closed-loop AI is the next step in building better devices.
“Robotic experiments have progressed to realize laboratory automation of chemical analysis and high-throughput screening in the field of biology,” write the researchers in their paper. “In addition, many methods have been proposed for black-box optimization in materials exploration, such as genetic algorithms, Monte Carlo tree search, rare event sampling, and algorithms using an Ising machine.”
Vertically integrated, closed-loop AI systems are the future of the physical world. Love this robotic weed murder machine. h/t @byjacobward pic.twitter.com/oASjG2STVH
— Aaron Slodov (@aphysicist) June 12, 2023
Closed-loop AI in the field.
Combining these developments into a closed-loop AI approach for novel materials discovery not only accelerates the search for better batteries and device elements, but also removes human error from experimental results gathering.
NIMS OS divides up the process into a series of modules that represent either individual AI algorithms or robotic systems. And users can engineer the closed-loop AI automation necessary for their materials discovery projects by assembling combinations of the various software modules.
Digital transformation in the lab
One of the most commonly used machine learning approaches in the field is so-called Bayesian optimization (BO), which is a statistical approach to optimizing design parameters. “Using BO, various real materials, such as Li-ion conductive materials, multilayered metamaterials, halide perovskite, superalloys, and electrolytes, have been explored,” the NIMS OS group explains.
Currently, the developers have implemented three types of AI algorithms, including BO, in their closed-loop AI platform. Examples include a Python package for exploring thin films and polymers as well as a predictor for selecting the next round of experimental conditions, which is based on random forest regression. And, to keep things interesting, there’s also a random exploration option. However, this is primarily used as a starting point for the AI algorithms, which should –as the robot gathered experimental results accumulate – converge towards promising materials candidates.
Closed-loop AI hardware
On the hardware side of the closed-loop AI, the setup features a liquid-handling dispenser, an electrochemical measurement unit, and a robotic arm. The apparatus is designed to enable high-throughput evaluation of electrolytes – for example, to improve the performance of lithium metal electrodes in batteries (a test scenario used by the team to evaluate NIMS OS).
A closed-loop AI discovery session progresses from parallel experiments initiated using random exploration to cycles of testing, which can add up to hundreds of evaluations – all performed automatically.
In their demonstration, the researchers investigated the optimum combination of 16 electrolyte additives and the potential of the composition to improve battery performance – evaluated by measuring the discharge time for a given current density. A total of 384 experiments were performed, and the results mirrored wisdom in the battery technology world, which suggests that the system is capable of following a credible investigative path.
The next step for the team is to expand the software to include a variety of automated experiments to widen the number of different systems that can be studied. However, the current setup already highlights how battery development can be accelerated using closed-loop AI methods.
And the future could be even brighter thanks to quantum computing architecture that promises to further speed up the search for improved device materials. The hope is that quantum computers, which – by design – are capable of considering multiple solutions at once, will provide an alternative to current trial-and-error style investigations.
Typically, it can take anywhere between 20 to 30 years to translate novel materials from first discovery into the marketplace. And, given the importance of advanced materials in key areas such as renewable energy, including battery storage, it’s easy to understand why governments are keen to find a shorter path from the lab into commercial devices.
In 2011, government agencies in the US teamed up to support what’s dubbed the Materials Genome Initiative – a program with the ambitious aim of ‘discovering, manufacturing, and deploying advanced materials twice as fast and at a fraction of the cost compared to traditional methods.’
There have been highlights in the decade that followed, but moving the needle on finding new materials for the billions of smartphones, laptops, tablets, and other must have gadgets, is tough going. Plus, there are environmental considerations that go beyond searching simply for better performance – for example, new electrolyte additives need to aid not impede battery recyclability.
However, those considerations can be added as targets and built into the parameter search using automated approaches such as closed-loop AI. The difficulty in discovering device materials that can tick all boxes is sometimes likened to finding a needle in a haystack.
But we can be encouraged by the fact that the number of tools at our disposal is increasing, and automated AI methods – as we’ve come to see in other fields – are highly scalable, providing multiple levers to pull on to boost productivity.