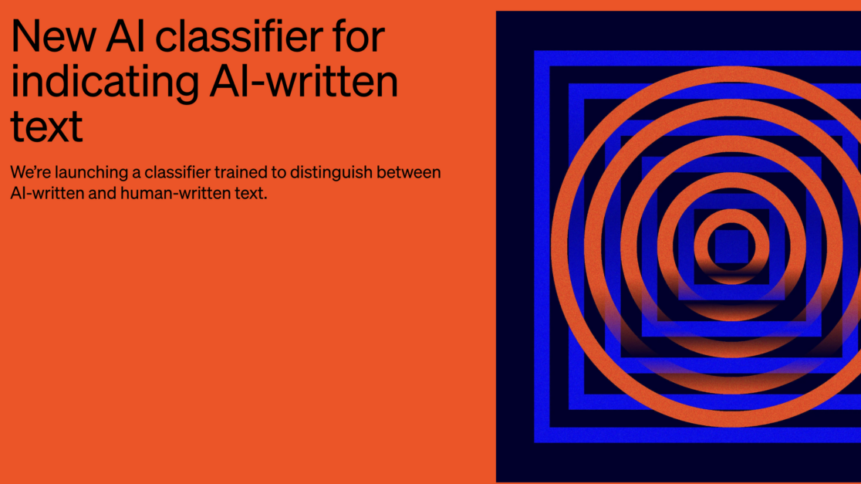
- OpenAI reveals it is scrapping ‘AI Classifier’ in a blog post addition
- When announced, it could correctly identify 26% of AI-written text
- Other detection tools are not much better – we look at why this is
OpenAI, the creator of the world’s most famous large language model (LLM) ChatGPT, has halted work on its AI detection tool. Dubbed ‘AI Classifer’, the tool was first announced via a blog post back in January, where OpenAI claimed it could “distinguish between text written by a human and text written by AIs from a variety of providers”.
Funnily enough, this blog post is also where the company revealed that it would no longer be working on AI Classifier, in an addendum published on July 20. It says that the ‘work-in-progress’ version that was available to try out is no longer available “due to its low rates of accuracy”.
Researchers in San Francisco may have been struggling to improve the system from when it was first unveiled in January, where it could only correctly identify 26 per cent of AI-written text and produced false positives 9% of the time. The recent blog post addition claims that they are now focusing on “more effective provenance techniques for text” but does not share where these techniques will be implemented once found.
OpenAI is not alone in struggling to create a beast to conquer its own ChatGPT and the clones of varying intelligence. A 2023 study from the Technical University of Darmstadt in Germany found that the most efficient tool could only correctly identify AI-generated text less than 50 per cent of the time.

AI Classifier is no longer available “due to its low rates of accuracy”. Source: OpenAI
Some AI-based spoofing is getting easier to spot: Intel’s ‘FakeCatcher’ has a 96 per cent success rate thanks to a technique that detects the changes in blood flow on people’s faces – a characteristic which cannot be replicated in a deepfake.
The difficulties in spotting AI-generated text appear to lie in the fundamental procedures that underpin them. They all generally work by measuring how predictable or generic the text is. For GPTZero, an AI detection tool built by Princeton University student Edward Tian, this is calculated by two metrics; ‘perplexity’ and ‘burstiness’.
The former is a measure of how surprising or random a word is given its context, with more generic phrases more likely to be flagged as AI-generated. The latter denotes how much a body of text varies in sentence length and structure, as bots tend towards uniformity while human creativity favours variety.
However, these are not hard and fast rules. Different human authors have different styles, some more robotic than others, and chatbots are getting better at mimicking these with every new piece of content they digest. This is where the difficulties stem from.
Over the past few months, social media users have discovered that AI detection tools claim sections of the Bible and US Constitution are AI-generated. As Mr Tian himself told Ars Technica, the latter specifically is a text fed repeatedly into the training data of many LLMs.
someone used an AI detector on the US Constitution and the results are concerning.
Explain this, OpenAI! pic.twitter.com/CNPTf8BhYL
— gaut (@0xgaut) April 18, 2023
“As a result, many of these large language models are trained to generate similar text to the Constitution and other frequently used training texts,” he said. “GPTZero predicts text likely to be generated by large language models, and thus this fascinating phenomenon occurs.”
This is not the only issue. Multiple studies have found AI detector tools to be inherently biased. Stanford University researchers found that they “consistently misclassify non-native English writing samples as AI-generated, whereas native writing samples are accurately identified”.
This suggests that the rules the tools are based upon may unintentionally punish those who have not spent their lives immersed in English, and as such have a more limited vocabulary or grasp of linguistic expressions than those who have.
Some tools also show bias towards labelling text as either human or AI-generated. Researchers from the Nanyang Technological University in Singapore evaluated the efficacy of six detection systems on lines of code from online databases like StackOverflow and that had been produced by AI.
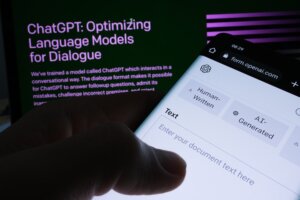
AI detection tools generally work by measuring how predictable or generic the text is. Source: Shutterstock
They found that some tools leaned in a different direction when labelling content and that the individual biases are due to “differences in the training data used” and “threshold settings”.
A separate paper, which compared the results of the Singapore study with others, found that the majority of detection tools were less than 70% accurate and had a “bias towards classifying the output as human-written rather than detecting AI-generated text”. The performance also worsened if the AI-generated text was manually edited or even machine paraphrased.
Indeed, just applying the correct prompt can allow content to bypass detectors. The Stanford researchers claim that telling an LLM to “elevate the provided text by employing literary language” will result in it turning a piece of content from a non-native English speaker that it assumes is AI-generated to one it deems human-written.
Models have been created that can spit out similar prompts which will allow AI-generated texts to evade detection. These are, of course, open to misuse, but can also be used to improve the performance of shoddy detectors – something sorely needed as LLMs become more prevalent in society.
YOU MIGHT LIKE
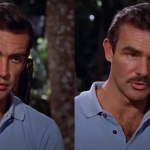