Bringing data science to the boardroom
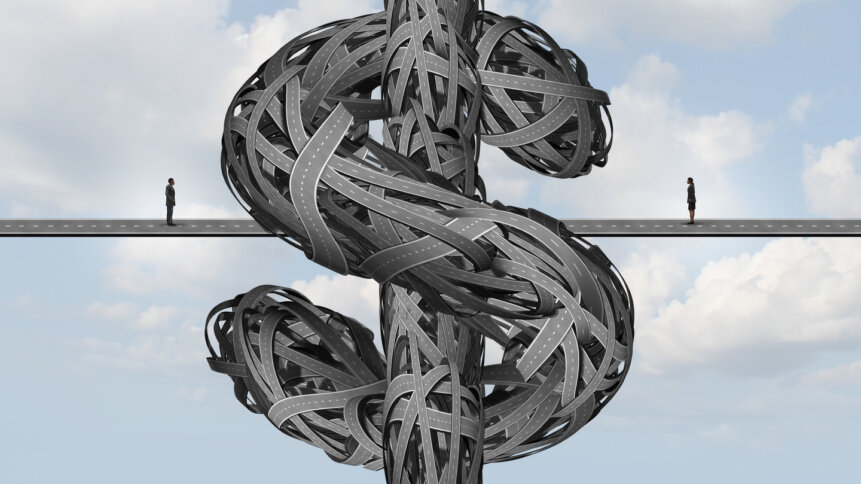
Getting your Trinity Audio player ready...
|
Despite the frequently-listed advantages of data-led projects as presented by in-house data teams (or data insight system vendors), it’s often very difficult for a CEO to justify the required investment for large data mining and business intelligence projects. Business intelligence platforms are many and varied and may promise immediate results, but what exactly can data sources show to the C-Suite (and the CEO particularly) that they don’t already know?
Many CEOs and boards have had their fingers burned in the past, with data scientists promising big but then either under-delivering, taking upward of a year to produce meaningful results, or worst of all, taking many months to produce nothing relevant. Sometimes too, data project leaders claim after the fact that they were “asked the wrong question” by decision-makers at a project’s inception. So why are data projects so slow, costly, and seemingly of so little value?
If we assume that there is a seam of digital gold in the mass of data that most organizations accrue daily, how can we realize better value from it? And more pertinently, how can real value be identified and presented more quickly (think weeks or days, not months or years) without throwing the equivalent of a small country’s GDP at a group of individuals who seem to have little notion of how a business works?
Think about vocabulary & education
Any executive-level decision-maker has had highly specialized training throughout their career. They have been promoted (hopefully) based on their abilities and are rewarded commensurately. They will know, in the case of the CEO, how the organization works and its medium to long-term goals. Specialist C-suite members likewise have shown talent and fortitude to get to where they are, with a good deal of operational experience thrown into the mix in finance, IT, marketing, and so on. Most strategic roles in today’s businesses have existed in one form or another for many decades, if not hundreds of years.
In contrast, data science as a discipline has only existed for a few years. We began to hear about “big data” perhaps ten years ago. The discipline of data processing, and gathering insight from raw information was, until quite recently, an academic exercise practiced at research institutions attached to universities.
Small wonder, then, that there is a mismatch between the language and motivations of the C-suite and a data analyst fresh out of higher education (or, at best, a more experienced IT professional who’s cross-trained in the “new” science of advanced data analytics). It’s a rare data scientist indeed that has proven data analysis and BI “chops,” yet who’s also conversant with how any company can proceed from A to B in terms of its strategy, sales, operations, marketing, and so on. The converse is also true: today’s CEO or CFO might be capable of wrangling complex Excel sheets but unable to build abstract data models.
It’s important, therefore, to cross-educate between business-focused groups and data analytics teams, whether in-house or outsourced. Without common ground, any insight worth drawing from data will be missed. Business Intelligence Platforms (BIPs) purport to help solve the mismatch between business focus and pure data. But there’s also the issue of approach.
Forming continuously collaborative working groups is imperative to this cross-education. Your CIO or CTO can confirm that the best way to produce any new product is by using an Agile methodology, where collaboration between users and software developers (for instance) has to be continuous to keep the project on track. The smart CEO will listen to them.
The same is invariably true when data insights are needed to inform business strategy. Because businesses change so quickly, the agility necessary can only come from dedicated working groups comprising multiple stakeholders working on the same problems as close to full-time as possible. As the team(s) mature, so the time-to-value will decrease, an effect largely down to each stakeholder group becoming more conversant with the requirements of the other(s).
Picking the business intelligence platform
As a relatively new concept, commercial platforms that support business-focused data teams are largely immature. Several platforms from enterprise-scale names such as Oracle and IBM represent many years of development and business/technology crossover. What quickly becomes apparent is that these platforms require extensive alterations to fit the use cases of their users, and such attenuation won’t come cheap.
At the other end of the scale are the many open-source data or business intelligence platforms that can be used as the building blocks to deliver a similarly bespoke solution. It is a well-accepted maxim that at least 60% of every proprietary piece of software available today comprises open-source elements. But building a data analytics and business intelligence solution from the ground up (albeit with as much open-source functionality as possible) is also massively costly. Software developers neither come cheap nor do they (often) come with the necessary specializations in data science. The software developer with experience in R (commonly used in statistical data analysis), Python, and advanced database administration is, like our data scientist-cum-business-professional, a rare beast.
We regularly feature and review data management, analysis, processing, and presentation platforms that are the market leaders in this emerging sector. Here though, we’re highlighting a business’s priorities when choosing an eventual partner (if third-party partnerships are considered appropriate).
Keep it bite-size
Throughout the Data Analysis and Business Intelligence spotlight series, our reporters have spoken to many thought-leaders in this area of next-generation, data-driven business intelligence. Without exception, all these leaders have advised that any data project start small, with very particular outcomes stipulated from the outset. Given that to keep any data science project on the right track, there’s a high degree of every stakeholder “finding their feet,” it’s essential that projects be small and discrete, at least to begin with.
The timescale of any project should also be kept short, partly to dial down expectations with a smaller scope, but also to ensure there is no “mission creep” over a year or more. The cadence of quick time-to-value needs to be instigated as soon as possible and developed as a working ethos for any data project group.
Not-so-great expectations
There tends to be a natural mismatch between the mentalities of a pure-bred data science professional and an MBA-touting CEO. The latter is often guilty of impractical ideas of timescales and, therefore, needs to consider where value can be realized in the medium term, rather than in just a few weeks.
Conversely, the academic nature of many data teams’ backgrounds means that professionals will find and appreciate the beauty of their models and the data itself. While intellectual rigor is (almost) always celebrated, the focus on outcomes must be constant and consistent. Data specialists may wish, for example, to refine models and data transformation routines so that there are negligible outliers in data sets. But like any Agile project, the focus should always be on viable products. Business intelligence platforms may be the common ground where short-term projects come to fruition, and longer-term, data-based startegies begin to appear.
All stakeholders have to be willing to collaborate, and collaboration requires compromise. There will be shifts in emphasis as teams evolve, but for short-term, small-scope projects, setting out the style of working together will need to play an important part of the day-to-day work.