What happens when AI and ML turn rogue? Data poisoning
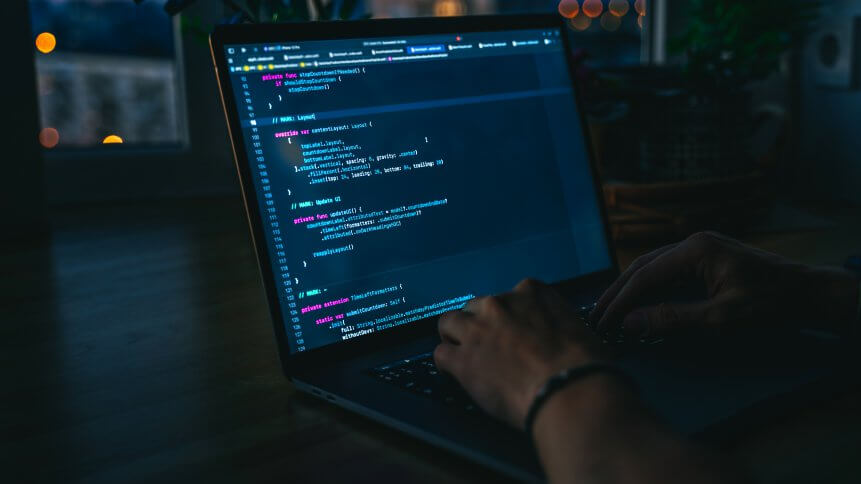
- Data poisoning is an attack method where hackers launch the attack through AI and ML
- The rise of AI and ML is leading directly to the increasing threat of data poisoning
Artificial intelligence (AI) has, for years, been a wide-ranging tool that changes how we integrate information, analyze data, and use the resulting insights to improve decision-making. However, just like how the advent of AI will lead to groundbreaking possibilities in a host of fields, cyber threat actors can also use this technology to wreak havoc.
In fact, more and more organizations are beginning to employ machine learning (ML) and AI as part of their defenses against cyber threats. In a recent report, Bloomberg noted that the combination of AI and cybersecurity is inevitable as both fields sought better tools and new uses for their technology. “But there’s a massive problem that threatens to undermine these efforts and could allow adversaries to bypass digital defenses undetected,” it said.
The problem? Data poisoning — more dangerous than traditional attacks because, instead of attacking from the outside, it attempts to make malicious inputs accepted into the training data, thereby affecting its ability to learn from ‘good’ data and produce accurate predictions. Data poisoning can occur if hackers gain access to a model’s private training data, or relies on user feedback to learn.
The irony is that the very nature of ML, a subset of AI, is the target of data poisoning. Given the vast amount of data one might be dealing with, computers can be trained to categorize information correctly. “Adversarial data poisoning is an effective attack against ML and threatens model integrity by introducing poisoned data into the training dataset,” researchers from Cornell University explain.
Bloomberg highlighted that the same approach is used in cybersecurity. “To catch malicious software, companies feed their systems with data and let the machine learn by itself,” the report noted.
What makes attacks through AI and ML different?
It doesn’t seem extremely difficult to subvert the algorithm as AI & ML only know what humans teach them to learn. In a paper for Harvard University’s Belfer Center for Science and International Affairs, the writer said there are inherent limits and weaknesses in the algorithms that can’t be fixed. So since AI & ML techniques rely heavily on data and certain algorithms to fight cyber threats, it allows for the problems to be identified more efficiently.
But that also makes this a real threat, as attackers can influence the data and to top it off, the rise of AI and ML is leading directly to the sleeper threat of data poisoning. Among the byproducts of data poisoning includes deepfakes which is expected to be the next big wave of digital crime.
YOU MIGHT LIKE
AI for cybersecurity: Friend or foe?
Under the deepfake umbrella are videos, images, and voice recordings that are altered in ways that regular humans can’t identify them, bad actors have started using the techniques for blackmailing, harassment or corporate espionage. Fake news and disinformation can also fall under the category of data poisoning.
More often than not, popular social media algorithms are either technically weak or corrupted to identify fake news. Hence why see the rise of incorrect or misleading information on a user’s news feed, replacing authentic news.
How to stop data poisoning since AI is in its prime time?
While the industry is not blind towards this ominous issue, cyber defense experts are still learning how to defend against data poisoning attacks in the best possible way. Bloomberg noted that one way to help prevent data poisoning is by having scientists who develop AI models regularly check that all the labels in their training data are accurate.
Some cybersecurity experts have also suggested using ‘open’ data with caution. Open-source data can be very appealing because they provide access to even more information to enrich existing sources. In principle, this should make it easier to develop more accurate models, but open data is just that: open. It makes for an easy target for fraudsters and hackers to come after.