Europe needs to hasten AI in manufacturing adoption
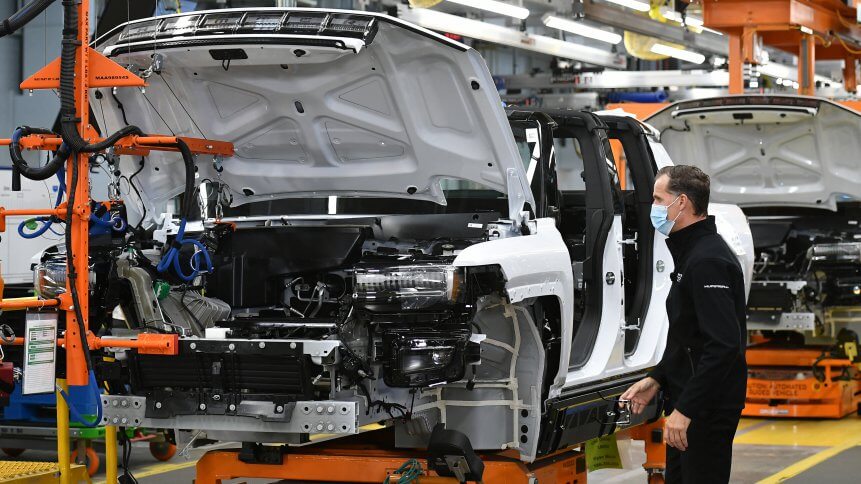
The manufacturing industry relies heavily on modern technologies to improve its production and minimize disruption. Since the COVID-19 pandemic leads to lockdowns in many countries, most manufacturing companies have moved to automate most processes.
When it comes to automating processes in the manufacturing industry, AI is a key technology component in making it happen. Today, AI in manufacturing has a myriad of use cases that can not only help businesses have better businesses continuity and productivity but also enable them to reduce their costs in the long run.
According to McKinsey’s The State of AI report, 57% of organizations in emerging economies reported an increase in AI adoption. The top three use cases are service operations optimization, AI-based enhancements of products, and contact center automation, with the biggest percentage-point increase in the use of AI being in companies’ marketing budget-allocation and spending effectiveness.
Despite this, the adoption of AI in manufacturing is not as high as it should be in some countries. Speaking to Tech HQ, Jonathan Masci, Co-founder and Director of Deep Learning at NNAISENSE, pointed out that AI adoption in manufacturing in some European countries is actually much slower compared to Asia and the US.
“The main difference is the US and China has an AI-first motto compared to some countries in Europe. They are using AI to enable tools for next-gen products or next-gen production. Companies like Tesla are leading in this. They have adopted it early compared to countries like Germany or any other European country,” said Masci.
For companies like NNAISENSE, they aim to bring AI to the physical world and enable AI-driven decision making. AI in manufacturing or industrial AI is all about coming up with the best strategy to optimize production. While most manufacturers want this, Masci said the reality is that 85% of AI projects fail to make it to production.
At the same time, some off-the-shelf AI solutions are growing in popularity among manufacturers aiming to automate factory processes, detect anomalies in production, and digitally transform the manufacturing process. However, these solutions quickly lose their value without proper guidance from experts who understand AI and its applications in individual factories, each with its unique needs. As a result, companies are losing valuable resources investing in technologies that ultimately do not deliver.
For Industrial AI to be most effective, CTOs and technical personnel must truly understand what AI can do, what technical questions need to be asked, and what ways AI can best be utilized to increase factory efficiency and drive company growth. With that said, NNAISENSE’s Industrial AI academy is built around three pillars to enable CTOs and technical teams to succeed in implementing AI into their factories.
The first pillar is built around teaching what Industrial AI actually is, its capabilities, and perhaps most importantly, what makes it different from traditional software implementation. The second pillar is based around teaching CTOs how to determine what kinds of experts and personnel they need to effectively implement AI immediately and at scale, and the third pillar focuses on enabling CTOs and their teams to accurately evaluate different AI options in their industry and pick the best solution for them.
Closing the gap for AI in manufacturing
AI is capable of transforming every industry today. While some industries will have more disruptive AI use cases, Masci pointed out that the most common use case of AI is to do with inspection use cases.
This includes AI in the use of cameras, sensors, and such to monitor, collect and process data. Such processes are repeatable and are also low-cost for implementation. For example, a manufacturing plant that uses IoT devices with sensors that produce data that can be automated.
Apart from that, AI in manufacturing is also not being used for digital twin processes. Digital twin, which is a digital replica of physical infrastructure or process, enables organizations to have a real-time view of their operations. What makes it interesting is that organizations can also use AI enables digital twin tech to look at the what-if scenarios and run simulations on their productions and such.
“With AI, every process is different as it’s a data-driven tech. One does not need complicated AI tech to save money or reduce energy consumption,” added Masci.
In this case, Masci pointed out an example of machines in factories. If a factory has several machines, they will realize that each machine has a different capacity of output and energy utilization. With digital twin tech, the organization may find a way to calibrate the machines and help them reduce their energy cost.
However, Masci also explained that is where the struggle can be for some organizations. The initial cost of having a digital twin and investment in AI may be too high than reducing the energy consumption.
“But what should be acknowledged, and which is not often the case is that if you have digital twin technology, you can optimize the process. And if you have multiple twins, you can connect them, synchronize the machines, and have consistent quality. If you can see this, you will see it as a game-changer. Because the reality is, even though machines are built the same, the output is always different. And if you can calibrate them, you can unlock a new type of business which is actually less energy-consuming,” explained Masci.
YOU MIGHT LIKE
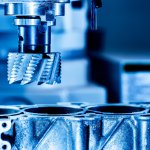
Add AI into the IIoT mix to smarten up manufacturing ops
With that said, Masci is hoping the adoption of AI in manufacturing in Europe may see an improvement as more use cases continue to be developed to improve productivity and reduce costs.
As Masci puts it, AI is the first stepping stone into a game-changing product at the end of the line and it should be done as fast as possible. While most organizations in Europe feel it does not bring value in the short term, it should be viewed as a deal-breaker as the long-term effects of AI will not only bring them better cost savings but also enable them to venture into new horizons.