How can AI derail the organization’s cybersecurity?
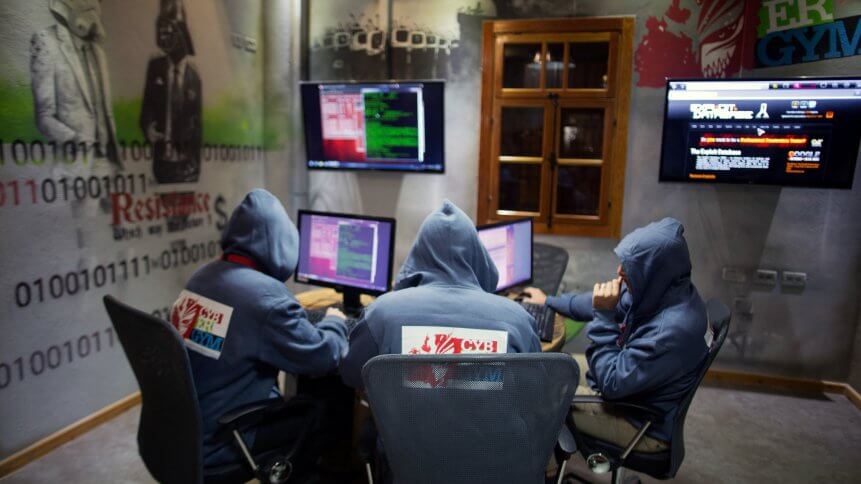
- AI could help to fight cybercrime but it is not a silver bullet, and could also be exploited by malicious hackers
- There is an undercurrent of fear about the consequences if things run amok or attackers learn to make better use of AI
Artificial intelligence (AI) is an umbrella discipline that covers everything related to making machines independently smarter and able to solve problems by themselves. However, when it comes to a heightened period of cyber risks, AI can be a double-edged sword that can be tapped by cybersecurity researchers and cybercriminals alike.
The reality is that AI and its disciplines like machine learning (ML) are playing an increasing role in cybersecurity, with intelligent security tools relying on some type of trusted ‘ground truth’ as reference point to base its assumptions, analyzing data from millions of cyber incidents, and using it to identify potential future threats. But at the same time, the cybersecurity industry is rapidly embracing the notion of ‘zero trust’, where architectures, policies, and processes are guided by the principle that no one and nothing should be trusted, and should be all bound to the same trustless security protocols.
It is first important to know that AI algorithms use training data to learn how to respond to different situations. They learn by replicating and adding additional information as they go along. Essentially, AI models are only as good as the data they consume. But what if everything you invent to defend yourself, can also eventually be used against you?
AI’s potential to automate security on a broader scale offers a welcome advantage in the short term. Yet unleashing a technology designed to eventually take humans out of the equation as much as possible naturally gives the industry some pause. What sort of predicaments could AI-based cybersecurity systems face?
Data poisoning
According to Citi’s Global Cyber Partnership VP Eyal Balicer, bad actors can poison training data by manipulating the datasets (even the pre-trained models) that the AI models are relying upon. This could then allow them to circumvent cybersecurity controls, while the organization at risk remains oblivious to the fact that the ground truth it relies on to secure its infrastructure has been compromised. Such manipulations could lead to subtle deviations, such as security controls labeling malicious activity as benign, or generate a more profound impact by disrupting or disabling the security controls altogether.
Errors by AI in cybersecurity
It is inevitable almost — even staple security-related training datasets are likely laced with inaccuracies and misrepresentations. After all, some of the benchmark datasets for many leading AI algorithms and exploratory data science research have proven to be rife with serious labeling flaws. To top it off, enterprise datasets can become misleading, obsolete, or just error-strewn over time unless the relevant data, and details of its lineage, are kept up-to-date and tied to relevant context.
YOU MIGHT LIKE
Six cybersecurity trends heading our way in 2021
Adversarial AI
As noted in a March 2019 article by MIT Technology Review, University of California professor and cybersecurity researcher Dawn Song stated that adversarial machine learning could be used to attack just about any system built on the technology. Song’s research group explored several examples of how adversarial learning can be used. For instance, in one case they demonstrated how attackers could exploit machine learning algorithms designed to automate email responses to instead “spit out sensitive data such as credit card numbers.”
Researchers at Princeton also explored how adversarial tactics applied to artificial intelligence could leave systems vulnerable. In the report, the researchers noted, “Just as software is prone to being hacked and infected by computer viruses, or its users targeted by scammers through phishing and other security-breaching ploys, AI-powered applications have their own vulnerabilities. Yet the deployment of adequate safeguards has lagged.”
Another example of adversarial attacks is the concept of deep fakes. As reported by the Financial Times, AI-powered deep fakes are already being used in everyday attacks such as fraud, as well as to manipulate videos. That being said, adversarial attacks can take several forms, including false flag attacks. By manipulating data, attackers can launch cyberattacks and make them appear to come from a specific country.