Google uses AI to design next-gen chips faster than humans
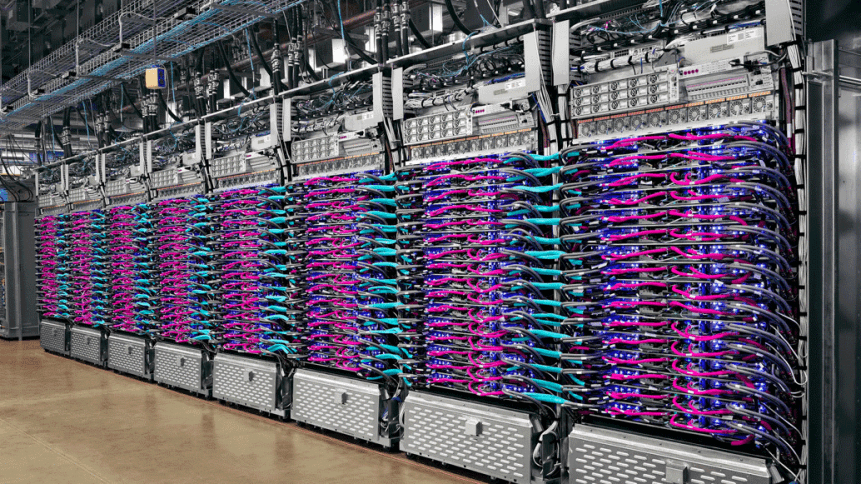
- Google is developing chips designed by AI, harnessing machine learning
- The AI has already been used to develop the latest iteration of Google’s tensor processing unit chips
- The tech behemoth’s engineers noted in the paper that the breakthrough could have “major implications” for the semiconductor sector
Working out where to place the billions of intricate components that a modern computer chip needs can take human designers months and, despite decades of research, has defied automation. Recently though, a team from Google revealed a new machine learning algorithm that develops AI software that designs computer chips faster than humans can.
The tech giant said in a paper in the journal Nature that a chip that would take humans months to design can be drawn up by its new AI in less than six hours. The AI has already been used to develop the next iteration of Google’s tensor processing unit chips, which are used to run AI-related tasks, Google said. The authors of the paper, led by Google’s co-heads of machine learning for systems, Azalia Mirhoseini and Anna Goldie, said, “Our method has been used in production to design the next generation of Google TPU.” To put it another way, Google is using AI to design chips that can be used to create even more sophisticated AI systems.
What does this latest AI by Google does exactly?
Google has been working on how to use machine learning to create chips for years, but this recent effort seems to be the first time its research has been applied to a commercial product: an upcoming version of Google’s own tensor processing unit (TPU) chips, which are optimized for AI computation.
To be precise, Google’s new AI can draw up a chip’s “floorplan”. This essentially involves plotting where components like CPUs, GPUs, and memory are placed on the silicon die in relation to one another — their positioning on these minuscule boards is important as it affects the chip’s power consumption and processing speed. To optimally design these floorplans usually takes humans months to optimally design but Google’s deep reinforcement learning system — an algorithm that’s trained to take certain actions in order to maximize its chance of earning a reward — can do it with relatively little effort.
Google’s engineers trained a reinforcement learning algorithm on a dataset of 10,000 chip floor plans of varying quality, some of which had been randomly generated. Each design was tagged with a specific “reward” function based on its success across different metrics like the length of wire required and power usage. The algorithm then used this data to distinguish between good and bad floor plans and generate its own designs in turn.
In the paper, Google’s engineers note that this work has “major implications” for the chip industry. It should allow companies to more quickly explore the possible architecture space for upcoming designs and more easily customize chips for specific workloads.
An editorial in Nature calls the research an “important achievement,” and notes that such work could help offset the forecasted end of Moore’s Law — an axiom of chip design from the 1970s that states that the number of transistors on a chip doubles every two years. AI won’t necessarily solve the physical challenges of squeezing more and more transistors onto chips, but it could help find other paths to increasing performance at the same rate.
Google itself has explored using AI in other parts of the process like “architecture exploration,” and rivals like Nvidia are looking into other methods to speed up the workflow. The virtuous cycle of AI designing chips for AI looks like it’s only just getting started. In fact, Google’s new floor planning strategy could also help tackle other problems that involve looking for the best uses of limited sets of resources, such as city planning or vaccine testing and distribution.