Why black box AI problem is bad for business
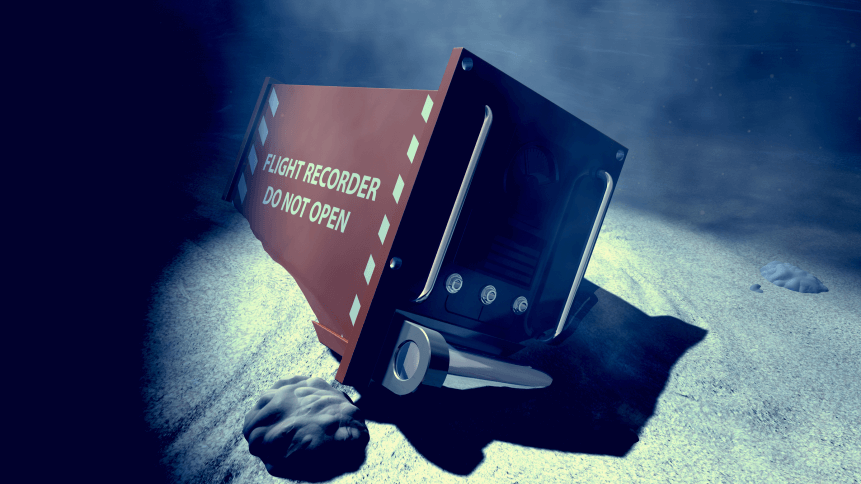
Deep learning algorithms take millions of data points as inputs, correlating specific features to produce an output.
While humans are involved in the initial management of data, such as data labeling, once fed into a system the process is largely self-directed.
Even for the data scientists and programs involved in the model’s development, it can be difficult to interpret and subsequently explain how a process has led to a specific output.
This is a complex issue earning the label ‘black box AI’ — and it is becoming a greater problem as artificial intelligence (AI) and machine learning plays a bigger role in our day-to-day and working lives.
When the workings of software used for important operations and processes within a business cannot be easily viewed or understood, errors and bias can go unnoticed and snowball into much bigger, potentially irreparable, problems.
Put in the context of AI’s use today, whether that’s the shortlisting and hiring of candidates for roles or loan decisions by banks, it’s clear to see why black box AI poses a problem.
Last year, the Information Commissioner’s Office (ICO) put forward regulations that would require businesses and other organizations are required to explain decisions made by artificial intelligence.
The group urged organizations using AI to produce documentation explaining the mechanics of models in use, as the use of the technology becomes rapidly widespread.
Organizations that cannot explain how an automated decision was made could even be in breach of the General Data Protection Regulation (GDPR), putting them at risk of potentially multi-million-dollar fines, depending on annual revenue.
Workers’ rights
But these guidelines haven’t necessarily done enough — to contest a decision made by an algorithm, an individual or organization would first have to know AI was behind the decision made. But disclosure is lacking.
In the UK, trade unions are now forming an AI task-force to lobby employers and regulators to increase transparency around where the technology is being deployed, and to offer workers recourse if they believe they are being discriminated against.
The initiative is being driven by the Trades Union Congress (TUC), an umbrella group for unions representing more than 5.5 million people.
“We realized that essentially there’s a revolution taking place in the world of work in terms of the use of AI to manage people,” Mary Towers, an employment rights policy officer at the TUC who’s leading the effort, told Bloomberg.
“What we’re really talking about is the use of AI to make decisions that really impact significantly on people’s job opportunities and the nature and fabric of their working lives.”
Employees speaking to the TUC said they are encountering AI in job applications and interviews, in shift and vacation management decisions, and performance analysis — areas where AI errors could result in unfair outcomes or discrimination.
YOU MIGHT LIKE
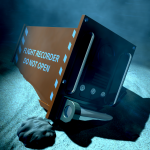
How much should we trust explainable AI?
The TUC said that a lack of transparency is compounding the problem. Just 14% of employees thought they would know if AI had made an automated decision about a job application, according to the UK’s Centre for Data Ethics.
It’s not just employees that are lacking trust in AI. Concerns over the ability to trust AI-enabled decisions could hold back the adoption of the technology more broadly, limiting or delaying its further advance and wider benefits.
The solution, according to the TUC, starts with unions finding ways to negotiate increasing transparency on where AI is being used, and control over the data that’s collected and used by algorithms.
AI is a powerful tool, but if we’re not able to show its workings, gaining acceptance will be difficult.
Last year, Google launched Explainable AI on its cloud platform, a set of tools and frameworks for developing machine learning models that allow developers to “visually investigate model behavior” and “detect and resolve bias, drift, and other gaps” in machine learning models.
“Machine learning models can identify intricate correlations between enormous numbers of data points,” said the firm’s Cloud AI Director of Strategy, Tracey Frey in a blog post; “While this capability allows AI models to reach incredible accuracy, inspecting the structure or weights of a model often tells you little about a model’s behavior.
“This means that for some decision-makers, particularly those in industries where confidence is critical, the benefits of AI can be out of reach without interpretability.”