Is this a breakthrough for green AI?
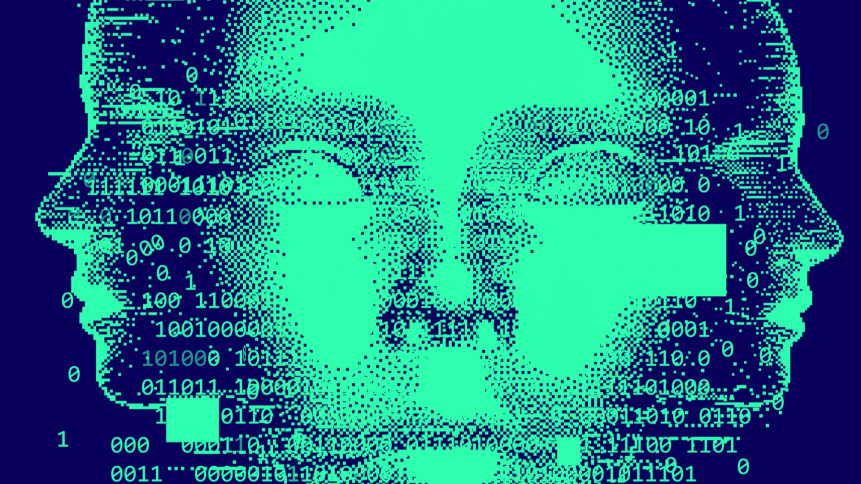
- The mathematical breakthrough helps AI applications like speech recognition, gesture recognition, and ECG classification to become a hundred to a thousand times more energy efficient
For all of its power and promise, artificial intelligence has some big drawbacks — its massive carbon footprint is one of them.
Training a ‘regular’ AI using a single high-performance graphics card produces the same amount of carbon as a flight across the United States, according to MIT Technology Review. That’s because AI requires so much data. All of it must be captured, stored, analyzed, and sent out, and this requires vast amounts of processing power. Data centers require more servers, larger footprints, and cooling.
As the tech industry continues to advance in applications of AI, and consumers and enterprise take for granted the results in better products and services, there is growing pressure to address and tackle AI’s environmental impact.
Energy-efficient AI
In the hopes of reducing that damage, researchers at the Centrum Wiskunde & Informatica (CWI), the Dutch national research center for mathematics and computer science, and IMEC/Holst Research Center from Eindhoven in the Netherlands, have successfully developed a learning algorithm for spiking neural networks (SNNs).
The mathematical breakthrough published in a paper catchily entitled ‘Effective and Efficient Computation with Multiple-Timescale Spiking Recurrent Neural Network’, helps AI applications like speech recognition, gesture recognition, and ECG classification to become up to a thousand times more energy efficient.
These breakthroughs, said researcher and professor of cognitive neurobiology, Sander Bohté, make AI algorithms “a thousand times more energy efficient in comparison with standard neural networks, and a factor hundred more energy efficient than current state-of-the-art neural networks.”
Brain-inspired AI
SNNs are artificial neural networks that more closely mimic natural neural networks or the way the human brain processes information. In the past, computers have imitated the brain’s neuronal networks to produce applications like image recognition, speech recognition, automatic translation, to medical diagnoses. But, so far, they have lacked in efficiency, and have required up to a million times more energy than the human brain.
SNNs require a lot less frequency in communication and involve minimum calculations for performing a task.
“The communication between neurons in classical neural networks is continuous and easy to handle from a mathematical perspective. Spiking neurons look more like the human brain and communicate only sparingly and with short pulses. This, however, means that the signals are discontinuous and much more difficult to handle mathematically,” added Bohté.
YOU MIGHT LIKE

How tech companies are moving into the energy sector
As of today, Bohté’s methods are capable of training spiking neural networks comprised of up to a few thousand neurons. Typically less than classical neural networks, the new method is still sufficient for many applications like speech recognition, ECG classification, and the recognition of gestures. The next challenge facing Bohté and his team of researchers will be to further expand the application possibilities and scale up these networks to one hundred thousand or a million neurons.
The underlying mathematical algorithms have been made available open-source, while prototypes for the new types of chips necessary to run spiking neural networks are already in development.
The environmental impact of AI and data is no secret. The latest breakthrough by researchers looks set to bring about more efficient tools readily available and primed for further development. At the same time, this alternative could enable the running of applications on small AI devices like chips or smartwatches with less need for cloud intervention.