Data labelling — overcoming AI projects’ biggest obstacle
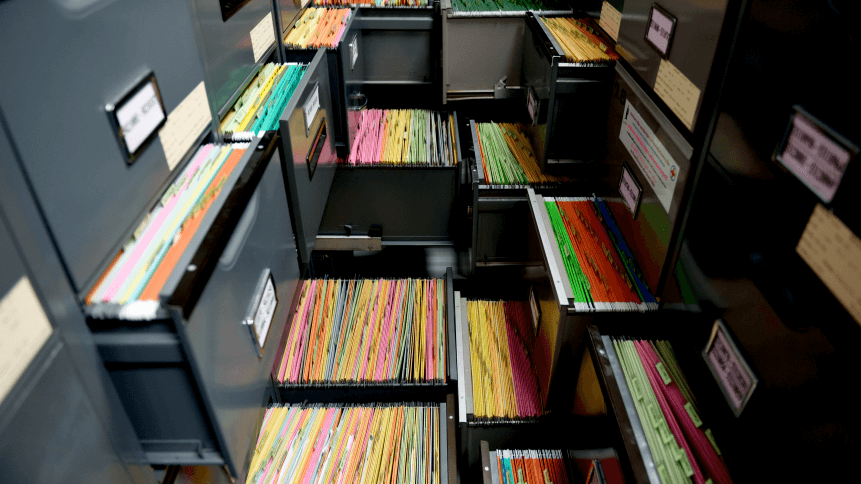
- Data labelling might not be sexy, but it’s crucial and necessary AI gruntwork
- It can be a tough task in house, while a new ecosystem of data labelling startups emerges to meet demand
Building artificial intelligence (AI) models is not like building software. It requires a constant ‘test and learn’ approach. Algorithms are continually learning and data is being refined — and as much relevant, high-quality data as possible is key.
Data labelling is an integral part of data pre-processing for machine learning. If you’re training a system to identify animals in images, for example, you might provide it with thousands of images of various animals from which to learn the common features of each, which would eventually enable it to identify animals in unlabelled images.
In autonomous vehicle systems, data labelling is used to enable the car’s AI system to tell the difference between a person crossing the street or a paper bag, for example, labeling the key features of those objects or datapoints and looking for similarities between them. In voice recognition, machines need accompanying text transcripts alongside audio as a basis for learning.
The data labelling ‘obstacle’
While data labelling is a fundamental component in training AI systems, however, it’s something that nearly every organization (96%) faces challenges with when it comes to AI implementation and production. That’s according to a report released from Alegion, which ultimately found that while there is huge interest in AI and machine learning projects, just half had successfully got AI/ML projects into production, while 81% said the process of training AI with data had been more difficult than expected.
“The single largest obstacle to implementing machine learning models into production is the volume and quality of the training data,” said Nathaniel Gates, CEO, and co-founder of Alegion in a press release.
It’s essential that training data is not only extensive but also annotated accurately and correctly. The vast majority of that work is left to humans who — while they can identify the cat in the picture or even a tumor in an x-ray — are expensive, slow, and prone to error. As such, the increasing demand for automation and AI applications has created a surge in interest for advanced data labeling tools and services that can hasten and augment the process, ultimately getting projects deployed all the more quickly.
In the last several years, a data labelling industry has emerged in answer to demand for solutions and services that help remove the “obstacle” of this work on organizations hoping to develop an AI model, or to analyze data once it’s been labelled for blindspots and biases.
Some organizations have turned to crowdsourcing; Refunite, for example, developed an app that allows those who have been uprooted by conflict to earn money by ‘training’ AI algorithms from their smartphones.
An entire ecosystem of tech startups has emerged that can contribute to the data labelling process — for a fee. In many cases, AI programs are used in this proccess too. Arturo.ai, for example — a spin out from American Family Insurance — specializes in machine learning software to analyze photography and satellite images for the insurance industry. San Diego startup Lytx sells systems for trucking businesses to access drivers’ behavior through cameras and sensors data, and claims to take about 10,000 hours of labelled 20-second video clips — or 4 to 5 million hours of video — to train a prototype AI system that can identify something like driver distrations.
Other firms, such as Scale AI and Labelbox, provide tools to help companies analyze data once it’s labelled, allowing them to identify blindspots and biases. That could be an over representation of men for example, or too few images of something.
Tel Aviv-based SaaS startup Dataloop, meanwhile, combines human and artificial intelligence for training computer vision programs. The platform feeds ‘real-time’ data back to a human user, to ensure the process — while expedited — is still performed at a high standard. It also means certain datasets may already be available from previous projects, so businesses don’t have to start the data labelling process from scratch. “Many organizations continue to struggle with moving their AI and ML projects into production as a result of data labeling limitations and a lack of real-time validation that can only be achieved with human input into the system,” said Dataloop CEO Eran Shlomo.
The demands of data labelling will continue to pose an inconvenient truth to the development process of AI programs, and whether organizations decides to take the work on in-house, crowdsource, or outsource, the quality and effectiveness of the end product will hinge on the preparation.