5 ways machine learning is transforming IT monitoring in 2020
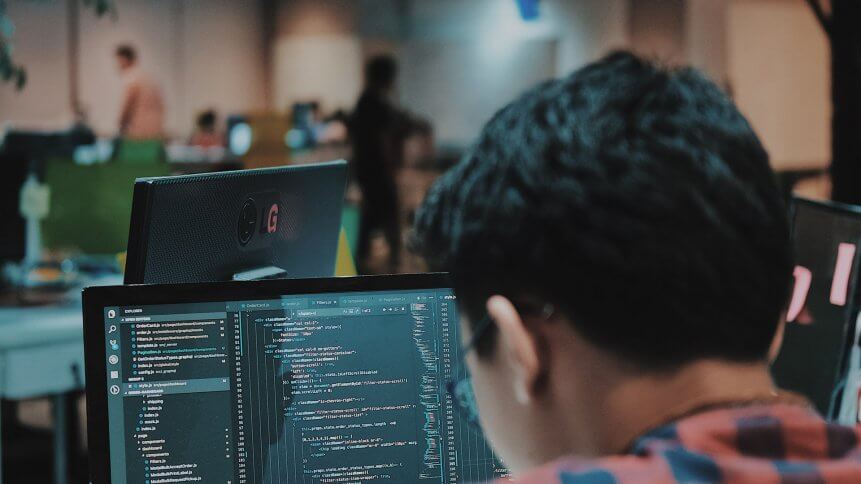
As IT infrastructures become increasingly complex, it has become imperative for IT leaders to create new monitoring procedures that grow in line with their organization.
IT monitoring comprises a broad range of products, to let analysts determine whether IT equipment is performing to expected service levels, while also managing any problems detected. This can range from basic checks to the use of advanced tools like machine learning (ML).
As the pace of change in the industry accelerates, it has been a critical requirement for IT operations to support an always-on business to fill the expertise gap and allow customers to focus on their business.
The challenge facing IT monitoring teams is the tendency to use legacy practices that need to be actively running constantly. This puts teams at a significant disadvantage and leaves them to sift through unnecessary noise and bundles of missing information. What if the workability of these systems were optimized?
Artificial intelligence (AI) and ML have so far been vital in taking pressure off of internal processes.
The race to take advantage of AI and ML has partly come from the need to implement “data-first” thinking when building core systems, while also partly being attributable to the cross-industry leap to the cloud.
In an era of COVID-19 where businesses have been scurrying to utilize the power of AI-driven tools, more organizations are creating roadmaps that reflect the need to shift strategy.
Machine learning in IT monitoring
# 1 | Tailoring alerts
Honing in on a known pain point within traditional anomaly detection systems, by using a combination of supervised and unsupervised machine learning algorithms, you can decrease the signal-to-noise ratio of alerts and correlating those alerts across a variety of toolsets in real-time. In addition, algorithms can be used to capture remediation behavior to recommend remediation steps when future incidents occur.
# 2 | Collating metrics
Through advanced anomaly detection systems based on machine learning algorithms, you can identify correlations between sets of metrics sent from different data sources within your infrastructure and applications. What’s more, some ML platforms offer unique cost optimizations reports that can compare instance utilization with AWS spend.
# 3 | Business intelligence
Through real-time analytics and automated anomaly detection systems, distinguished outliers can be discovered within vast amounts of data to turn them into valuable business insights.
Machine learning logic can be applied to metrics obtained from multiple sources to perform automated anomaly detection, before crunching the data to flag anomalies which can then be scored to determine how much of an anomaly the event actually is.
# 4 | Natural language processing
Through the use of natural language processing, semantics, clustering, and topology algorithms, machine learning can help to distill millions of events down to a manageable set of insights. Similar to the above solutions, the use of these algorithms helps to reduce triggered events and alerts to allow for more efficient use of resources and faster issue resolution.
# 5 | Cognitive insights
Alternative use of machine learning for IT monitoring is to combine it with crowdsourcing to sift through vast amounts of log data to identify events. This helps focus on how actual humans are interacting with the data, instead of focusing on mathematical analysis. The approach has been termed as cognitive insights and signifies critical events that may be taking place that needs to be looked at.
While the application of machine learning is by no means straight forward, its potential to transform IT monitoring is clear to see. As IT infrastructures continue to grow, it is already apparent how the industry is increasingly looking towards ML to find cost-efficient and effective solutions today and in the future.