Is this a breakthrough for safety-critical ML?
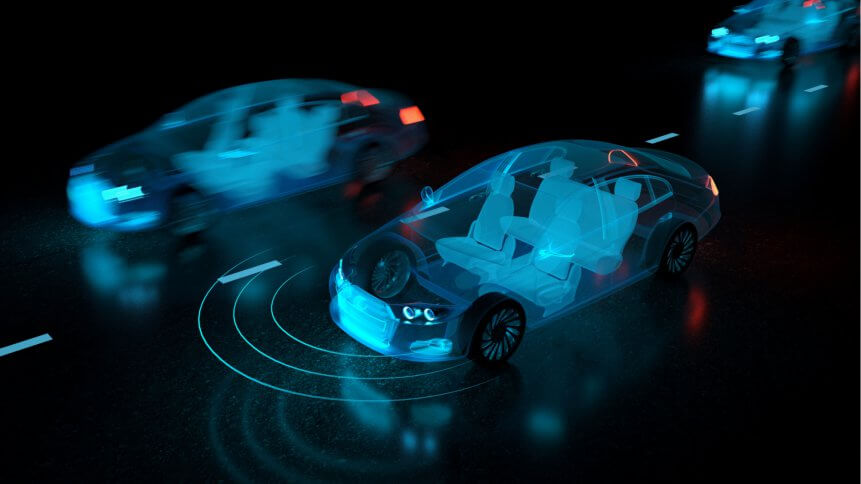
- Researchers from top US colleges have devised a method for predicting failure rates of safety-critical machine learning systems
- The neural bridge sampling method provides the ability to assess risks associated with deploying complex machine learning systems in safety-critical environments
- The breakthrough could lead the way in developing a framework suitable for safe implementation in high-risk environments
Artificial intelligence (AI) is coming at us fast. It’s being used in the apps and services we plug into daily without us really noticing, whether it’s a personalized ad on Facebook, or Google recommending how you sign off your email. If these applications fail, it may result in some irritation to the user in the worst case. But we are increasingly entrusting AI and machine learning to safety-critical applications, where system failure results in a lot more than a slight UX issue.
One of the most significant examples of this is in autonomous vehicles, where the safety of AI systems is paramount to the technology’s adoption and acceptance in society.
In 2018, Elaine Herzber was hit and killed by an Uber self-driving car on a pilot test when the backup driver (who has this week been charged) failed to intervene. A Tesla vehicle’s fatal crash in California on March 23 of this year raised a further cloud over the safety and readiness of self-driving technology.
Safety-critical machine learning systems are occupying wider roles in anything from robotic surgery, pacemakers and autonomous flight systems. Any kind of failure in these cases could lead to injury, death or, perhaps at best, serious financial or reputational damage.
According to researchers from MIT, Stanford University, and the University of Pennsylvania, this could all be about to change.
A breakthrough
In a recent paper titled Neural Bridge Sampling for Evaluating Safety-Critical Autonomous Systems, published on arXiv, the neural bridge sampling method specified in the paper draws on decades-old statistical techniques and builds upon a simulation testing framework that evaluates black box autonomous vehicle systems.
The authors claim neural bridge sampling can give regulators, academics and industry experts a common reference for discussing risks associated with certain safety-critical machine learning systems.
The approach can reassure the public that a system has been tested rigorously, while enabling organizations to keep the workings of the technology a competitive secret, a factor which has hampered investigation into models previously.
“They don’t want to tell you what’s inside the black box, so we need to be able to look at these systems from afar without sort of dissecting them,” co-lead author Matthew O’Kelly told VentureBeat.
“And so one of the benefits of the methods that we’re proposing is that essentially somebody can send you a scrambled description of that generated model, give you a bunch of distributions, and you draw from them, then send back the search space and the scores.”
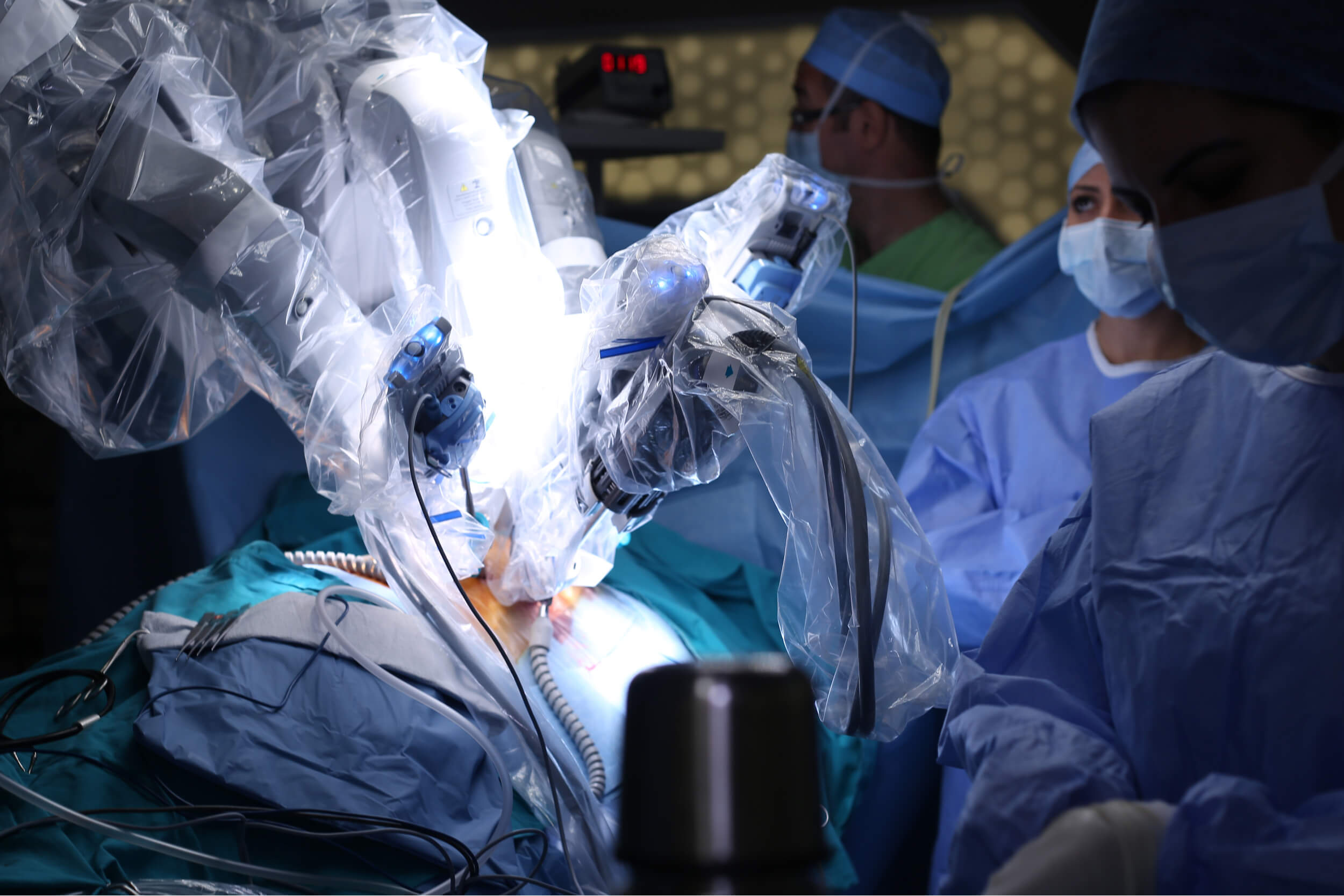
The stakes of ML failure are dire in some applications. Source: Shutterstock
Many safety-critical systems have such low failure rates that they can be difficult to compute, and failure rates are harder to estimate, particularly as the machine learning models develop. Researchers use a scheme to identify areas in a distribution believed to be close to failure.
“Then you continue this process and you build what we call this ladder toward the failure regions,” co-lead author Aman Sinha told VB.
“You keep getting worse and worse and worse as you play against the Tesla autopilot algorithm or the pacemaker algorithm to keep pushing it toward the failures that are worse and worse.”
In addition to the neural bridge breakthrough, its authors favored continued advances in privacy-conscious tech, which touches upon the public backlash over AI and privacy, as well as future concerns that may arise in line with these developments.
“We would like to see more statistics-driven, science-driven initiatives in terms of regulation and policy around things like self-driving vehicles”, said O’Kelly.
“We think that it’s just such a novel technology that information is going to need to flow pretty rapidly from the academic community to the businesses making the objects to the government that’s going to be responsible for regulating them.”
YOU MIGHT LIKE
Autonomous vehicles – why safety takes the front seat
More success
While the application of AI in safety-critical systems has had its setbacks when used incorrectly, there have been notable instances where AI has been heralded a success.
In health care settings, drug discovery and radiology have made important steps towards making AI a useful tool in aiding diagnostic applications.
AI in drug discovery has illuminated the productivity of medical facilities and helped boost care facilities significantly, and is reported to have a global market value in 2020 of US$343.78 million.
What’s more, the growing cost of bringing a drug into the market means that just a minimum of 10% improvement in the prediction of accuracy can save billions of dollars invested in drug development for AI in drug discovery vendors.
Elsewhere, the clinical application areas of artificial intelligence in oncology are far-reaching, with radiology based examples including thoracic imaging, abdominal and pelvic imaging, colonoscopy, mammography, brain imaging, and radiation oncology.
The primary driver behind the emergence of AI in medical imaging is said to be down to the desire for greater efficacy and efficiency in clinical care.
Most applications of safety-critical machine learning are still related to low consequence scenarios, however, developments such as the neural bridge sampling method can help eliminate risk significantly and provide a suitable framework for further safe implementation in high-risk environments, thus raising the standards for what could be possible.