Where explainable AI will be crucial in industry
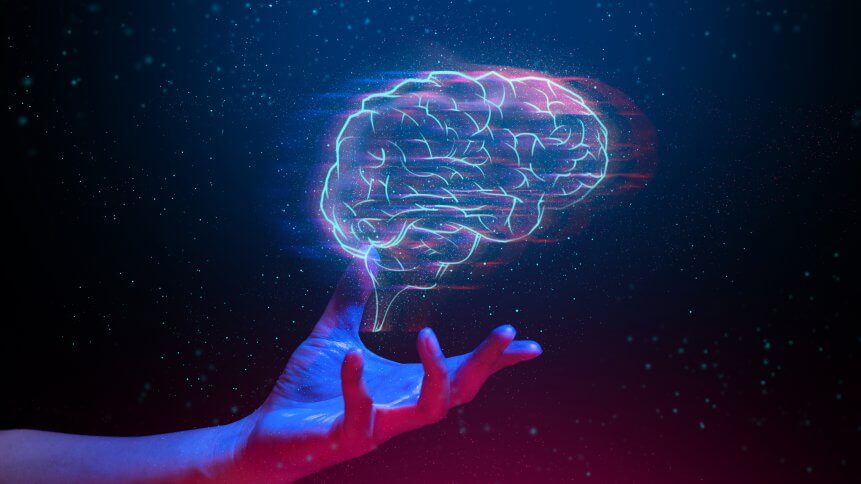
- Explainable AI is set to have a major impact on healthcare, banking, manufacturing, and automobile
- Alleged bias in AI models pushes for more transparency and accountability in AI-based decisions
- UK regulators have imposed guidance for businesses to employ explainable AI
As artificial intelligence (AI) matures and new applications boom amid a transition to Industry 4.0, we are beginning to accept that machines can help us make decisions more effectively and efficiently. But, at present, we don’t always have a clear insight into how or why a model made those decisions – this is ‘blackbox AI’.
In light of alleged bias in AI models in applications across recruitment, loan decisions, and healthcare applications, the ability to effectively explain the workings of decisions made by AI model has become imperative for the technology’s further development and adoption. In December last year, the UK’s Information Commissioner’s Office (ICO) began moving to ensure businesses and other organizations are required to explain decisions made by AI by law, or face multimillion-dollar fines if unable.
Explainable AI is the concept of being able to describe the procedures, services, and outcomes delivered or assisted by AI when that information is required, such as in the case of accusations of bias. It comprises both the decision-making process and the data used to arrive at a decision.
“People are trying to understand how you can make sense of what neural network models come up with – how did they get to their answers? For many regulated industries, such as finance and healthcare, this is a big hurdle to deep learning adoption,” Björn Brinne, Chief AI Officer at Peltarion, previously told TechHQ.
If you were to apply for a mortgage or a loan at a bank and were refused, you’d want to know why, Brinne explained. If the bank had used deep learning, however, they may not be able to explain why the decision was made; “[…] you will need to have some form of ‘explainability’ to understand how the model uses the data to then provide the predictions.”
As AI gains more influence and uptake within the world, ensuring systems are penetrable will be a crucial in making AI ethical, ensuring trust among organizations, individuals and society at large. Let’s take a look at how explainable AI will impact some of the biggest industries in the immediate future:
# 1 | Healthcare
The healthcare industry is set to hit US$11.09 trillion by 2022, with streams of electronic health records (EHRs) contributing greatly to that figure.
From sifting through and organizing medical records; analyzing tests, X-Rays, CT scans; drug discovery and treatment plans, AI has far-reaching applications in the healthcare sector. Earlier this year, scholars from Google Health and DeepMind, along with London’s Imperial College, found that, in some cases AI algorithms were able to outperform human radiologists in reading a mammogram.
But dealing with highly-sensitive data, and contributing to potentially life-changing decisions, complete transparency into decision making processes is required. Explainable AI will be key to allowing the industry to manage, organize, and analyze its colossal datasets, and eventually guide medical professionals into understanding how these AI-based conclusions are made, which may lead to more informed human decision making as a result.
# 2 | Banking
AI systems are playing a rapidly-growing role in the banking sector, from things like daily operations like customer acquisition, KYC checks, customer services to highly sophisticated procedures like approving – or rejecting – applications for mortgage loans.
In such a heavily-regulated industry, the decisions for such high-stakes procedures will require condensed and clear reasoning, including what data models were used and the basis of predictions that formed the AI-backed conclusions.
Last year, the Bank of England’s working paper series explored the need to access the inner workings of AI and ML models in finance. The authors highlighted the need to address a ‘black box’ problem found in AI/ML applications and proposed to design a framework. “Our main contribution is to develop a systematic analytical framework that could be used for approaching explainability questions in real-world financial applications,” the report stated.
# 3 | Manufacturing
In manufacturing, separate companies often rely on what’s referred to as “tribal knowledge” – unwritten information that is not always known by others in the same company. When it comes to decision making, explainable AI offers a set of consistent and debatable solutions for fixing and maintaining equipment.
Since tribal knowledge is almost like a disparate, collective wisdom within an organization, technicians moving in and out means the ‘right way to do things’ isn’t always consistent. With natural language processing (NLP), unstructured data, including manuals, maintenance logs, and equipment details, alongside other structured data such as sensor readings and historical work orders, an AI system can come up with informed guidance for technicians to follow. Explainable AI can provide insight the level of confidence there is in those decisions, why it was prioritized, and allow the user to choose other possible, better choices which can enable the machine to improve its performance.
# 4 | Automotive
For the emerging autonomous vehicle industry, which operates using vast amounts of data generated every millisecond, explainable AI will be critical part of development, particularly in regard to safety.
While the technology is predicted to reduce road deaths significantly, even autonomous vehicles’ highly-advanced sensors and cameras won’t put an end to accidents. Technologists, authorities and insurance companies alike will need complete transparency into the decisions made by AI systems in the run up to any incident, and this will help their further development.
Taking into account the versatile role of AI in high-stake decision-making scenarios, accountability will be imperative for business leaders to attest, support, and go forward with AI-powered decisions. Adding on transparency in decision making will essentially teach us about the mechanics and logic behind AI.