How Bosch is trying to solve ‘low tech’ autonomous vehicle threats
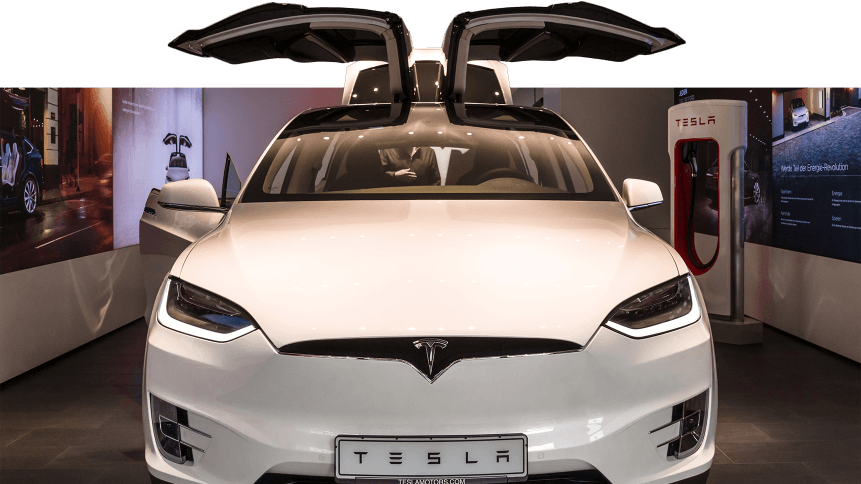
As the age of connected, AI-powered vehicles hurtles towards us, the biggest challenge to achieving full autonomy is endowing systems with the ability to handle the unexpected.
In 2016, Tesla made headlines with one of its vehicles involved in the first fatal self-driving car crash. The driver of the Model S was killed when the car’s autopilot system was unable to recognize a white 18-wheeler crossing the road ahead against a bright sky.
Since then, safety has remained the focus of autonomous vehicle technology development, and, ultimately, the deciding factor of when fully autonomous vehicles can be deployed on our roads— the ability of cameras, sensors and software to react to the unanticipated is therefore absolutely vital.
One of the key ways in which self-driving cars will operate is based on the visual interpretation of surrounding objects, such as road signs, which owed to standardization, are well-suited to machine-learning processes that can identify images.
However, these signs can also be easily defaced to intentionally trick self-driving systems to misinterpret them; “With a few strategically placed pieces of tape, a person can trick an algorithm into viewing a stop sign as if it were a 45 mile-per-hour speed limit sign,” noted a report Wall Street Journal.
While no incidents of this type have taken place yet, it represents a possible new avenue for exploitation as autonomous vehicles become a commonplace tool in society and business. Ultimately, as AI replaces decision-making processes previously made by humans, the very data programs work off itself can compromise operations.
This type of threat has already been subject to experimentation by ‘hackers’. By placing interference stickers on a road, researchers from Tencent have been able to interfere with Tesla autopilot lane assist program, ultimately manipulating the vehicle to make abnormal decisions, including repositioning itself in the reverse lane.
More innocently, the same hackers were able to make a Tesla’s windscreen wipers switch on by displaying a TV playing an image of dripping water in front of it.
The threat of visual manipulation is a focal point of work by German engineering and technology firm Bosch which develops autonomous vehicle technology, including sensors and cameras for traffic-sign recognition.
As reported by WSJ, according to the firm’s CTO, Michael Bolle, rather than pulling back on the AI that can potentially be duped by deliberately altered inputs, such as manipulated road signs, the answer is to ‘double down’ on the technology with AI-driven ‘countermeasures’.
The firm is researching a computer vision process that works in parallel with existing systems, where AI algorithms seek to emulate human visual-processing systems. The method involves analyzing an object from two different perspectives and then compare them against each other.
While one deep-learning system can be used to identify the meaning of a road sign and act on it accordingly, a second system— utilizing computer vision— can be used to analyze the same information differently, acting as a ‘double-check’ on the information the entire system is working form. If there is a discrepancy between the two results, it can signal that somebody is aiming to spoof the system.
This countermeasure approach is based on this type of attack being design to target a particular element of an autonomous vehicle, such as a trained neural network, while another separate element (computer vision) can cross-check the interpretation for anomalies.
The research by Bosch represents the ongoing work by members of the autonomous vehicle industry to cover all bases in vehicle safety and security, but it also highlights that as we become more reliant and trusting of AI algorithms to make important decisions, some of the most severe threats could be those that are low-tech.
“The hacking methods in question reflect on a new kind of cyberattack, in which hackers compromise the information that is fed into an algorithm. This low-tech form of hacking differs from traditional methods of attack, such as penetrating complex information-technology systems,” WSJ reported.
Darren Shou, Head of Technology at software company NortonLifeLock Inc, told the publication; “When we talk about cybersecurity, we talk about hackers who come in our systems and change code and harm our systems.
“In the area of machine learning and AI, products and machines learn from data, and so the data itself can be part of the attack.”