UK data regulator urges business towards explainable AI
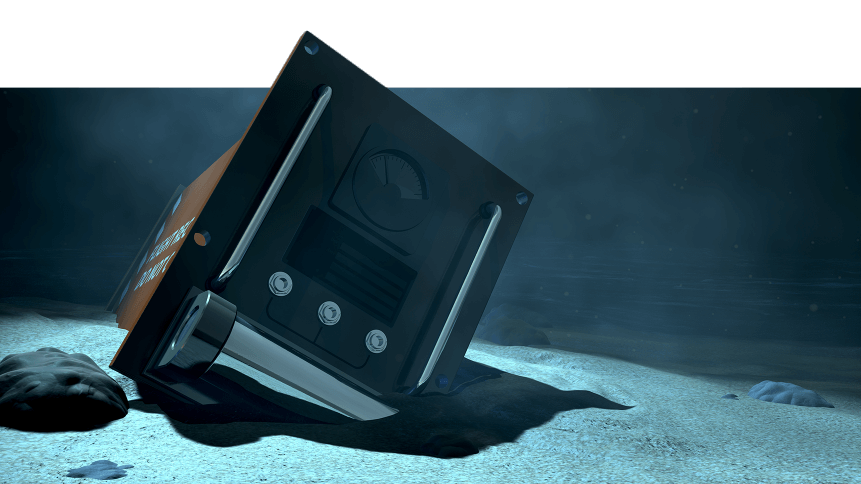
The Information Commissioner’s Office (ICO) is putting forward a regulation that businesses and other organizations are required to explain decisions made by artificial intelligence (AI) or face multimillion-dollar fines if unable to.
The guidance will provide advice such as how to explain the procedures, services, and outcomes delivered or assisted by AI to affected individuals. The report would detail the documentation of the decision-making process and data used to arrive at a decision.
In extreme cases, organizations that fail to comply may face a fine of up to 4 percent of a company’s global turnover, under the EU’s data protection law.
The new guidance is crucial as many firms in the UK are using some form of AI to execute critical business decisions, such as shortlisting and hiring candidates for roles.
At the same time, two-thirds of UK financial services rely on AI to support customer services, drive business decisions and transactions. The Department for Work and Pensions (DWP) is also exploring the potentials of AI to assist in assessing benefit claims.
The greater adoption of AI across sectors has raised concerns among the public on the power and influence of algorithms in decision making. AI researchers are progressively decoding ‘black-box’ machine learning and are going forward to an era where “supervised” learning is the reality and its outcomes are explainable.
“This is purely about explainability. It does touch on the whole issue of black box explainability, but it’s really driving at what rights do people have to an explanation. How do you make an explanation about an AI decision transparent, fair, understandable, and accountable to the individual?” Simon McDougall, Executive Director Technology Policy and Innovation of ICO, said.
“People are trying to understand how you can make sense of what neural network models come up with – how did they get to their answers? For many regulated industries, such as finance and healthcare, this is a big hurdle to deep learning adoption,” Björn Brinne, Chief AI Officer at Peltarion, told TechHQ.
If you were to apply for a mortgage or a loan at a bank and were refused, you’d want to know why, Brinne explained. If the bank had used deep learning, however, they may not be able to explain why the decision was made; “[…] you will need to have some form of ‘explainability’ to understand how the model uses the data to then provide the predictions.”
YOU MIGHT LIKE
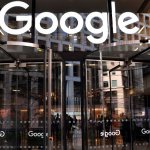
Google hit with $57m fine for GDPR breach
The developments of the framework in the UK
Recently, the UK Parliament released a report, Automation and the future of work, stating that the country is falling behind with automation and businesses as well as employees may face drawbacks.
Even so, the UK government has been adamant in supporting AI innovation by investing in AI projects and initiatives. The government will invest up to £20 million (US$25) in research and collaborative R&D to uncover new applications of AI in industries such as insurance and law. Besides that, a GovTechFund worth £20 million (US$25) will assist tech businesses that helps the government develop more effective and innovative solutions for the public sector.
Therefore, the increasing adoption of AI in supporting or making decisions in organizations is a hot topic among experts. As a result, calls for a framework to outline the mechanics behind AI-driven decisions has been noted since 2017 by Professor Dame Wendy Hall and Jérôme Pesenti and again in 2018 by the government.
This year, the ICO, together with The Turing (The Alan Turing Institute), launched a consultation in preparation for the regulation’s introduction to the public in 2020.
Google just recently released “Explainable AI“, a set of tools and framework which enables developers to monitor the behavior of their machine learning models visually.
The stated initiatives encourage the transparency of how machine learning models make decisions whereby AI adopters can have a more comprehensive view of the matter, taking more accountability of decisions generated by the machines.