Why fair artificial intelligence might need bias
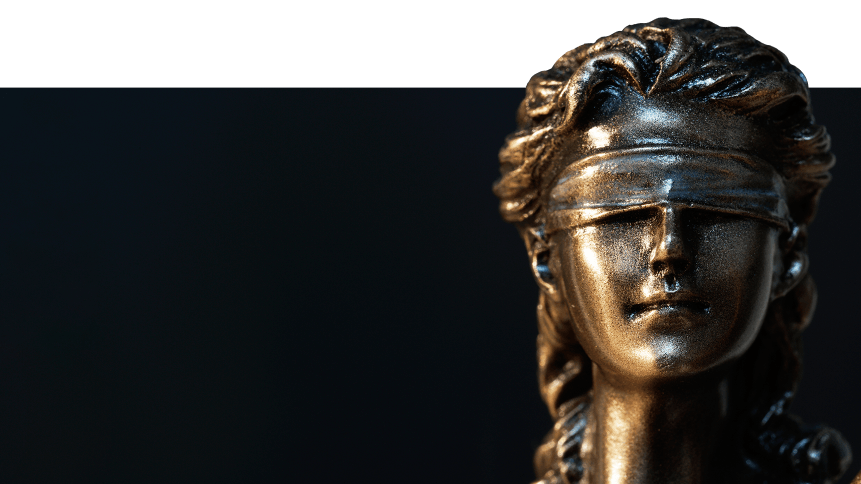
Businesses across industries are racing to integrate artificial intelligence (AI). Use cases are proliferating, from detecting fraud, increasing sales, improving customer experience, automating routine tasks, to providing predictive analytics.
With machine learning models relying on algorithms learning patterns from vast pools of data, however, models are at risk of perpetuating bias present in the information they are fed. In this sense, AI’s mimicking of real-world, human decisions is both a strength and a great weakness for the technology— it’s only as ‘good’ as the information it accesses.
Of course, this challenge is not news; as innovation continues, AI and machine learning ethics are regularly touted as crucial to the technology’s development.
It’s on the radar of organizations, world governments, and the machine learning community. In fact, to date, there has been a huge amount of work on ethical AI principles, guidelines and standards across different organizations, including IEEE, ISO and the Partnership on AI.
On top of these principles, there has been a growing body of work in fairness, accountability, and transparency— with a number of possibilities offered to help remove quantitative bias from the picture, but few real solutions.
Fair data, fair AI
As applications get more varied and complex, huge benefits in speed and efficiency must be traded off against the technology’s potential for bias.
Organizations must agree on concrete values and what they consider ‘fair’— but with no universal definition of fairness, and companies made of many people with conflicting opinions and different ideas— determining these wide, moral-based policies is easier said than done.
In an article for TechHQ entitled Responsible AI takes more than good intentions, Accenture’s Responsible AI Strategy Manager, Caryn Tan, said creating “ethical guardrails” relied on company staff from across the business working together and sharing perspectives.
“The objective is to collaboratively make effective decisions. Key individuals should be chosen from within development teams so that they can escalate issues as they arise,” she said.
“There also needs to be strong links between data scientists and legal and compliance specialists.”
Tan continued: “People who can operate comfortably in both worlds are increasingly valuable, so training should focus on embodying such ways of thinking and working.
“While data scientists wield the power of the code, and ultimately the outcome of the AI, others within the organization have critical input in guiding how the code is written.”
Writing in Harvard Business Review, Accenture Labs’ Senior Principle of Responsible Innovation and Data Ethics, Steven Tiell, said that with regards to forming an AI ethics committee within the business, there is an important distinction to be made between the definition of bias and fairness.
“Bias is a feature of statistical models, while fairness is a judgment against the values of a community,” said Tiell. “Shared understandings of fairness are different across cultures. But the most critical thing to understand is their relationship.”
YOU MIGHT LIKE
Responsible AI takes more than good intentions
While we may naturally assume that, by definition, fairness lacks bias, Tiell said, data scientists must often introduce bias in order to achieve fairness.
In this sense, synthetic data can be added to historic data in order to promote a fair, representative balance— it is the introduction of positive bias. For example, an HR tool may rely on historic hiring data where women have been under-represented. Data scientists might choose to “correct” it, to ensure future machine learning models are fed data that leads to fair decisions.
“In many cases, there’s no possible way to be both unbiased and fair,” said Tiell.
Leading in AI governance
For companies with serious investments and future roadmaps based on AI, Tiell echoed the views of his colleague, in that organizations should develop ethics committees based on a broad, swathe of individuals from various areas of the company, both technical and non-technical.
The remit of this group, however, won’t just be in determining fundamental principles of fairness and ethics, it will also be in monitoring the impact of their products and services in the real-world.
“Who sits on these committees, why they were selected, to whom they are accountable, and what their purpose is, should be clearly thought through and articulated.”
To lead in the realm of AI governance as it continues to rise in importance, Tiell suggested focusing on three areas for growth.
# 1 | Establish governance for ethics & AI
Unintended consequences of AI can be severe and present serious risks to organizations. Businesses should have “robust and stakeholder-rich” governance to help ensure they identify and manage potential risks.
“Essential governance”, team-based process integrations that introduce minimal friction, can be a way to get the foot in the door quickly, but the overall strategic value may be limited.
# 2 | Understand potential bias (and fairness) upfront
Responsible organizations and data scientists invest huge amounts of time into discovering potential biases in data, and what the impact of this could be in algorithm selection.
How these biases might impact perceived fairness, if they are mitigated or amplified, should be considered carefully upfront.
# 3 | Provide mechanisms for recourse
Every organization using AI should be striving to provide a solution better than that which already exists. However, if an AI system fails to meet user expectations, an organization should have the ability for immediate recourse.
Organizations should consider how recourse can be made to a responsible engineering team, internal AI or ethics committee, and external governance.