Google’s Explainable AI to help decypher black box machine learning
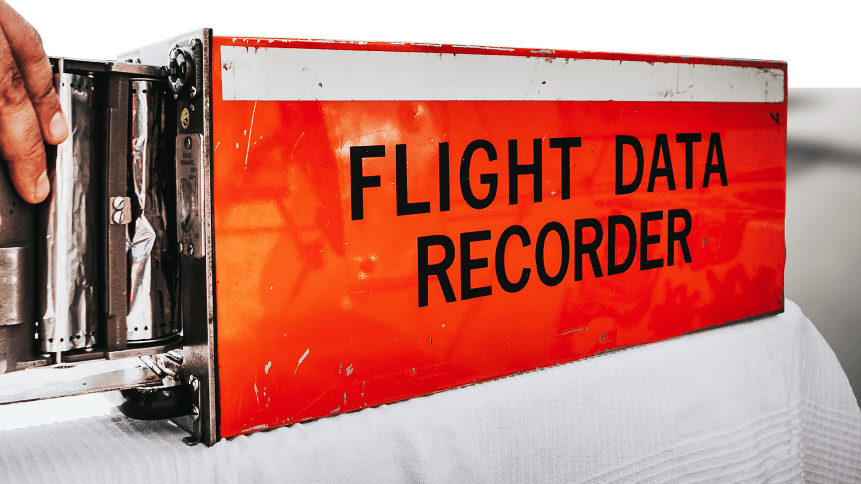
For all the advances that artificial intelligence (AI) offers in its ability to work through dizzying amounts of information to solve problems out of our reach, there is a problem holding us back– we don’t know much about how it makes its decisions.
Therefore, when an AI model works as it should, we lack the insight into how to enhance and optimize it to develop it further. When it doesn’t work in the way that it should, or how we’d expect or want it to, it is difficult to determine what elements of the decision-making process is going wrong.
Google is making a step closer to solving this conundrum with the launch of Explainable AI, a new service added to its cloud platform, which is aimed at adding some clarity to how machine learning models make their decisions.
According to Google, Explainable AI is a set of tools and frameworks for developing machine learning models that allow developers to “visually investigate model behavior” and “detect and resolve bias, drift, and other gaps” in machine learning models.
“Machine learning models can identify intricate correlations between enormous numbers of data points,” said the firm’s Cloud AI Director of Strategy, Tracey Frey in a blog post; “While this capability allows AI models to reach incredible accuracy, inspecting the structure or weights of a model often tells you little about a model’s behavior.
“This means that for some decision-makers, particularly those in industries where confidence is critical, the benefits of AI can be out of reach without interpretability.”
According to Frey, Explainable AI will work by quantifying each data factor’s contribution to the outcome generated by the model, helping users understand what has led to a decision.
The analysis could provide more transparency into the workings of AI and machine learning models, allowing developers to articulate those components to stakeholders. However, Frey acknowledged there were still limits to the service.
“Any explanation method has limitations,” she said. “For one, AI Explanations reflect the patterns the model found in the data, but they don’t reveal any fundamental relationships in your data sample, population, or application.
“We’re striving to make the most straightforward, useful explanation methods available to our customers while being transparent about its limitations.”
Speaking to the BBC, the IDC’s Philip Carter said that while Google may be the “underdog” in cloud against rivals AWS and Azure, that’s not the case for AI workloads; “There’s a bit of an arms race around AI… and in some ways Google could be seen to be ahead of the other players.”
YOU MIGHT LIKE
Knowledge mining will drive the next wave of AI
Google’s Professor Andrew Moore, the firm’s Cloud AI lead, told the publication that Explainable AI came as a result of the company’s own efforts to develop ways of understanding the decision-making processes behind its “really accurate machine learning models.”
“[…] in many of the large systems we built for our smartphones or for our search-ranking systems, or question-answering systems, we’ve internally worked hard to understand what’s going on.”
“Now we’re releasing many of those tools for the external world to be able to explain the results of machine learning as well. The era of black box machine learning is behind us.”
Making efforts to crack ‘black box machine learning’ is important for society, fairness and safety as AI sees rising adoption, said Prof Moore, but added that “no self-respecting AI practitioner” would release a safety-critical machine learning system without guardrails “beyond just Explainable AI”.
“[…] we’re able to help data scientists do strong diagnoses of what’s going on. But we have not got to the point where there’s a full explanation of what’s happening.
“For example, many of the questions about whether one thing is causing something or correlated with something – those are closer to philosophical questions than things that we can purely use technology for.”