Responsible AI takes more than good intentions
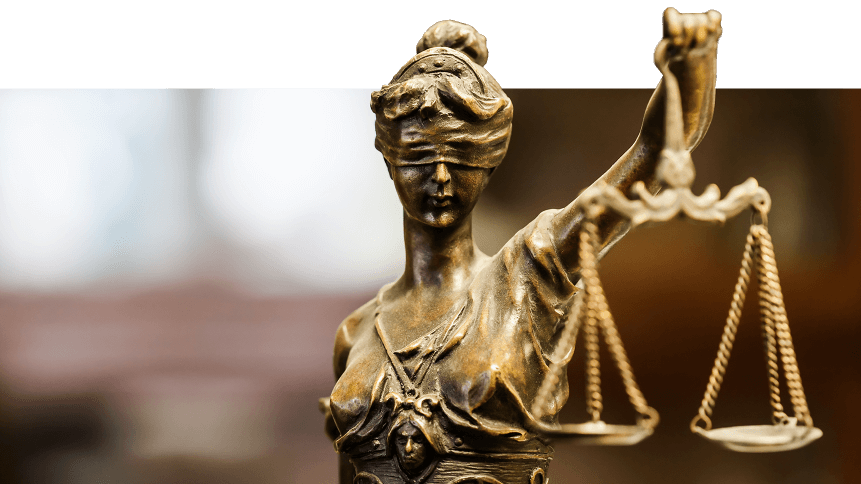
Last month, 42 countries signed up to the OECD’s common artificial intelligence (AI) principles. Just before that, the European Commission published its own ethics guidelines for trustworthy AI.
In fact, to date, there has been a huge amount of work on ethical AI principles, guidelines and standards across different organizations, including IEEE, ISO and the Partnership on AI.
On top of these principles, there has been a growing body of work in the fairness, accountability, and transparency machine learning community with a growing number of solutions to tackle bias from a quantitative perspective.
Grey areas in AI ethics
Both organizations and governments alike clearly recognize the importance of designing ethics into AI, there’s no doubt about that. Despite all of this, there has been little headway in finding practical ways for organizations to tackle real-life ethical dilemmas and make decisions when faced with them.
The use cases for AI are becoming increasingly varied and complex. The technology will undoubtedly bring huge benefits in terms of speed and efficiency, for example in predictive maintenance. But it may also force organizational leaders to make difficult trade-offs, especially in navigating the space where AI is used to make decisions that will impact human lives.
Fairness does not have a universal definition and requires organizations to think about what AI outcomes they are comfortable with. This will leave a huge grey area that they’re yet to navigate.
To make the problem even more challenging, organizations are made up of people trained in different fields, with different metrics of success. With so many conflicting opinions, how can they think about practical solutions to move forward?
AI ethics solutions
The grey area, where there’s often no single right answer, is the place to start. They need to bear in mind that ethical issues rarely present themselves as black and white. Many different responses might be appropriate.
These have to take into account the organization’s wider purpose and mission. And as the world doesn’t stand still, effective governance has to be a continual process that’s open to constant review and reflection. That process needs to consider both the organization’s vision and values as well as changes in the external context.
Combining the skills of people from an interdisciplinary background – data scientists, lawyers, business people and so on –will help to make sure that ethical dimensions are explored and analyzed from multiple perspectives.
Interdisciplinary teams are likely to have the breadth of vision needed to clarify the range of ethical standards to which a company must hold itself accountable.
For example, legal may see data protection and legal liability issues, whereas business people may detect ethical problems as a damaging brand and reputational trust that could drive customers away. And data scientists may see all these as potentially stifling their work.
But by working together and sharing their perspectives, different team members can identify a balanced response and make decisions that keep the business within its ethical guardrails.
Preparing for an AI-driven future
So how can organizations organize these interdisciplinary teams in practice?
An analogy well used by our responsible AI team is that rather than a police patrol passing down orders, you need to think about them as fire wardens. That means they are responsible for spotting and escalating issues that need attention. They raise the alarm if something looks like it could cause a problem.
YOU MIGHT LIKE
EU publishes new guidelines on ethical AI
Top of the list is executive-level buy-in to adopting a new approach to decision making about how AI is built. This means organizations should be training and supporting team members to comfortably work with employees from other functions.
The objective is to collaboratively make effective decisions. Key individuals should be chosen from within development teams so that they can escalate issues as they arise.
There also needs to be strong links between data scientists and legal/compliance specialists. People who can operate comfortably in both worlds are increasingly valuable, so training should focus on embodying such ways of thinking and working.
While data scientists wield the power of the code, and ultimately the outcome of the AI, others within the organization have critical input in guiding how the code is written. Effective communication between data scientist and other team members is key to enabling interdisciplinary decision making.
It is also important to encourage teams to raise potential risks, even if they turn out to be false alarms. To understand what’s involved in developing responsible AI, teams will need to develop their instincts.
That takes time, so they should be encouraged not to fear the consequences of pressing the alarm button. After all, dealing with a false alarm is infinitely preferable to one that’s gone unnoticed and is spiraling out of control.
Responsible, sustainable success with AI is ultimately all about people. They have to understand the ethical approach that best embodies their organization’s purpose.
They need to be empowered to take responsibility and act accordingly. That approach is most likely to support effective, agile governance resulting in responsibly designed, built and maintained AI.
For AI to fulfill its potential, organizations must seek the opinions of those whose lives it will affect, whether that’s consumers, employees or citizens more broadly.
That way, they’ll ensure that the AI they are developing remains responsible and ethical in a fast-changing world.
This article was contributed by Caryn Tan, Responsible AI strategy manager at Accenture.