Busting three pervasive myths about AIOps
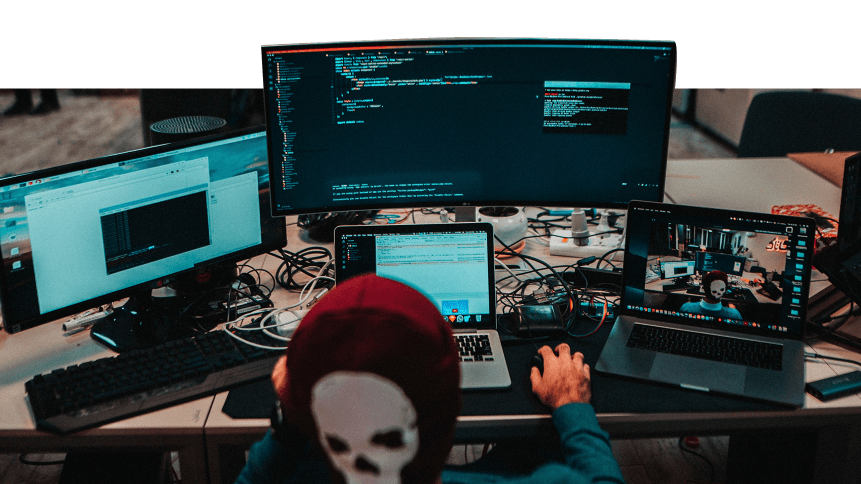
Since Alan Turing formalized the concept in the 1950s, business leaders, technologists, and futurists alike have been enamored of the potential for artificial intelligence (AI). Today, the dream of AI is largely a reality, as nearly 30 percent of global businesses have already adopted it in one form or another and 75 percent of executives expect to have actively implemented it in the coming years, according to research by The Economist.
Businesses across industries and verticals are eagerly hopping on the AI train, finding immediate and innovative uses for the technology in their daily operations. Consumer-facing businesses are using AI-powered solutions to improve customer engagement and business outcomes. Organizations in industries like healthcare, recruiting, and energy exploration rely on AI to sift through billions of data points to drive smarter, more efficient, and cost-effective daily operations. Even IT professionals are getting in on the act, using AI to fortify cybersecurity capabilities and streamline time-consuming and repetitive support tasks.
Yet, like all emerging technologies, the hype surrounding AI is quickly reaching a crescendo. A quick search of articles around AI for use in IT Operations and Services Management (ITOM/ITSM) yields more than one million results, each seemingly with its own hot take about possible AI use cases and capabilities for enterprise IT teams.
But with so much buzz around AI it’s easy to get lost in the excitement and potential of the technology, and difficult to separate AI fact from AI fiction. So, it’s time to set the record straight and bust three of the more pervasive myths about AI in IT and get a clearer understanding of what it can and can’t do.
Myth 1: Teams are eager to trust AI
Many publications and prognosticators are hailing how AI will revolutionize the IT operations sector. Business leaders are buying in, hoping to reap the benefits of the massive gains in cloud computing power and advanced algorithmic capabilities to support improvements in critical IT operations like threat detection, event management, and configuration management.
But not everyone is eager to hop on the AI train just yet. IT pros have invested time, money, and a ton of effort into their careers and there’s real fear — even if it’s mostly in response to the hype — of eventually being replaced by machines.
Implementing an AIOps solution is a delicate process that must be meticulously planned and strategically deployed. Organizational leaders must consider the potential impact of AI on its business and people, and determine how the technology can be best applied to meet the demands of the business without disrupting the entire culture, and be transparent with the entire team about how and why it’s being considered.
YOU MIGHT LIKE
Poppy Gustafsson: ‘The war on cybercrime needs AI’
The most successful AI deployments begin with a robust training program that helps assuage those fears and builds a culture of innovation. Part of that effort means gaining buy-in and acceptance from the teams that will be most impacted by the new technology and dispelling their skepticism by proving the value of the solution.
One method for proving the solution’s value to the team could be to mirror their existing agile software development methodology to run several small-scale tests in a development environment.
For example, if an operations team’s biggest challenge is identifying a root cause from amid a flood of alerts from application or network performance monitoring tools, they must first be able to trust that the alerts are accurate, timely, and relevant. Using the same data from the production environment, teams can deploy the AIOps tools in a development environment to evaluate its capacity for sifting through noise, identifying a root cause, and proposed remediation.
With complete transparency to the results and running similar tests across a variety of scenarios, it’s possible to build a consensus among the team that adopting AI tools is a prudent move.
Myth 2: AI is set it and forget it
Many IT pros assume that AI solutions — especially AI for IT operations (AIOps) platforms — can be tuned once, deployed everywhere, and left untouched indefinitely. After all, that’s the expectation and their experience with modern automation systems, which allow users to just set them once and forget about them until new automation-ready tasks and activities come along.
But today’s IT environment is more dynamic and complex than ever, and conventional automation approaches based on rules and policies are no longer enough for the ever-changing nature of today’s cloud-based, serverless environments.
The promise behind AIOps tools is that by using AI, organizations can fully automate many of their IT Ops teams’ traditional tasks and free resources for higher-value, more complex challenges. The expectation is that instead of relying on human engineers to figure out why a service or application has stopped working or slowed down, AIOps tools can use data to interpret the problem and fix it automatically.
But because artificial intelligence isn’t a direct replacement for human intelligence, AIOps tools still need to be directed and “trained” how to do their job. Emerging technologies such as cloud-native architecture (serverless, ephemeral workloads), DevOps, and multi-cloud infrastructure produce exponentially more data than legacy technologies, meaning that human engineers must still intervene to continuously optimize the tools and tweak workflows to accommodate changes in data sets, workloads, and the demands of mission-critical functions like DevOps.
YOU MIGHT LIKE
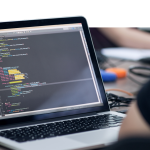
Five essential steps to prepare for AIOps
Myth 3: Technology is what makes AI valuable
One of the more persistent myths about AI (and AIOps in particular) is that the value of the technology resides in the tools itself. It’s easy to get caught up in the marketing speak about features or capabilities.
In reality, the true value of AI tools doesn’t entirely come from the quality of the algorithms, as much as from the quality of the data that powers them. Many IT professionals mistakenly believe that AI is easily bolted on to existing data or platforms and ready to deliver magic. But the algorithms that underlie the sparkling branding and packaging require having a steady stream of accurate, relevant, and complete data to work their magic.
As a result, for organizations implementing an AIOps strategy or deploying new technologies, it’s imperative to critically evaluate where data for the tools is coming from, how it’s ingested, and whether there are gaps in the information or if it provides a complete picture of the state of the IT environment. Is the data contextual? Is it accurate? Is it optimized for algorithmic ingestion? In order to get the most value from any of these tools, it’s critical to focus on the data first.
AIOps is an exciting development in IT’s never-ending pursuit of efficiency and reliable service delivery. The buzz around the technology — and the myriad solutions it powers — grows stronger and louder by the day, enticing many IT pros with promises that may or may not be real. Organizations looking to implement AIOps tools in their digital operations should take great care to critically evaluate solutions against their promises to help ensure they get the specific tools and capabilities their business demands, without any of the unpleasant surprises.
This article was contributed by Jiayi Hoffman, Data Science Architect at OpsRamp.