Google rolls out privacy tool for AI developers
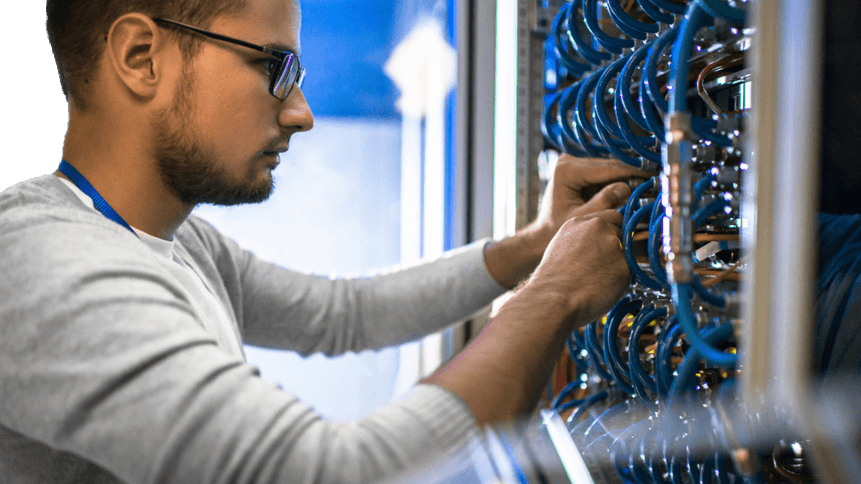
Google has released the TensorFlow Privacy tool, an update to its open source TensorFlow machine learning framework, that will allow developers to enhance the privacy of their AI (artificial intelligence) models.
The TensorFlow framework is used the world round by AI engineers to create text, audio, and image recognition algorithms; TensorFlow Privacy will enable these projects to integrate a statistical technique known as ‘differential privacy’.
“If we don’t get something like differential privacy into TensorFlow, then we just know it won’t be as easy for teams inside and outside of Google to make use of it,” Carey Radebaugh told The Verge. “So, for us, it’s important to get it into TensorFlow, to open source it, and to start to create this community around it.”
The introduction of the privacy update is in keeping with Google’s principles for responsible AI development. The use of differential privacy essentially means that AI models trained on user data can’t encode personally identifiable information. It’s a common approach for AI models and one that has been employed by other companies including Apple.
YOU MIGHT LIKE
VR is being used to train staff in ‘soft skills’
Google also uses it for its Gmail smart reply feature and, as The Verge notes, this is a good analogy of the importance of personal data privacy; the function is based on highly-personal data collected from millions of email users. If any of those recommended replies featured any of this personal information, it would be catastrophic.
Differential privacy removes the chance of personal information being presented with certainty, without changing its overall meaning. The outcome will, therefore, be relevant, but independent of any one person’s data.
There are drawbacks to differential privacy— it came sometimes remove relevant or interesting data, which could be vital in making language sound natural, for example. By making TensorFlow Privacy openly available, however, Google hopes AI engineers around the world can help to overcome some of these challenges.
The ability to enhance user privacy with just a few lines of code is a significant step for the ethical development of AI, of which the quality and privacy of the vast, human-generated datasets that power projects has been a key concern.