Model developed to stop autonomous AI mistakes
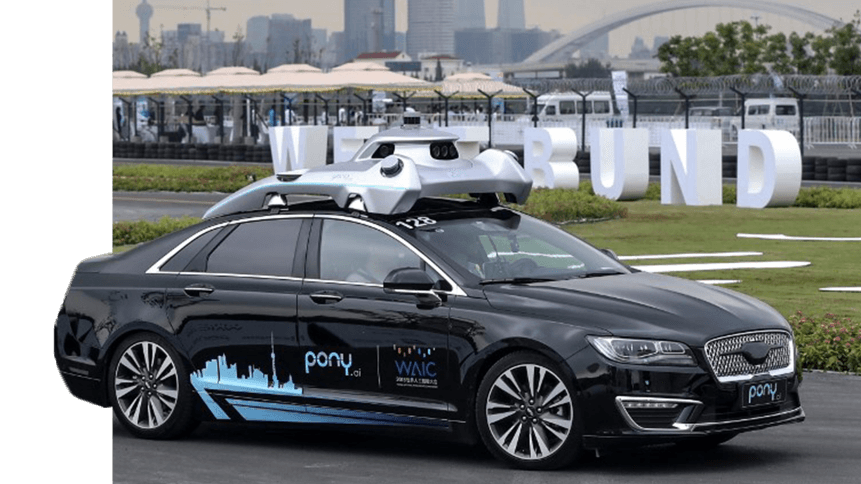
Artificial intelligence (AI) systems are centered on the principle of ongoing ‘learning’— but what happens when the information used to train those algorithms doesn’t quite sync with their intended real-world application?
In the case of AI software used to control an autonomous vehicle or an industrial robot, the implications could be pretty significant.
That challenge is being addressed in a joint project by MIT and Microsoft— the two have developed a model that identifies when an autonomous system has learned from training examples that don’t quite gel with reality.
According to MIT News, engineers could use the model to improve the safety of AI systems in driverless vehicles or autonomous robots.
In the case of driverless cars, AI systems are trained in virtual systems to prepare them for events on the road but, occasionally, the car may make an error in the real world because an event occurs that should, but doesn’t, alter the car’s behavior.
“Consider a driverless car that wasn’t trained, and more importantly doesn’t have the sensors necessary, to differentiate between distinctly different scenarios, such as large, white cars and ambulances with red, flashing lights on the road,” reads the MIT News report.
“If the car is cruising down the highway and an ambulance flicks on its sirens, the car may not know to slow down and pull over, because it does not perceive the ambulance as different from a big white car.”
The example has inescapable parallels with the highly-publicized crash of a Tesla in 2016. Following an extensive probe, the incident was revealed to be a result of a combination of driver inattention and the car’s autopilot system, which was not able to distinguish between the white tractor of a truck and the bright sky behind it.
The model developed by Microsoft and MIT uses the human element to refine the AI’s development and uncover “blind spots”. As with traditional approaches, the researchers will put an AI system through simulation training. However, a human will monitor when the system makes, or is about to make mistakes.
Researchers will then combine training data with human feedback data and use machine-learning to create a model that pinpoints the situation in which the system is most likely to need more information in order to act in the right way.
Interestingly, the researchers validated their methods using video games, with a simulated human correcting the learned path of an on-screen character. The next step will be to incorporate the model with traditional training and testing approaches for autonomous robots with human feedback.
“The model helps autonomous systems better know what they don’t know,” says first author Ramya Ramakrishnan, a graduate student in the Computer Science and Artificial Intelligence Laboratory.
“Many times, when these systems are deployed, their trained simulations don’t match the real-world setting [and] they could make mistakes, such as getting into accidents..the idea is to use humans to bridge that gap between simulation and the real world, in a safe way, so we can reduce some of those errors,” Ramakrishnan said.
The end goal is to have these ambiguous situations labeled as blind spots. But that goes beyond simply tallying the acceptable and unacceptable actions for each situation.
Researchers used the Dawid-Skene algorithm, a machine-learning method used commonly for crowdsourcing to handle label noise. The algorithm takes as input a list of situations, each having a set of noisy ‘acceptable’ and ‘unacceptable’ labels.
It then aggregates all the data and uses some probability calculations to identify patterns in the labels of predicted blind spots and patterns for predicted safe situations. If the system performed correct actions nine times out of 10 in the ambulance situation, for instance, a simple majority vote would label that situation as safe.
“When the system is deployed into the real world, it can use this learned model to act more cautiously and intelligently…if the learned model predicts a state to be a blind spot with high probability, the system can query a human for the acceptable action, allowing for safer execution,” Ramakrishnan says.
While the safety of autonomous vehicle and machinery systems is paramount, it’s not to say the same model couldn’t be applied to other AI systems to increase the accuracy and quality of actions when a situation is ambiguous.