Knowledge graphs change the nature of business intelligence
The treasure trove of data at a company’s disposal can uncover insights that bring a highly positive impact on strategic decision-making. But most of this data is dispersed across the organization and cannot be used easily by existing data processing systems, including AI.
In fact, around 90% of information held in an average business comprises what’s known as unstructured data. Common types of unstructured data include freeform text from customer reviews, the contents of company messages and emails, report documents – any digital information that’s not rigidly formatted. In contrast, structured data might be spreadsheets, existing database contents, survey questionnaire responses in multiple-choice format, and numeric, tabulated financial information.
In the past, unstructured data required extensive pre-processing before it could be analyzed, and there are plenty of solutions that claim to be able to do just that. However, they are often use-specific and limited in capability. One such example is OCR, which is useful to extract data, but the data itself remains unstructured and of minimal benefit. Speech-to-text software likewise creates unstructured data that, in turn, requires collation and standardization before it creates value.
Knowledge graphs are a solution that can surface information from both structured and unstructured data. They create connections between data stored in disparate systems and build maps of related information. In a business context, knowledge graphs allow users to discover information that’s related to what they’re doing but sourced from places that were previously difficult to access.
As an example, a Marketing department’s records of online product reviews on social media can be cross-referenced to the sales database, creating a record of comments against each SKU. Further inquiries might uncover that the majority of negative reviews are from customers in a particular area and are concerned with delivery problems. That then can inform decisions around the choice of logistics suppliers or at least create an action point to address the issue before it impacts the brand badly.
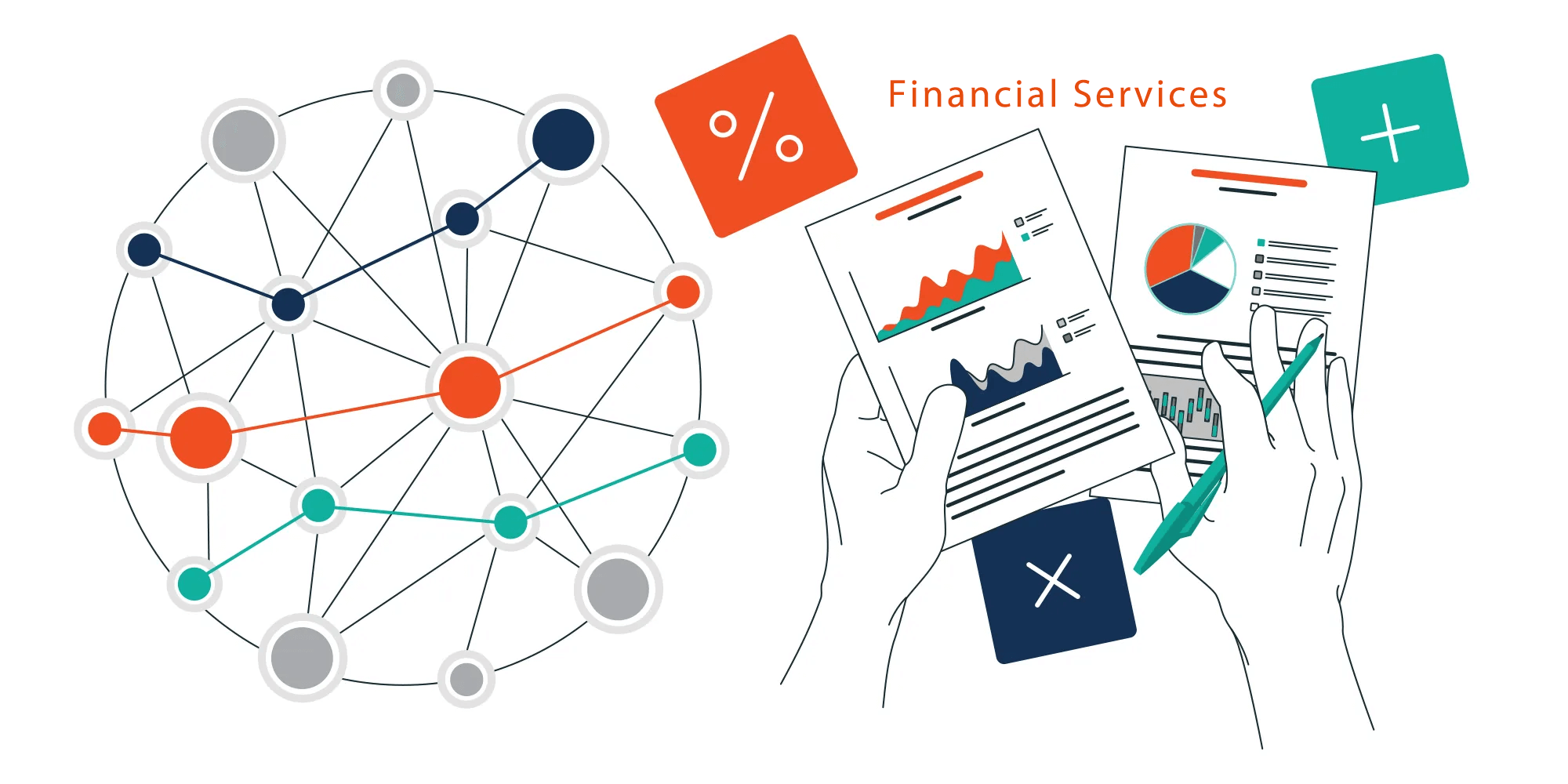
Source: Ontotext
In traditional databases, either relational or non-relational, those types of queries are difficult to construct and highly resource-intensive to run. They stumble at the format of unstructured data and have to rely on manual sifting through datasets. It means responses are slow, a problem that worsens exponentially as queries process through various data sources. Each unique query is ephemeral, with connections made on the fly each time.
Conversely, knowledge graphs in Ontotext GraphDB are reusable, so connections between data points (a customer’s name, their social media handle, the location of the store they buy from, the content of their communications with the customer service desk, and so on) are established once and queries are quick in traversing across multiple data instances.
In addition, the graph database creates a structure that exposes large amounts of metadata (information that provides context and associated information), including non-semantic sources. It forms the link between explicit, quantitative data from structured sources and, in some cases, the extra data that places it in context. That creates rich search results that come with a traceable record of provenance (where data is coming from) and enables deeper analytics and understanding of trends.
As a result, companies get access to a broader range of information they possess. This enables them to make better, more informed decisions based on provable, known sources from internal and external data repositories.
The database engine is scalable thanks to its microservice-based code, so it can create high-availability, robust resources as required and can be hosted anywhere that can run a Kubernetes cluster. Cloud-agnostic, Ontotext GraphDB interfaces easily with existing tools and BI platforms, ensuring users work in familiar environments, using familiar language and query methods. The API-first architecture means the platform is highly extensible, with a range of connectors and plugins that give access to existing data resources and tools.
The knowledge graph infrastructure and processing requirements are even lightweight enough to handle near real-time data. This enables powerful options to explore and draw inferences from data sources such as IoT sensors and time-dependent databases.
Businesses have a goldmine of information, often referred to as an untapped resource that has been, until now, difficult to mine economically. With the capabilities that Ontotext GraphDB enables, these rich seams of business insight are now easily accessible. Unstructured data poses challenges, but they are surmountable. Companies that incorporate knowledge graphs into their data architecture foundation have an opportunity to leverage their existing resources to gain a competitive advantage over those relying on last-generation data processing.
To find out more about GraphDB and Ontotext, head over to the company’s website, and you can download and run GraphDB to begin your initial assessment of what’s possible right away.