Revenue boosts, improved efficiency, and reduced risks: The role of semantic knowledge graphs
The problem with disorganized data
Medium to large-sized businesses today are presented with a seemingly impenetrable morass of data. It can certainly be frustrating when there is obvious actionable intelligence available, but no clear means of extracting it. Analysts are challenged by data coming from diverse sources and in different formats, and complex and time-consuming sanitization processes have to happen before analysis can begin.
Data stored in isolated silos prevent a comprehensive view of information, making it difficult to ensure quality and consistency of results. Without a full understanding of the relationships between and meanings in data resources, it’s challenging to interpret raw information.
When data lacks clear and consistent interpretation, it hurts a business’s ability to gaininsights for decision-making, leading to missed opportunities or incorrect judgments. Data transformation and schema alterations further increase the risk of data incongruities. Furthermore, the presence of unstructured data, which lacks any consistent model, can exacerbate problems with queries and inference.
Disorganized data may not always start out so and is often produced by legacy technology. While once state-of-the-art, relational databases now hinder flexibility due to the innate rigid structure on which operations are forced to rely. Relational databases can become a further problem as data volumes grow alongside the business because they become fragmented for use in different environments. This has the potential to affect multiple departments and stakeholders, as it hinders data accessibility and collaboration.
Doug Kimball is the CMO of Ontotext, a leading provider of semantic technology and enterprise-grade knowledge graph solutions. He told TechHQ: “We are in a time where more people than ever are aware of and thinking about data. It may not be specific as in types of data, or the need to integrate, but in a world where ChatGPT grew to such amazing popularity so quickly, there’s an inherent carryover to seeing the importance of data.”
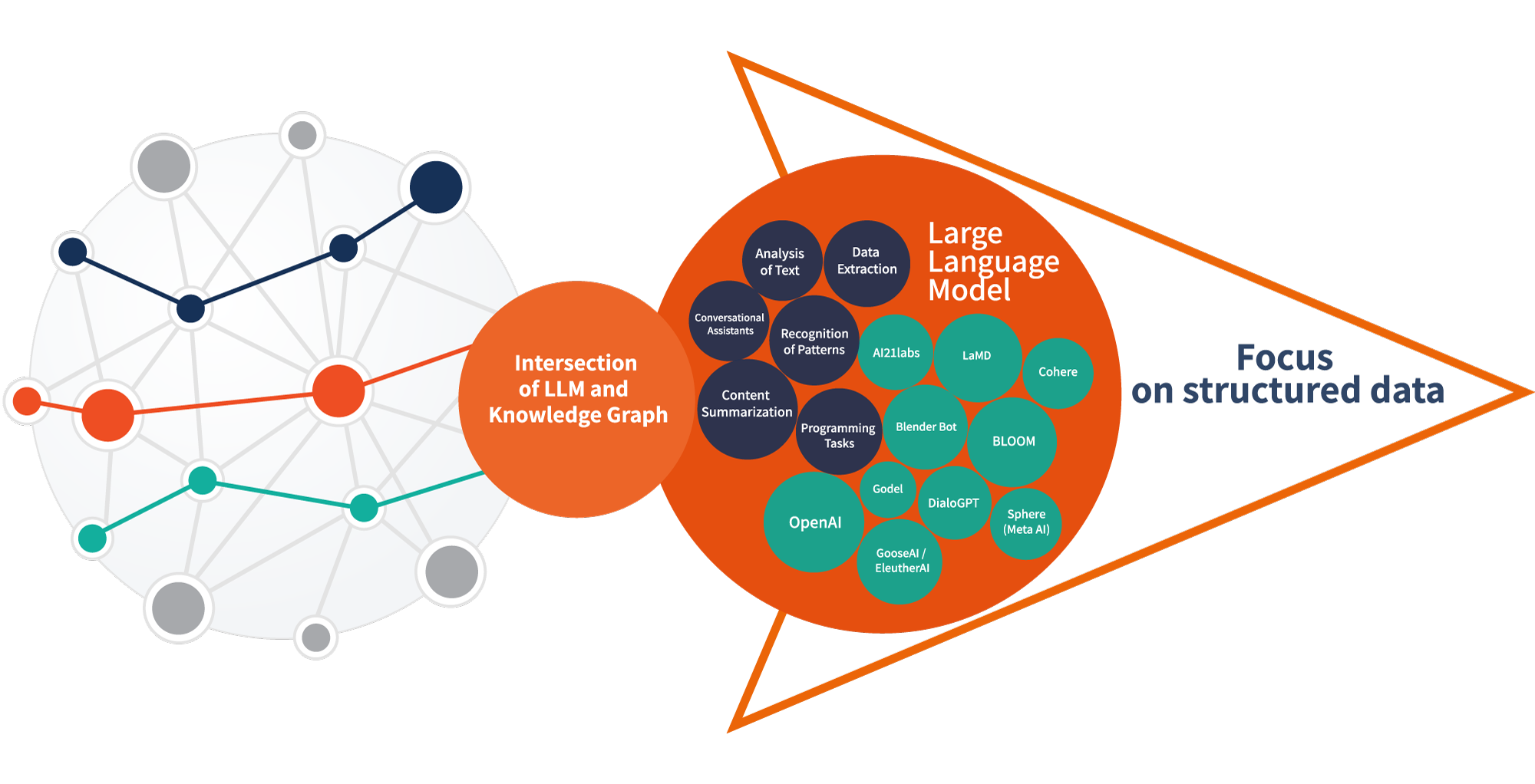
Source: Ontotext
Sumit Pal, the Strategic Technology Director at Ontotext, added: “With the AI juggernaut rolling on, data will decide who wins the race. Enterprises are evolving beyond software-driven to data-driven and AI-Knowledge-driven. With the commoditization of infrastructure, data stack and AI, data is becoming the currency in building a competitive edge.
“There is a yawning gap between data-driven capabilities enterprises are seeking and actual data with bottlenecks of silos, centralization, and organizational hierarchies hindering access, lack of ownership and accountability, data quality, usability, trust, and discoverability.”
What are semantic knowledge graphs?
To solve data challenges, drive efficiencies, and work with clean, quality data, enterprises need their data to have a proper foundation based on semantic knowledge graphs. Over the past few years, most companies have been navigating some form of digital transformation with the hopes that leveraging data effectively will work to their advantage. However, many efforts ultimately fail because the absence of semantic graphing prevents companies “connecting the dots” in their data.
A semantic knowledge graph is a network of interconnected data descriptions using ontologies and semantic metadata that contextualize information. Leveraging the structured semantics provided by RDF standards, it models real-world facts and utilizes automated reasoning for deeper insights. Computers can effortlessly interpret data, while users excel at discovering and sharing what’s needed by different departments and stakeholders, even when data is silo-ed.
When data is parsed without centralized control, semantic knowledge graphs help prevent misinterpretation by ensuring consistency, accuracy, and interoperability across diverse data sources. These might include data lakes and fabrics in different formats, like TSV, JSON, or XML. They can also act as a check for the quality of data and metadata from different environments, ensuring consistency and reliability.
Kimball told TechHQ: “With most organizations having multiple types of data, multiple ways of accessing and sharing those, and multiple types and experiences of users needing to leverage that data, applying semantics to have a shared and agreed understanding of the data is key. Beyond that, being able to make the most of all the data a company has, especially its unstructured data, usually held in different places, semantics can help to tie all these together for best usage.”
Unlike traditional data management systems, knowledge graphs grow alongside enterprise data and adapt to changing needs without necessitating schema restructuring. Their flexibility and extensibility allow addition or modification of elements, ensuring businesses remain agile and future-proof. They also update automatically as new data and data sources emerge, significantly reducing the manual effort typically required for data maintenance.
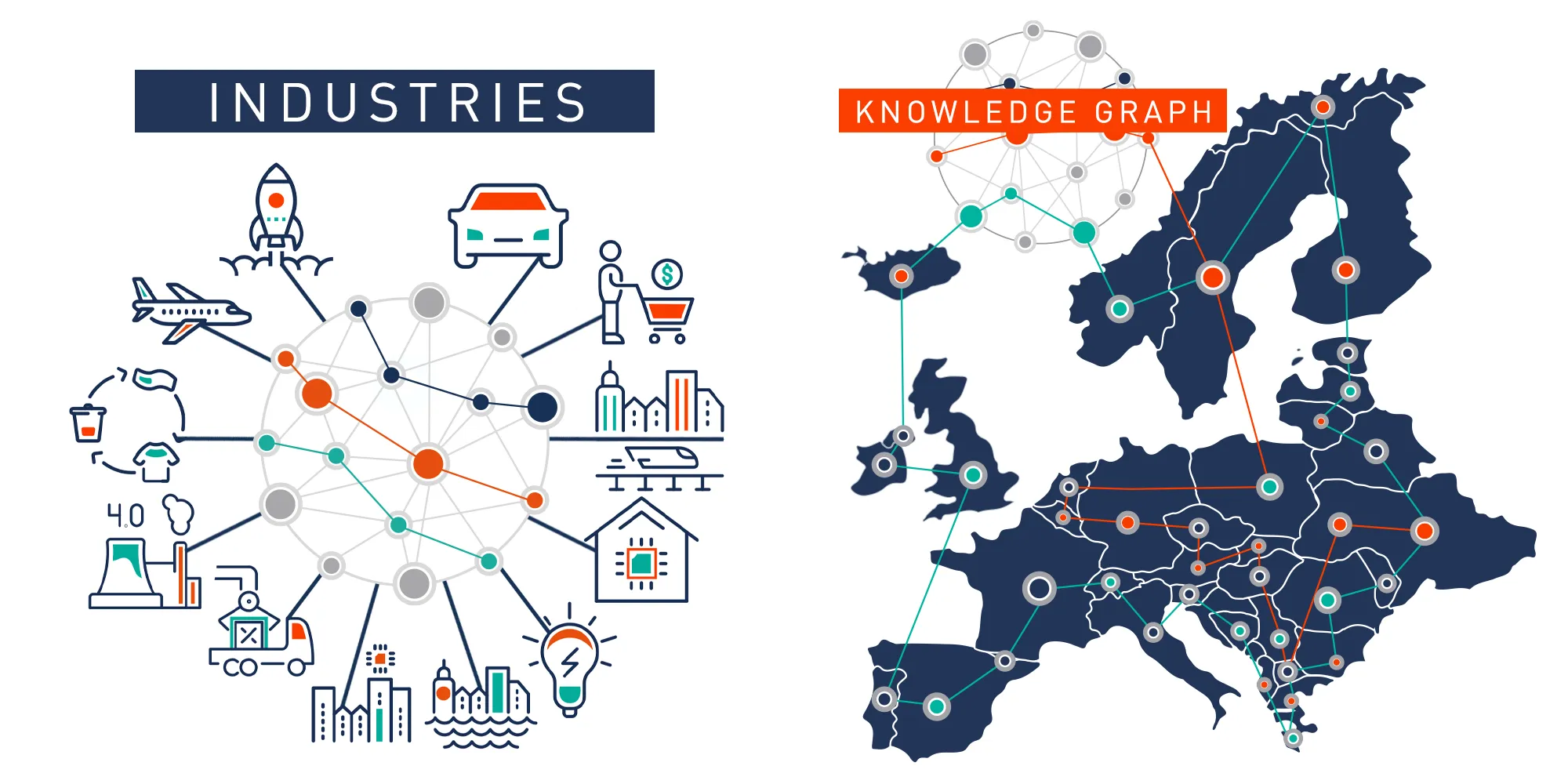
Source: Ontotext
While you may not have explicitly seen them, knowledge graphs are now used under the hood of many established systems and platforms, including search engines, AI, digital twins and other initiatives. Knowledge graphs can be seen as the co-pilot for LLM’s, where knowledge is modelled as a graph, and help Generative AI applications provide accurate responses, mitigating hallucinations and mis-information. Before taking the big bound towards Artificial General Intelligence, we need to take a smaller step of achieving
Artificial Contextual Intelligence and Knowledge graphs is the foundation that this AI revolution should be based on.
The knowledge graph difference
Pal told TechHQ: “Data and information is only as good as it is contextually navigable. Knowledge graphs are a game changer that interconnects, integrates and harmonizes siloed data, adds context and semantics, removes ambiguity, expedites knowledge discovery, data integration, analysis and decision making.
The single most attractive reason why enterprises are embracing the idea of organizing their knowledge in a knowledge graph for enhanced analysis to create competitive advantage. It diminishes the distance between data personas and data with semantics and context to identify non-intuitive effects and outcomes.”
By providing a coherent and comprehensive view of data, semantic knowledge graphs create better decision-making in organizations. The tangible impacts include those closest to a C-level executive’s heart: revenue boosts, improved operational efficiency, and reduced risk.
State-of-the-art graph technologies allow new revenue streams to be developed easily from existing assets and data products. They facilitate a deeper understanding of customer behavior, preferences, and market trends, so businesses can tailor their offerings more effectively. They improve efficiency by extracting value from previously inaccessible enterprise data, either from siloes or disparate systems presenting unusual or incompatible formats. Decision-making risks are reduced thanks to the clearer understanding of data relationships and patterns that knowledge graphs provide, resulting in an enhanced ability to see pitfalls or market shifts.
Knowledge graphs can also help with the integration of new technologies, like generative AI. AI models can use semantic graphs to gain clearer insights into data entities and their relationships, resulting in more accurate and context-rich content. The graph as a point of reference also helps combat issues like hallucinations and logical errors in AI-generated content, reducing the risk of misleading information.
Applications
Semantic knowledge graphs are applicable in most industries, especially when considering any form of AI integration. In healthcare, they can integrate data from sources that were previously unconnected, like patient records, research papers, drug libraries, and clinical notes. Healthcare professionals can perform advanced analysis, facilitating quicker diagnoses, personalized treatment options, and less wasteful clinical decisions. Additionally, knowledge graphs can aid researchers in navigating disparate scientific literature, expediting drug discovery, and enabling evidence-based research for improved healthcare outcomes.
In financial services, semantic knowledge graphs aid risk management by amalgamating diverse data sources, detecting fraud, assessing market trends, and ensuring regulatory compliance. They also optimize customer experiences through personalized services based on comprehensive customer data from multiple collated sources.
Media and publishing businesses benefit from semantic graphs by using them to improve content recommendation systems and offering users tailored content based on wider preferences and peripheral behaviors exhibited outside the core of the main platform.
Public sectors and defense agencies utilize knowledge graphs for intelligence analysis, connecting discrete datasets to uncover insights critical for national security and policymaking. In manufacturing and logistics, semantic graphs optimize supply chain operations, enabling predictive maintenance and quality control by linking and analyzing data from production stages and third-parties.
Most companies tend to deploy knowledge graphs on a case-by-case basis rather than strategically across the organization. While this may seem like a more pragmatic approach, it often limits the broader potential and benefits that a comprehensive implementation of knowledge graphs could offer.
Making decisions with confidence is difficult without, structured data management, and the Ontotext team has decades of experience enabling businesses to harness the full potential of all their data with semantic knowledge graphs.
Pal told TechHQ: “Ontotext offers the necessary tools and partners with key players to manage, integrate, harmonize, and analyze disparate data for building successful knowledge graphs. Its recipe includes linking data for context, adding semantics, and leveraging a semantic data model.”
Kimball added: “Essentially, one of the greatest strengths of a knowledge graph is its ability to ingest data from a variety of sources, classify and organize them, and add value through reasoning, opening more connection points and insights. This, in turn, also supports the de-siloing of data, to make sure that decisions are taken on the right information, regardless of where it is managed.”
Whether optimizing knowledge distribution, supporting digital twin or data fabric initiatives, advancing generative AI, or addressing data catalogue challenges, Ontotext can fuel your data strategy and help maintain a competitive edge. Learn more about knowledge graphs and how they can solve your business’s data challenges by downloading the Ontotext whitepaper ‘Demystifying Semantic Standards and Knowledge Graphs’.