The AI technology revolution in practical data protection in Finance
Giving out what was once considered highly personal and sensitive information has now become a part of our everyday lives. More people go online to do their shopping, manage their finances and make digital payments. But with consumers increasingly concerned by what might happen to their data once the transaction is over, financial firms are looking into new and improved techniques to keep personal information secure.
The public’s privacy protection interests are strongly backed by legislation. Last year, a total of $1.2 billion in fines were imposed by the EU on those who had breached its General Data Protection Regulation (GDPR) since 28 January 2021 – a $180 million or seven-fold increase compared with 2020 figures, according to a recent report by DLA Piper law firm.
Protecting Personal Data
As the consequences of data privacy breaches extend beyond loss of consumer trust and hit the bottom line hard, methods to secure data are also evolving. When someone shares photos of the damages to their property on an insurance app, they know that the data has to be processed and compared to a relevant database to enable them to resolve their claims.
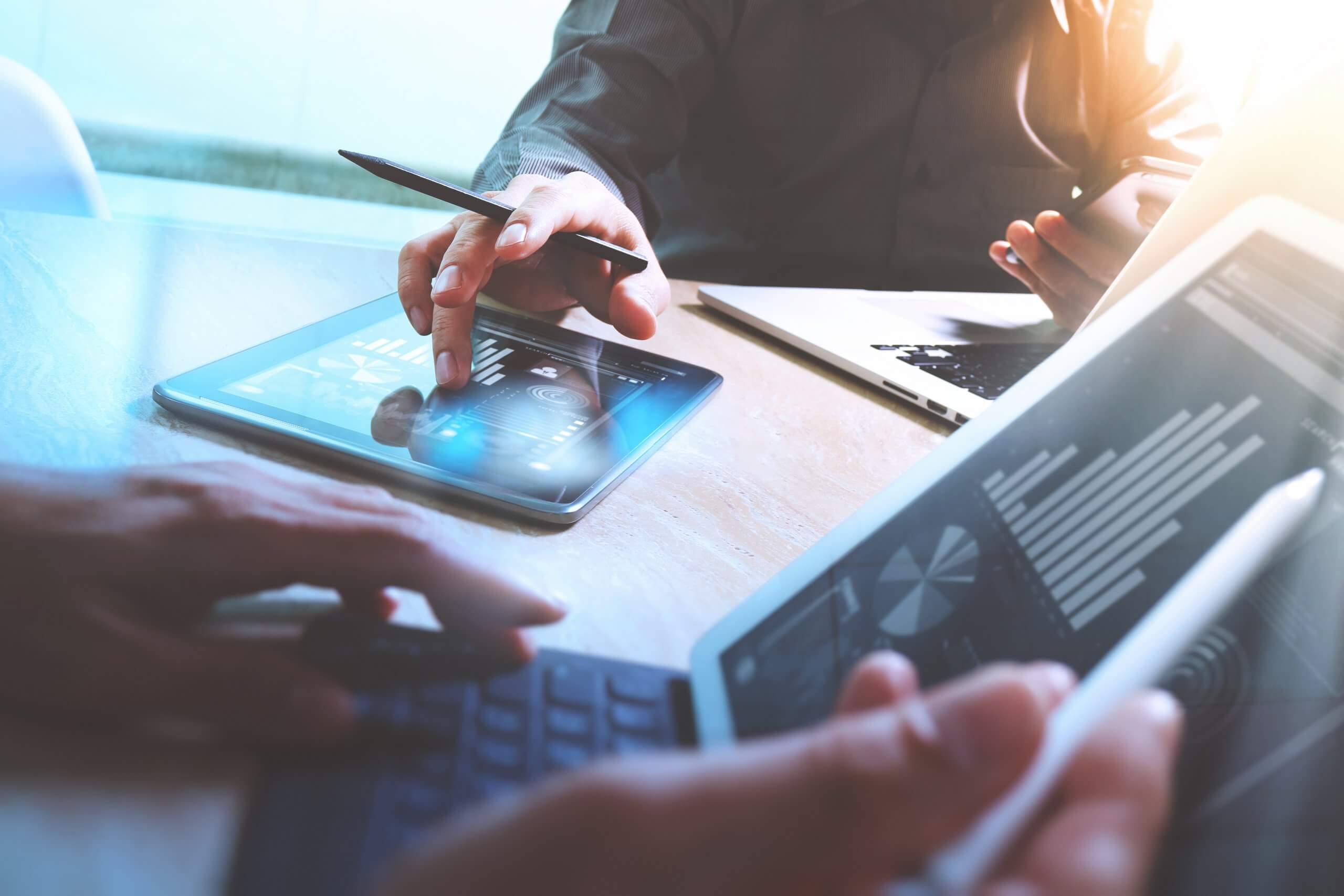
Source: Shutterstock
Sharing this type of identifiable information raises privacy and anonymity concerns. And it can be relatively trivial to put information together. Such was the case in 1997 when a graduate student successfully de-anonymised the Governor of Massachusetts from health records released by an insurance group.
Even though the records didn’t have apparent personal information like names and addresses, the student managed to pinpoint the governor by cross-referencing the anonymised health records with other sources of information, the public voter list in this case.
This tactic, known as the linkage attack, also managed to identify Netflix users based on their watching history – even though the subscription streaming company had assured all personally identifiable information (PII) was removed when the dataset was released for its 2006 Netflix Prize challenge.
Since 2006, and certainly since 1997, the amount of data that is sent digitally has multiplied by a significant factor. This has resulted in the issue of the ease of linkage attacks, and the need for de-anonymised information at scale.
Differential privacy
Anonymising data alone is not enough. There needs to be a way to prevent linking one dataset to another, uncovering the individual or individual company. That’s where differential privacy (DP) comes in.
This advanced AI technique protects data by adding digital “noise” to blur any connection to an original data source, while retaining all the required information to expedite genuine uses. However, achieving this on large datasets is too computationally expensive using traditional AI computing methods based on GPUs and CPUs. Until now.
Stanford University researchers have found they could speed up AI training with differential privacy by more than 10 times using Graphcore IPUs (Intelligence Processing Units).
Differential privacy stochastic gradient descent (DPSGD) technique
The Stanford team conducted the first analysis using IPU hardware to train on a dataset containing 1.3 million images, leveraging the differential privacy stochastic gradient descent (DPSGD) technique. DPSGD protects sensitive data by ensuring an individual’s data cannot be inferred or restored from the original dataset. Previously DPSGD could only be done, economically, on small subsets such as data identified as particularly sensitive.
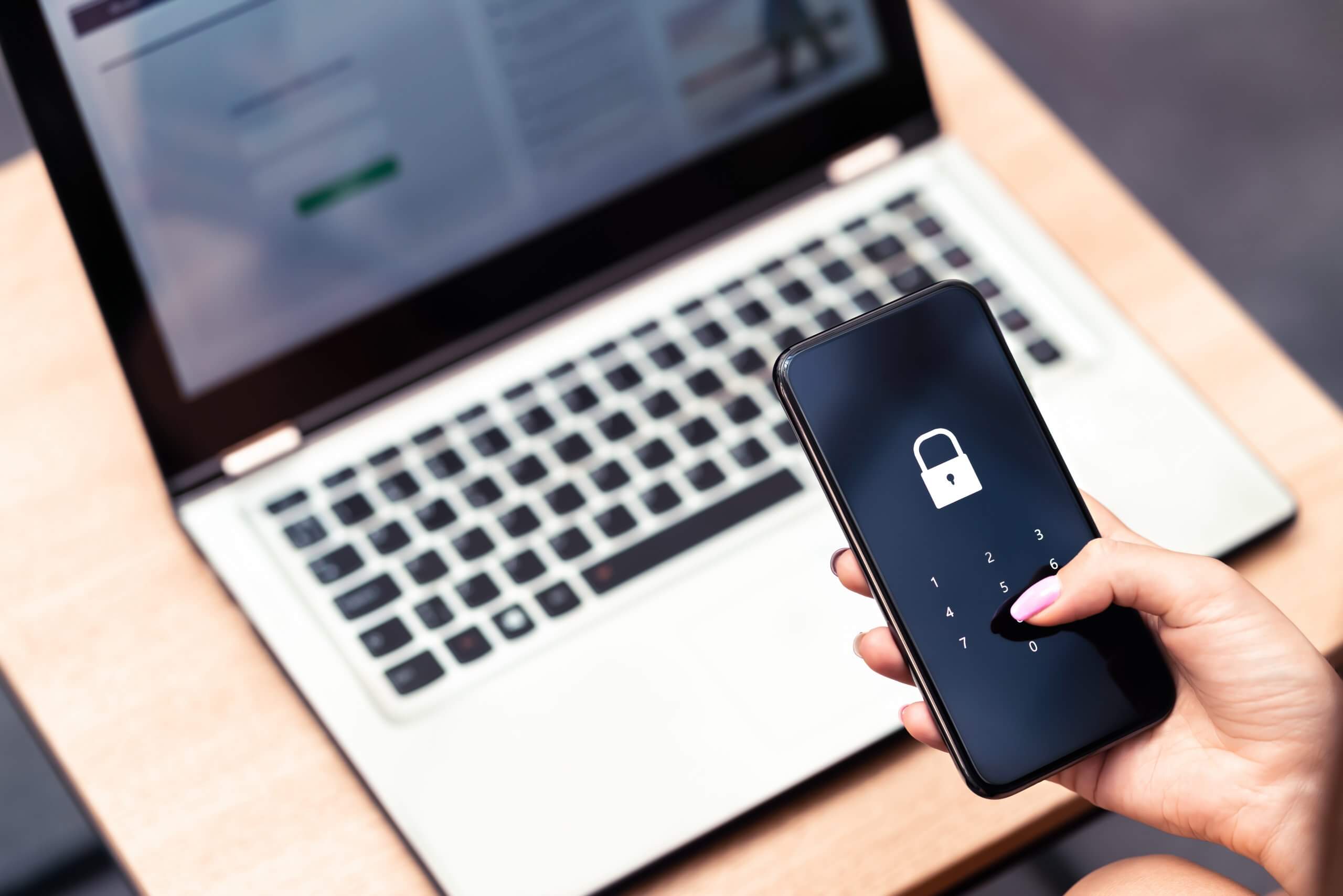
Source: Shutterstock
With Graphcore IPUs, the Stanford researchers could now do what used to take days on GPUs in hours, or 8-11 times faster. This means DPSGD could finally be cost-effective enough to become a viable real-world solution. The secret behind this performance boost is IPU’s MIMD (multiple instructions, multiple data) architecture which enables greater processing efficiency.
The computational overhead from the additional operations required for DPSGD was only an impressive 10% instead of an expected 50% to 90%. Furthermore, the DPSGD-trained AI achieved a 71% accuracy during analysis, 5% below the non-private baseline, which was better than expected given the added noise. The research points to many interesting future possibilities in enhancing data protection undertaken at scale – potentially allowing large data sets to be anonymised as a matter of course.
Balancing accuracy and privacy
Balancing accuracy and privacy are crucial for effective data protection. The sweet spot where privacy is assured while valuable insights can be extracted from the data has yet to be established, but AI engineers continue to make algorithmic progress. Now is the time to invest in technology that can leverage new privacy protection advances such as DPSGD before they become a core part of any business’s operations.
Graphcore IPU is designed for AI
The Graphcore IPU is designed for AI from the ground up. It is the world’s most complex processor, but it is easy to deploy as it can be addressed via industry-standard programming languages, and ships with the company’s own Poplar software.
The British semiconductor company has released the second generation of its systems for AI and machine learning, based on the Colossus™ MK2 GC200 IPU chip, which records an 8x boost in real-world performance compared to the Colossus™ MK1 IPU – the world’s first Intelligence Processing Unit.
Aside from enhancing computing time-to-results and scalability, it also delivers better performance-per-dollar than its competitors.
This is good news for consumers and businesses alike. The technology to implement differential privacy and other ways to minimise privacy violations is becoming more available to the mass market.
Click here for more information on state-of-the-art performance for natural language processing, computer vision, data anonymity and accelerated machine learning.